Impact of surface data assimilation using an ensemble Kalman filter technique on the improvement of weather prediction
Journal of Physics Conference Series(2019)
摘要
Initial condition data is known to be an important factor, which contributes to the accuracy of a weather prediction. One of an attempt to improve the initial condition is by applying data assimilation method. Ensemble Kalman Filter (EnKF) is a sophisticated data assimilation method that has shown great promise for atmospheric data assimilation in a way that the method uses flow-dependent error statistics estimated from relatively short period of prediction. Data assimilation of surface observations using an EnKF technique is evaluated for the potential improvement of a regional nonhydrostatic model. We use Weather Research and Forecasting - Advanced Research WRF (WRF-ARW) model to implement the EnKF data assimilation system. The EnKF system combined with the WRF-ARW model on a 1-km horizontal grid spacing over Karimunjawa Island is cycled over a field course period from 14-18 August 2015. The analysis ensemble is generated every 6 h by assimilating surface observations from three different locations of automatic weather stations (AWSs). By assimilating the surface observation data, the models produce colder temperature over the sea near Karimunjawa Island compare to those without assimilation process. Thus, the assimilation data give a stronger and more defined sea breeze circulation because the circulation generated by temperature difference between the sea and land.
更多查看译文
AI 理解论文
溯源树
样例
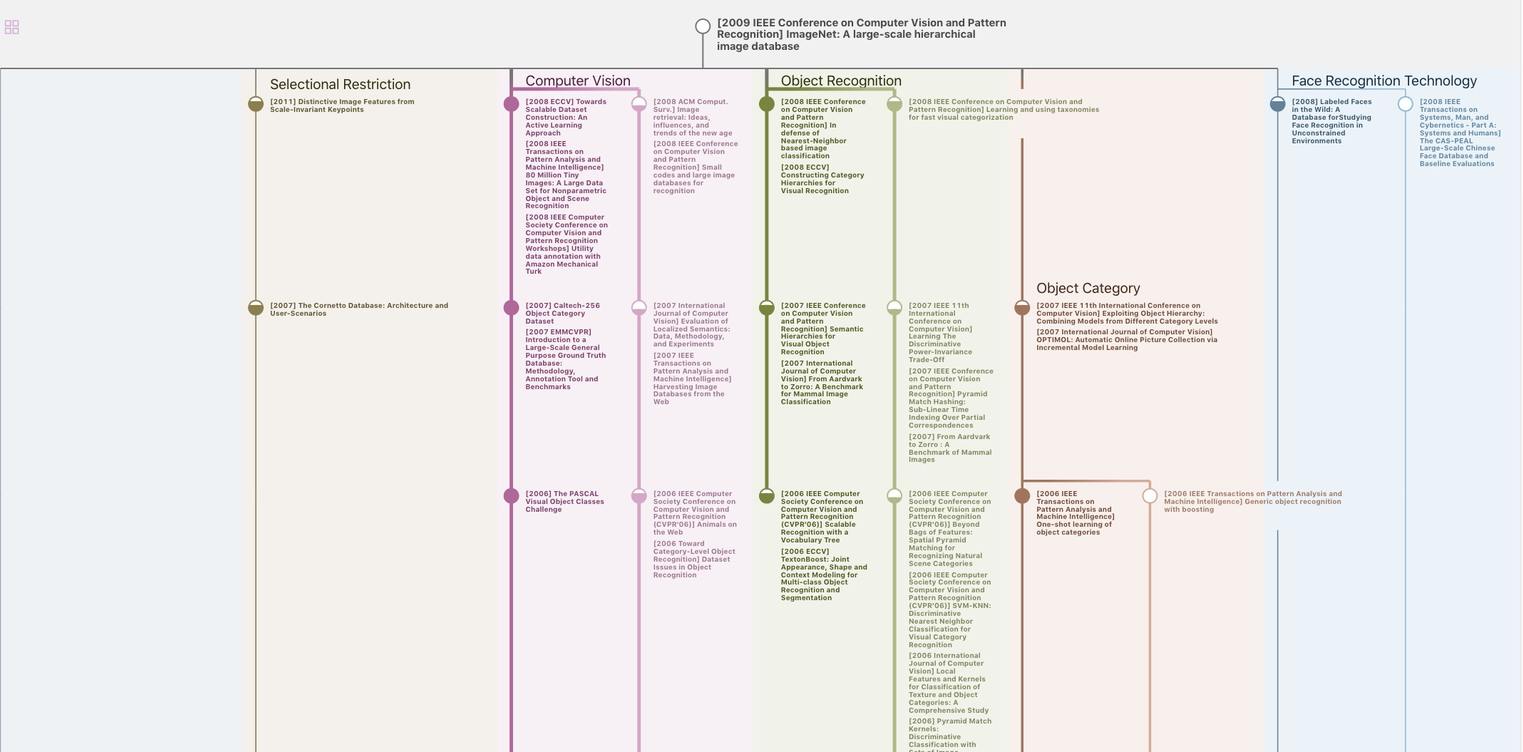
生成溯源树,研究论文发展脉络
Chat Paper
正在生成论文摘要