Radar-Based Bayesian Estimation Of Ice Crystal Growth Parameters Within A Microphysical Model
JOURNAL OF THE ATMOSPHERIC SCIENCES(2021)
摘要
The potential for polarimetric Doppler radar measurements to improve predictions of ice microphysical processes within an idealized model-observational framework is examined. In an effort to more rigorously constrain ice growth processes (e.g., vapor deposition) with observations of natural clouds, a novel framework is developed to compare simulated and observed radar measurements, coupling a bulk adaptive-habit model of vapor growth to a polarimetric radar forward model. Bayesian inference on key microphysical model parameters is then used, via a Markov chain Monte Carlo sampler, to estimate the probability distribution of the model parameters. The statistical formalism of this method allows for robust estimates of the optimal parameter values, along with (non-Gaussian) estimates of their uncertainty. To demonstrate this framework, observations from Department of Energy radars in the Arctic during a case of pristine ice precipitation are used to constrain vapor deposition parameters in the adaptive habit model. The resulting parameter probability distributions provide physically plausible changes in ice particle density and aspect ratio during growth. A lack of direct constraint on the number concentration produces a range of possible mean particle sizes, with the mean size inversely correlated to number concentration. Consistency is found between the estimated inherent growth ratio and independent laboratory measurements, increasing confidence in the parameter PDFs and demonstrating the effectiveness of the radar measurements in constraining the parameters. The combined Doppler and polarimetric observations produce the highest-confidence estimates of the parameter PDFs, with the Doppler measurements providing a stronger constraint for this case.
更多查看译文
关键词
Cloud microphysics, Ice crystals, Radars, Radar observations, Bayesian methods, Cloud parameterizations
AI 理解论文
溯源树
样例
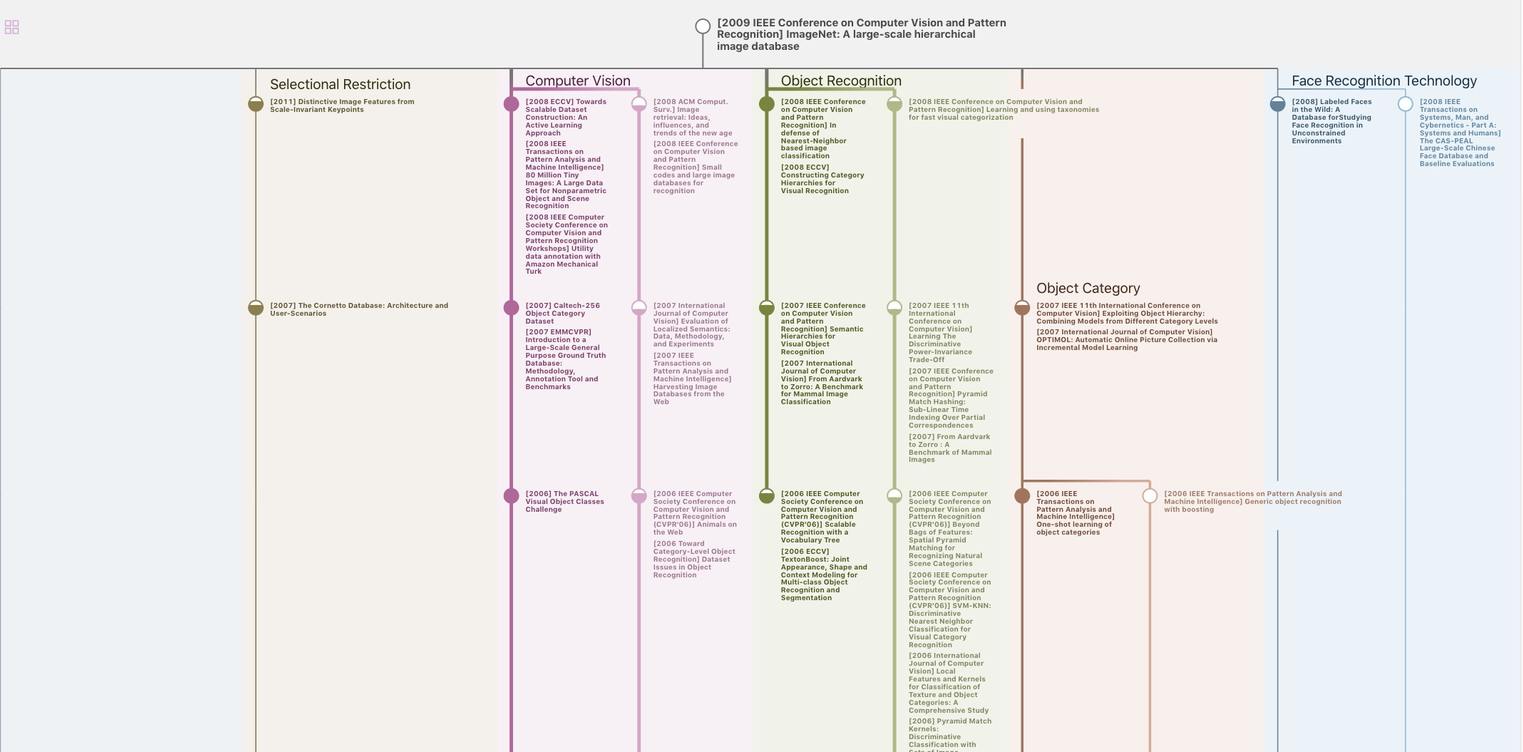
生成溯源树,研究论文发展脉络
Chat Paper
正在生成论文摘要