A Joint Diagonalization Based Efficient Approach To Underdetermined Blind Audio Source Separation Using The Multichannel Wiener Filter
IEEE-ACM TRANSACTIONS ON AUDIO SPEECH AND LANGUAGE PROCESSING(2021)
摘要
Blind source separation (BSS) of audio signals aims to separate original source signals fromtheir mixtures recorded by microphones. The applications include automatic speech recognition in a noisy/multi-speaker environment, hearing aids, and music analysis. Independent component analysis (ICA) can perform BSS efficiently, but it is basically inapplicable to the underdetermined case-the number of sources > the number of microphones. In contrast, a BSS approach using the multichannel Wiener filter (MWF) is applicable even to the underdetermined case, but conventional methods based on this approach-including full-rank spatial covariance analysis (FCA)-are highly inefficient. This is because these methods require massive numbers of matrix inversions to design the MWF. To obtain the best of both worlds, we take a joint diagonalization approach: We restrict spatial covariance matrices of all sources to the class of jointly diagonalizable matrices. This enables the above matrix inversions to be replaced by mere scalar inversions of the diagonal elements of diagonal matrices. Based on this, we present FastFCA and Fast MNMF-efficient methods for underdetermined BSS. In an experiment, Fast FCA was several orders of magnitude faster than FCA without sacrificing separation performance. We also present a unified framework for underdetermined and determined BSS, which highlights theoretical connections between various methods including ours. The efficiency of our BSS methods makes them suitable for large data (e.g., data augmentation for machine learning) or limited computational resources encountered in, e.g., hearing aids, distributed microphone arrays, and online BSS.
更多查看译文
关键词
Blind source separation, joint diagonalization, maximum likelihood estimation, microphone arrays, multichannel Wiener filter
AI 理解论文
溯源树
样例
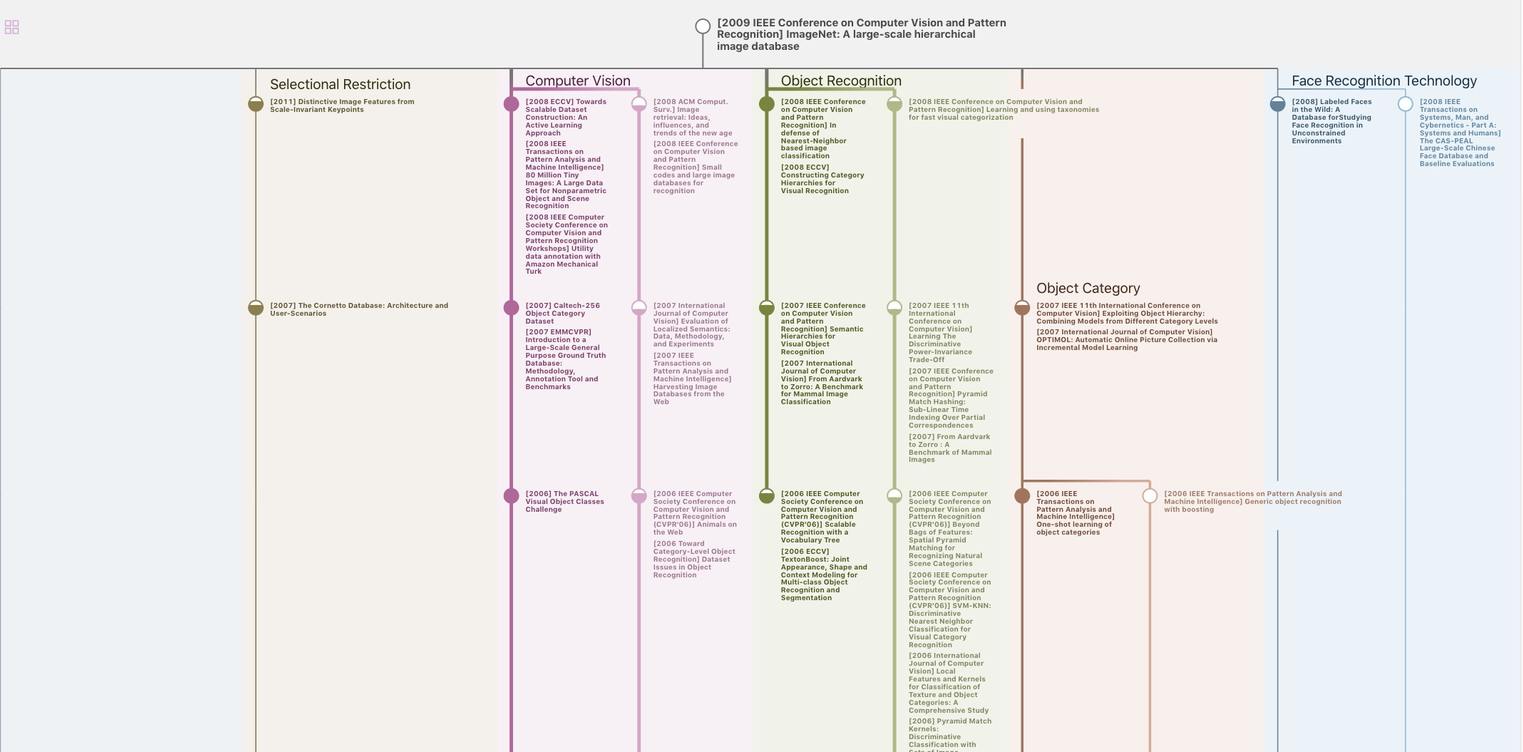
生成溯源树,研究论文发展脉络
Chat Paper
正在生成论文摘要