Efficient Learning Of Healthcare Data From Iot Devices By Edge Convolution Neural Networks
APPLIED SCIENCES-BASEL(2020)
摘要
Wireless and mobile health applications promote the development of smart healthcare. Effective diagnosis and feedbacks of remote health data pose significant challenges due to streaming data, high noise, network latency and user privacy. Therefore, we explore efficient edge and cloud design to maintain electrocardiogram classification performance while reducing the communication cost. These contributions include: (1) We introduce a hybrid smart medical architecture named edge convolutional neural networks (EdgeCNN) that balances the capability of edge and cloud computing to address the issue for agile learning of healthcare data from IoT devices. (2) We present an effective deep learning model for electrocardiogram (ECG) inference, which can be deployed to run on edge smart devices for low-latency diagnosis. (3) We design a data enhancement method for ECG based on deep convolutional generative adversarial network to expand ECG data volume. (4) We carried out experiments on two representative datasets to evaluate the effectiveness of the deep learning model of ECG classification based on EdgeCNN. EdgeCNN shows superior to traditional cloud medical systems in terms of network Input/Output (I/O) pressure, architecture cost and system high availability. The deep learning model not only ensures high diagnostic accuracy, but also has advantages in aspect of inference time, storage, running memory and power consumption.
更多查看译文
关键词
deep convolutional generative adversarial network, deep learning, edge computing, electrocardiograms
AI 理解论文
溯源树
样例
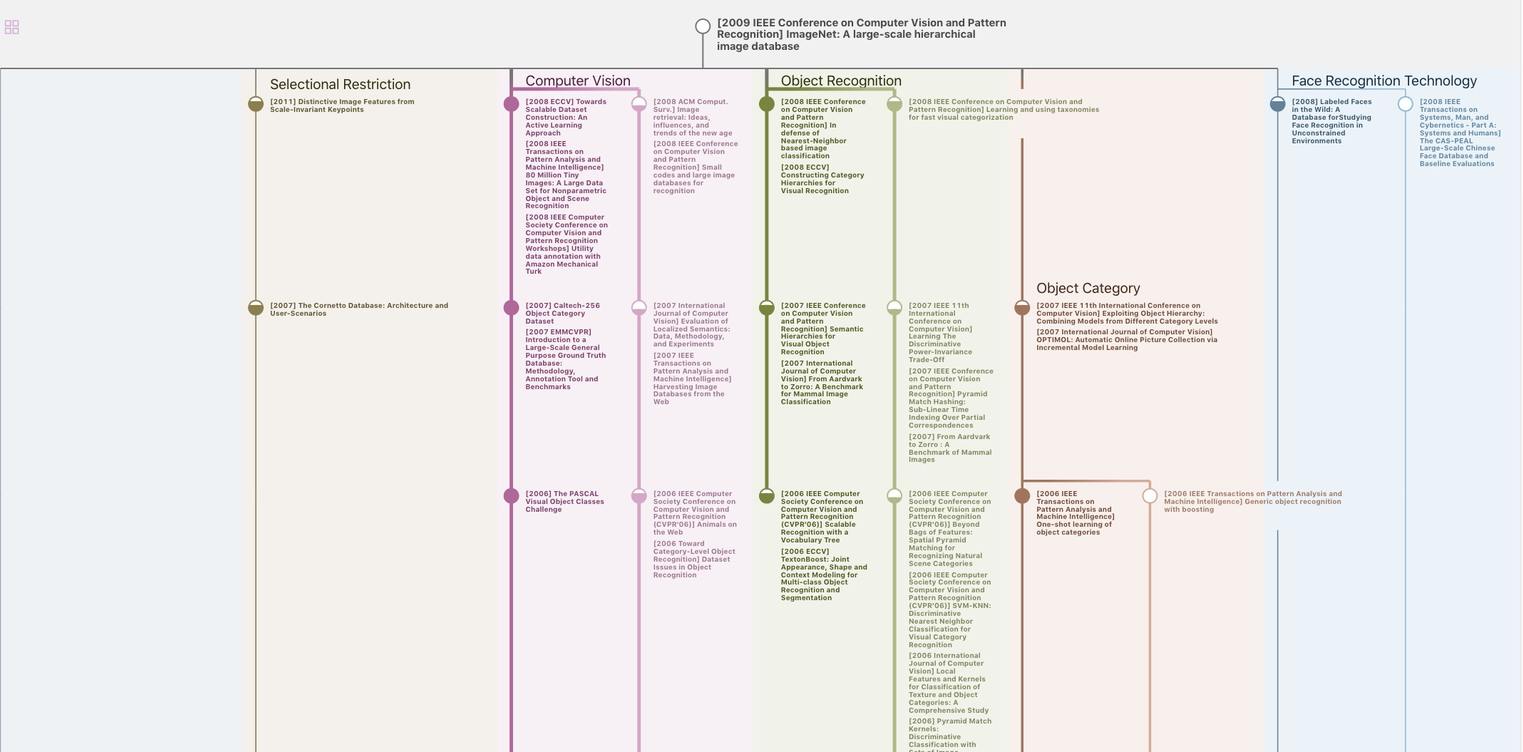
生成溯源树,研究论文发展脉络
Chat Paper
正在生成论文摘要