Extracting Topological Features from Big Data Using Persistent Density Entropy
Journal of Physics Conference Series(2019)
摘要
Topological data analysis is a method of extracting shape information of big data by means of algebraic topology in mathematics. Persistent homology is a very important method in topological data analysis. It constructs multi-scale simplicial complexes (also called filtration) to approximate the underlying space of the data set. By studying these simplicial complexes, the topological features of each dimension of big data are summarized. However, it does not give us the uncertainty of each simplicial complex to approximate the underlying space of the data set. This paper defines an entropy called persistent density entropy, which gives the uncertainty of each simplicial complex approximating the underlying space. The examples demonstrate that it is able to find the best simplicial complex that approximates the underlying space and can be used to detect outliers to a certain extent.
更多查看译文
AI 理解论文
溯源树
样例
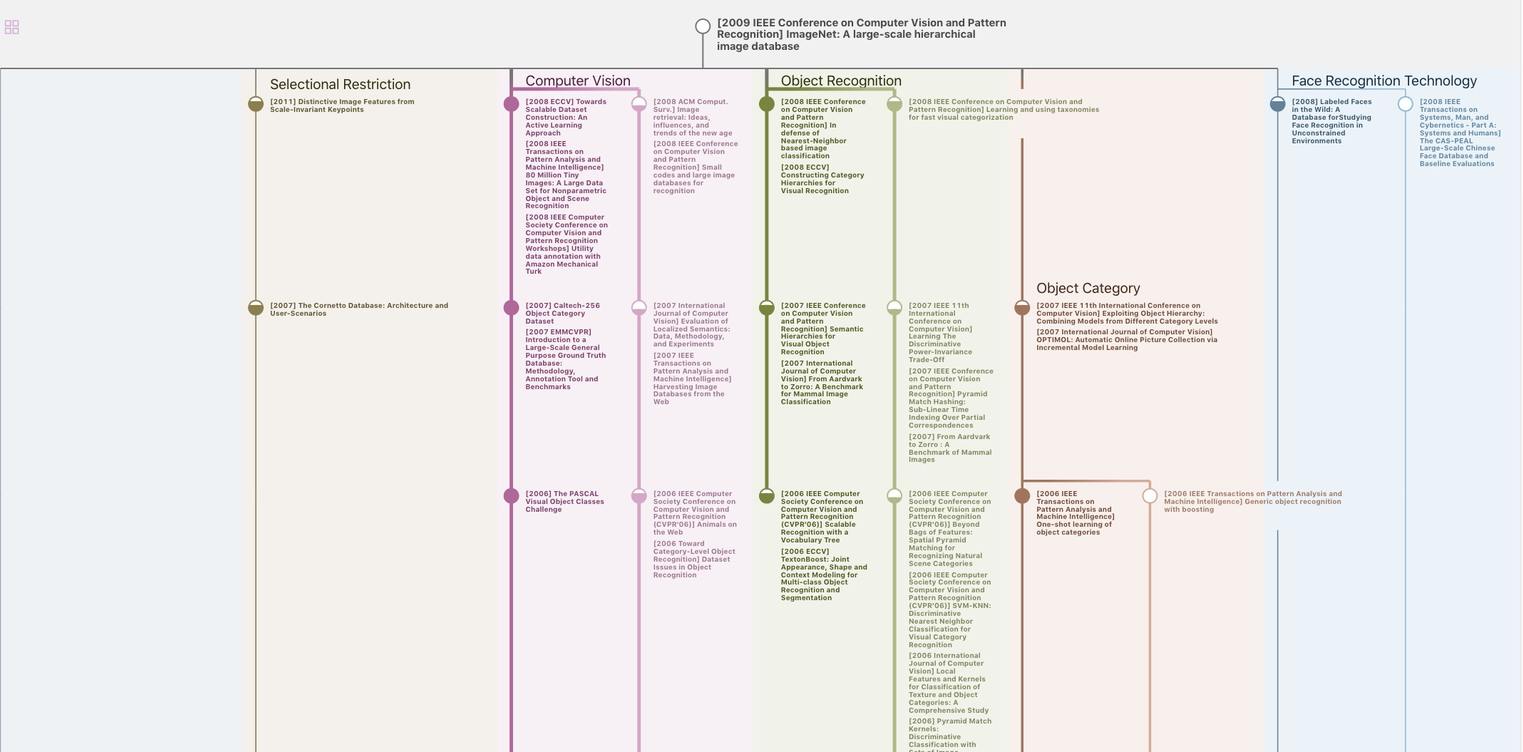
生成溯源树,研究论文发展脉络
Chat Paper
正在生成论文摘要