Automated Experimentation Powers Data Science In Chemistry
ACCOUNTS OF CHEMICAL RESEARCH(2021)
Abstract
Data science has revolutionized chemical research and continues to break down barriers with new interdisciplinary studies. The introduction of computational models and machine learning (ML) algorithms in combination with automation and traditional experimental techniques has enabled scientific advancement across nearly every discipline of chemistry, from materials discovery, to process optimization, to synthesis planning. However, predictive tools powered by data science are only as good as their data sets and, currently, many of the data sets used to train models suffer from several limitations, including being sparse, limited in scope and requiring human curation. Likewise, computational data faces limitations in terms of accurate modeling of nonideal systems and can suffer from low translation fidelity from simulation to real conditions. The lack of diverse data and the need to be able to test it experimentally reduces both the accuracy and scope of the predictive models derived from data science. This Account contextualizes the need for more complex and diverse experimental data and highlights how the seamless integration of robotics, machine learning, and data-rich monitoring techniques can be used to access it with minimal human labor.We propose three broad categories of data in chemistry: data on fundamental properties, data on reaction outcomes, and data on reaction mechanics. We highlight flexible, automated platforms that can be deployed to acquire and leverage these data. The first platform combines solid- and liquid-dosing modules with computer vision to automate solubility screening, thereby gathering fundamental data that are necessary for almost every experimental design. Using computer vision offers the additional benefit of creating a visual record, which can be referenced and used to further interrogate and gain insight on the data collected. The second platform iteratively tests reaction variables proposed by a ML algorithm in a closed-loop fashion. Experimental data related to reaction outcomes are fed back into the algorithm to drive the discovery and optimization of new materials and chemical processes. The third platform uses automated process analytical technology to gather real-time data related to reaction kinetics. This system allows the researcher to directly interrogate the reaction mechanisms in granular detail to determine exactly how and why a reaction proceeds, thereby enabling reaction optimization and deployment.
MoreTranslated text
AI Read Science
Must-Reading Tree
Example
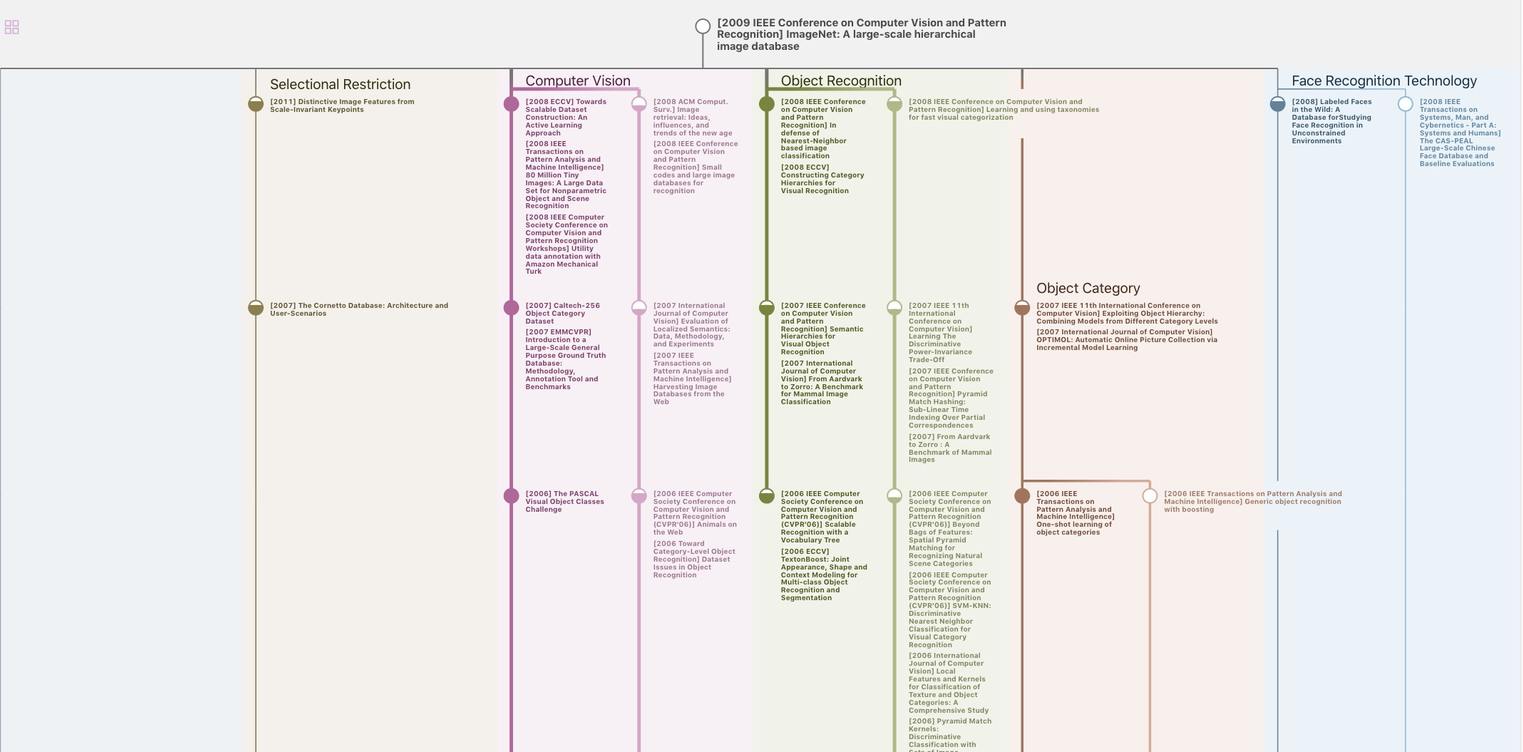
Generate MRT to find the research sequence of this paper
Chat Paper
Summary is being generated by the instructions you defined