LIRNet - Local Integral Regression Network for Both Strongly and Weakly Supervised Nuclei Detection.
BIBM(2020)
摘要
Nuclei detection is one of the most important issues in the field 0fhistopathology i mage analysis. Recent studies exploit convolutional neural networks to either locate the centroids of nuclei (counting-based approaches) or isolate them with sophisticated boundaries (segmentation-based algorithms). However, the training of these two methods demands considerable and laborious pixel-wise annotations labeled by pathology experts. To reduce the annotation cost, we propose a novel local integral regression network (LIRNet) that allows both strongly and weakly supervised learning (SSL/WSL) frameworks on nuclei detection. In addition, the LIRNet is able to predict a refined density map of n uclei, in which locating e ach n ucleus is barely affected by the post-processing algorithms. Quantitative experimental results demonstrate that the SSL version of the LIRNet achieves state-of-the-art performance compared with counterparts. Besides, the WSL version possesses a competitive detection performance and an effortless data annotation that dramatically reduces annotation cost by 74.3%.
更多查看译文
关键词
Nuclei detection, convolutional neural network, weakly supervised learning
AI 理解论文
溯源树
样例
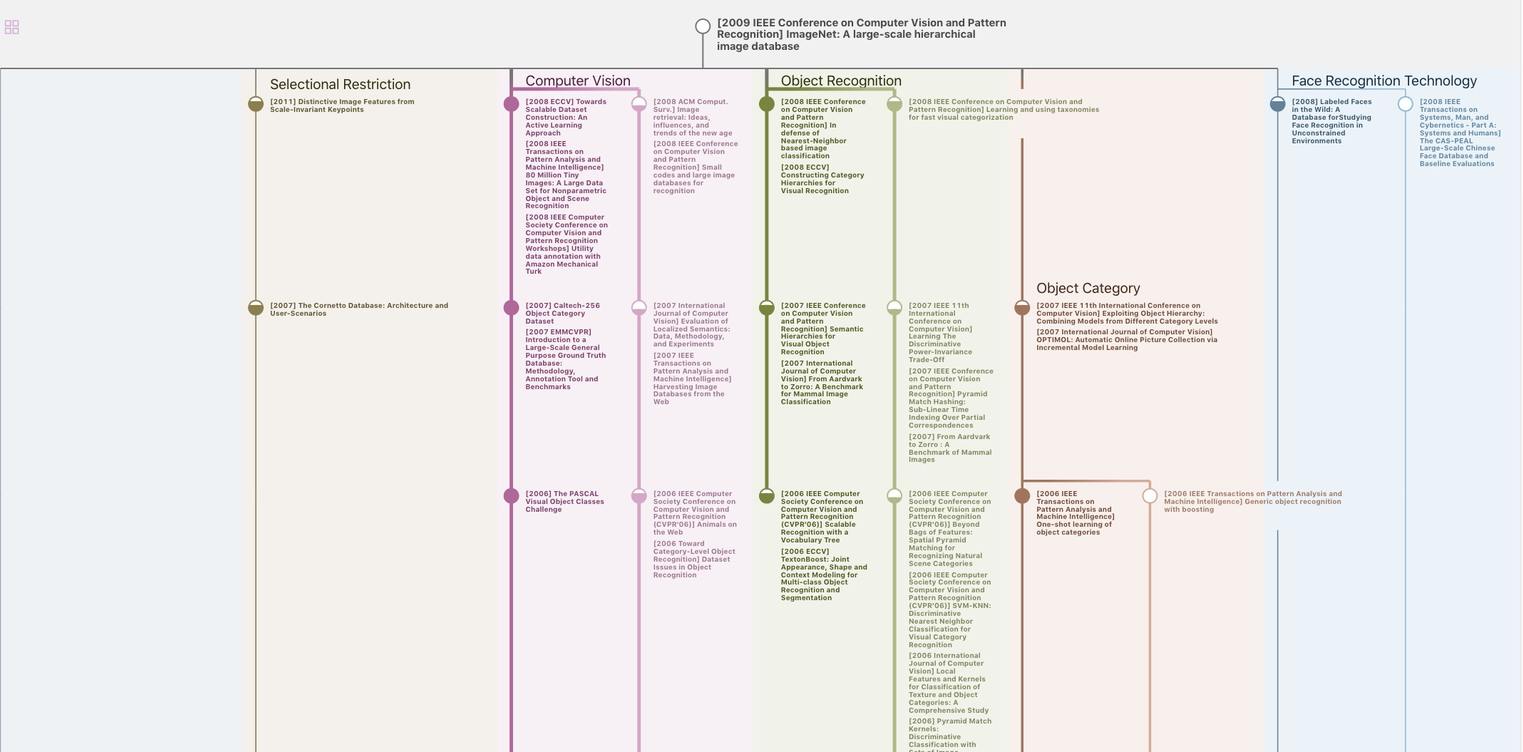
生成溯源树,研究论文发展脉络
Chat Paper
正在生成论文摘要