MLIFeat: Multi-level Information Fusion Based Deep Local Features.
ACCV (3)(2020)
摘要
Accurate image keypoints detection and description are of central importance in a wide range of applications. Although there are various studies proposed to address these challenging tasks, they are far from optimal. In this paper, we devise a model named MLIFeat with two novel light-weight modules for multi-level information fusion based deep local features learning, to cope with both the image keypoints detection and description. On the one hand, the image keypoints are robustly detected by our Feature Shuffle Module (FSM), which can efficiently utilize the multi-level convolutional feature maps with marginal computing cost. On the other hand, the corresponding feature descriptors are generated by our well-designed Feature Blend Module (FBM), which can collect and extract the most useful information from the multi-level convolutional feature vectors. To study in-depth about our MLIFeat and other state-of-the-art methods, we have conducted thorough experiments, including image matching on HPatches and FM-Bench, and visual localization on Aachen-Day-Night, which verifies the robustness and effectiveness of our proposed model.
更多查看译文
关键词
features,local,deep,information,multi-level
AI 理解论文
溯源树
样例
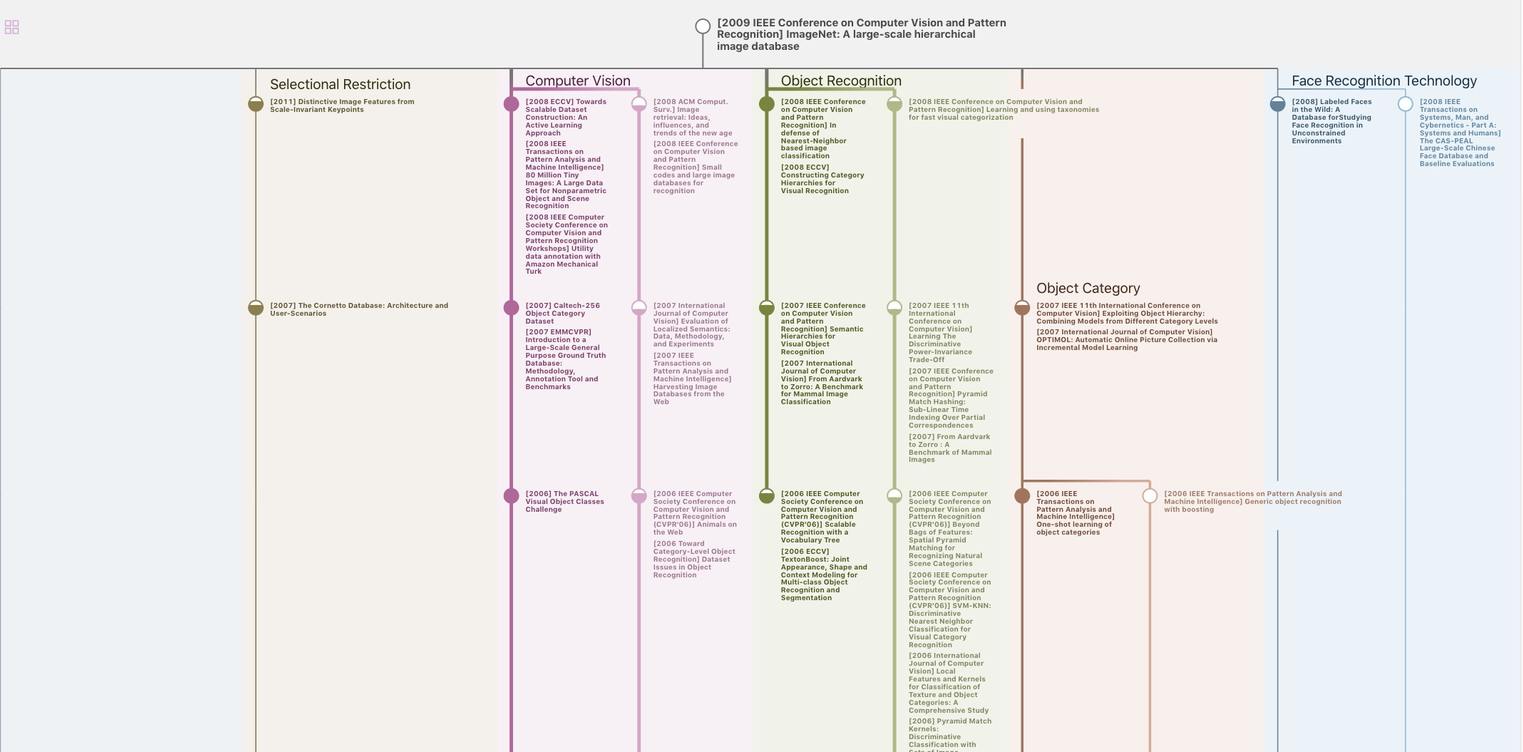
生成溯源树,研究论文发展脉络
Chat Paper
正在生成论文摘要