Fault diagnosis technique in internal combustion engines using machine learning
REVISTA CUBANA DE INGENIERIA(2020)
摘要
The current industrial scenario points to an increase in industrial competitiveness, in the complexity of machinery and equipment, in the costs of industrial installations, in high demand products, in addition to the concern with aspects of industrial safety and the environment. This trend induces large global industries to increasingly invest in devices, technologies and tools designed to predict failures resulting from non-conformities and breakdowns in machinery, equipment and industrial installations. Given this scenario, the field of action that deals with predictive maintenance, forecast analysis and failure diagnosis has gained a prominent place, in addition to several investments in research and development, mainly with policies aimed at the design of industry 4.0. With the industry 4.0 approach, it is possible to analyze mechanical components in a dynamic regime and respond in real time, that is, without the need to stop the machine, which is directly related to the reduction of costs and production time. That said, the present thesis aims to present a new methodology in the detection and monitoring of tribological failures in internal combustion engines, through machine learning by unsupervised methods and big data, using signal processing techniques, from the collected data engine vibration and sound pressure level, using algorithms based on artificial neural networks (RNA) and cluster analysis, creating an intelligent system capable of detecting fault patterns, based on fault conditions and mechanical load variation in motors internal combustion Otto cycle.
更多查看译文
关键词
tribology,internal combustion engines,machine learning,big data,artificialneural networks,fail diagnosis
AI 理解论文
溯源树
样例
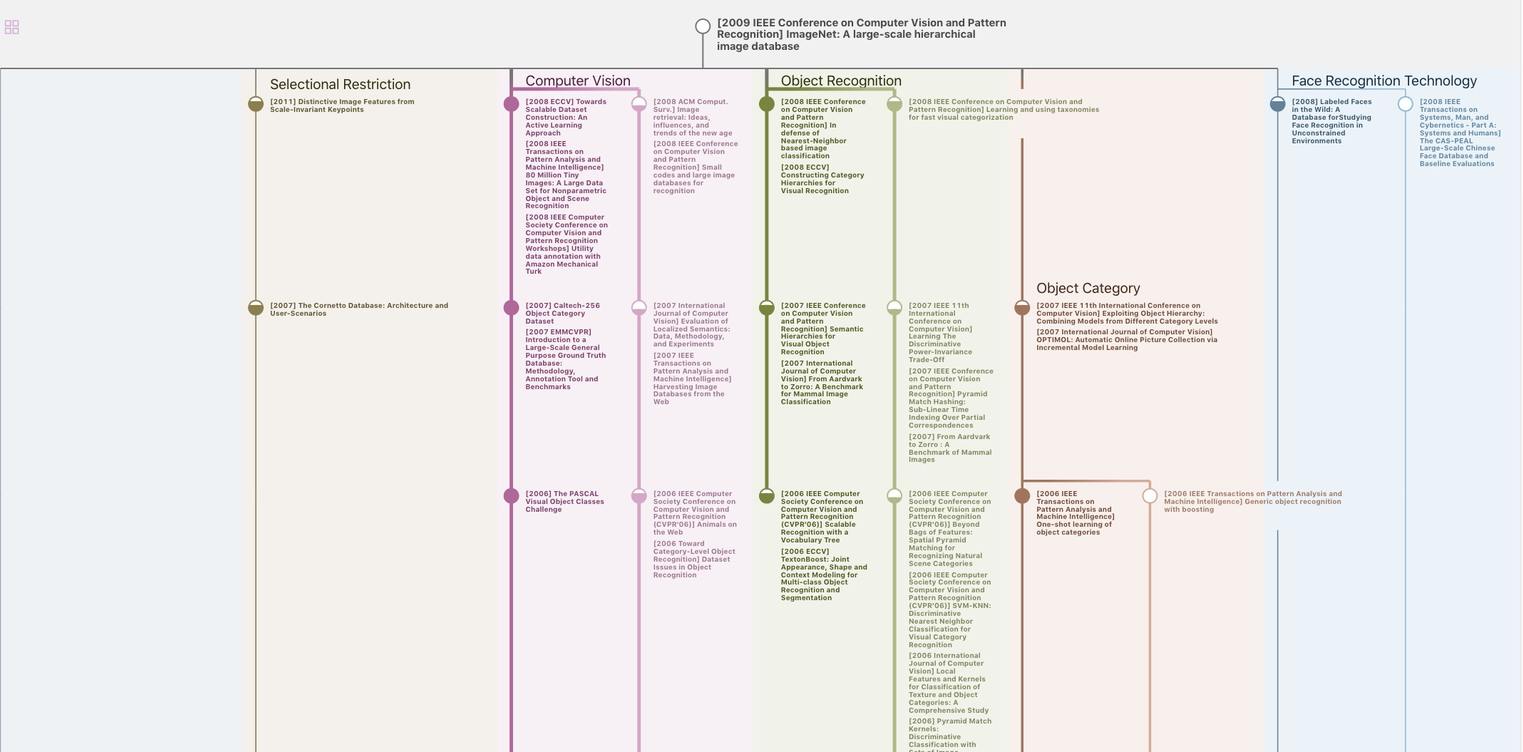
生成溯源树,研究论文发展脉络
Chat Paper
正在生成论文摘要