Malware Classification Using Attention-Based Transductive Learning Network.
SecureComm (2)(2020)
Abstract
Malware has now grown up to be one of the most important threats in the internet security. As the number of malware families has increased rapidly, a malware classification model needs to classify the samples from emerging malware families. In real-world environment, the number of malware samples varies greatly with each family and some malware families only have a few samples. Therefore, it is a challenge task to obtain a malware classification model with strong generalization ability by using only a few labeled malware samples in each family. In this paper, we propose an attention-based transductive learning approach to tackle this problem. To extract features from raw malware binaries, our approach first converts them into gray-scale images. After visualization, an embedding function is used to encode the images into feature maps. Then we build an attention-based Gaussian similarity graph to help transduct the label information from well-labeled instances to unknown instances. With end-to-end training, we validate our attention-based transductive learning network on a malware database of 11,236 samples with 30 different malware families Comparing with state-of-the-art approaches, the experimental results show that our approach achieves a better performance.
MoreTranslated text
Key words
Malware classification, Tranductive learning, Attention mechanism, Deep learning
AI Read Science
Must-Reading Tree
Example
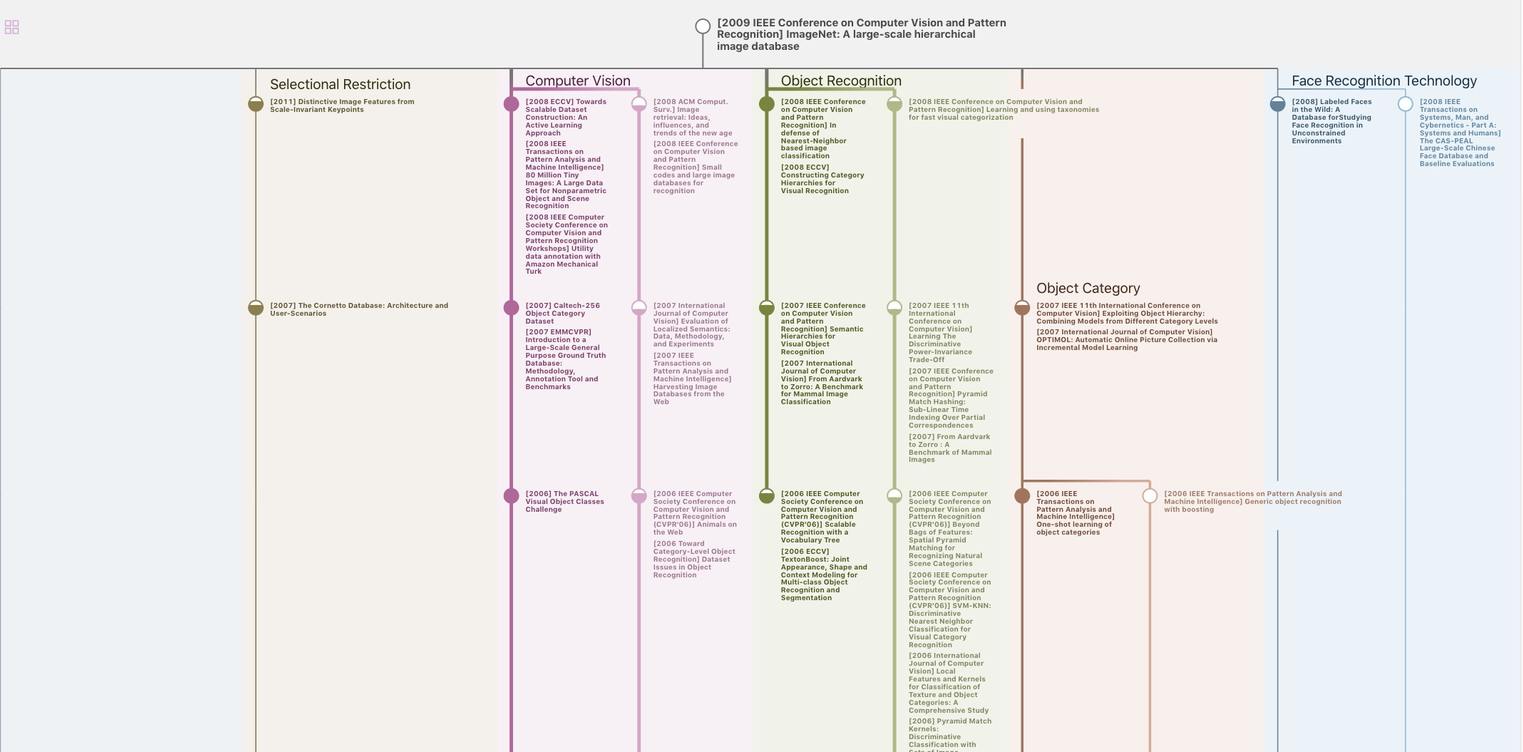
Generate MRT to find the research sequence of this paper
Chat Paper
Summary is being generated by the instructions you defined