A Sequential Graph Convolutional Network with Frequency-domain Complex Network of EEG Signals for Epilepsy Detection.
BIBM(2020)
摘要
Automatic epilepsy seizure detection based on electroencephalography (EEG) signals has been a hot topic in the bioinformatics community. Recently, graph representations named complex networks have been increasingly utilized to characterize EEG signals. However, existing time-domain complex networks often suffer from undesired intra-class variance due to phase shift. Addressing this problem, we propose to obtain complex network representations in frequency domain where perfect data alignment can be achieved. The transformation to frequency domain highlights the urgency to retain sequential information in the signals. To this end, we propose to further extract features from the complex network representation using a novel deep model called Sequential Graph Convolutional Network (SGCN). Specifically, we incorporate state-of-the-art graph neural network (GNN) architecture with a novel sequential convolution operation which is key to preserving sequential information. Extensive experiments demonstrate the effectiveness and interpretability of our method. Our source code is available at https://github.com/JL-Wang-source-code/SGCN-for-epilepsy-detection.
更多查看译文
关键词
Epilepsy detection,EEG,Graph representation,Complex network,Graph neural network
AI 理解论文
溯源树
样例
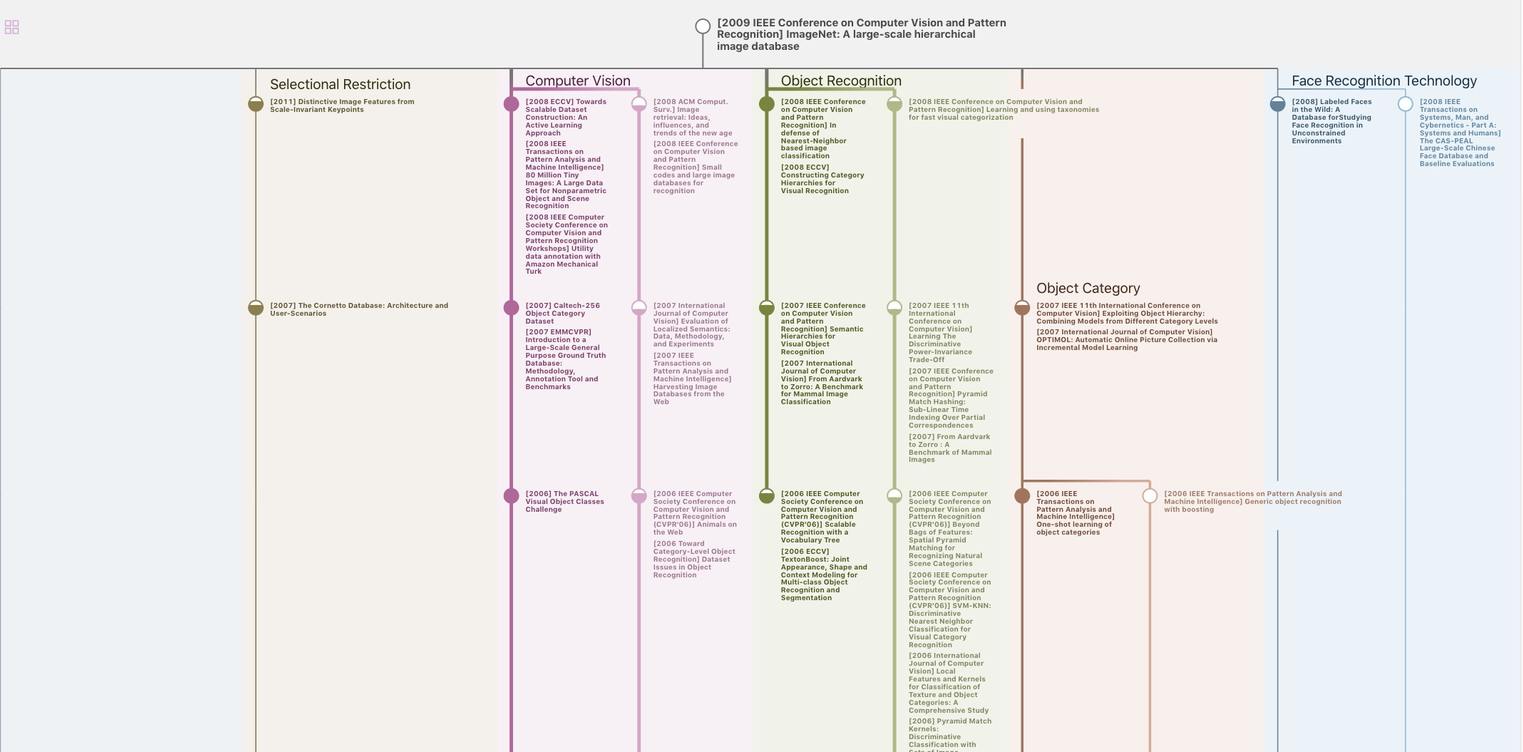
生成溯源树,研究论文发展脉络
Chat Paper
正在生成论文摘要