Exploring Optimal Adaptive Activation Functions for Various Tasks.
BIBM(2020)
摘要
An activation function is a key component of artificial neural networks (ANNs). It has a great impact on the performance and convergence of neural networks. In this work, a self-adapting methodology is proposed to explore the optimal adaptive activation functions for various tasks based on S-shaped or ReLu-shaped activation functions, which are regulated only by introducing several parameters. To verify the effectiveness of the proposed methodology, a series of comparison experiments are performed with MLP, CNN and RNN network structure on the benchmark datasets of image, text and audio. The experimental results are encouraging, and show that the proposed methodology can locate the optimal activation functions for various tasks. Nevertheless, the obtained functions are competitive and the improvements on network performance are significant compared with other popular activation functions, such as ELU, PReLU, ReLU, and Sigmoid.
更多查看译文
关键词
Activation functions,Adaptable parameters,Various tasks
AI 理解论文
溯源树
样例
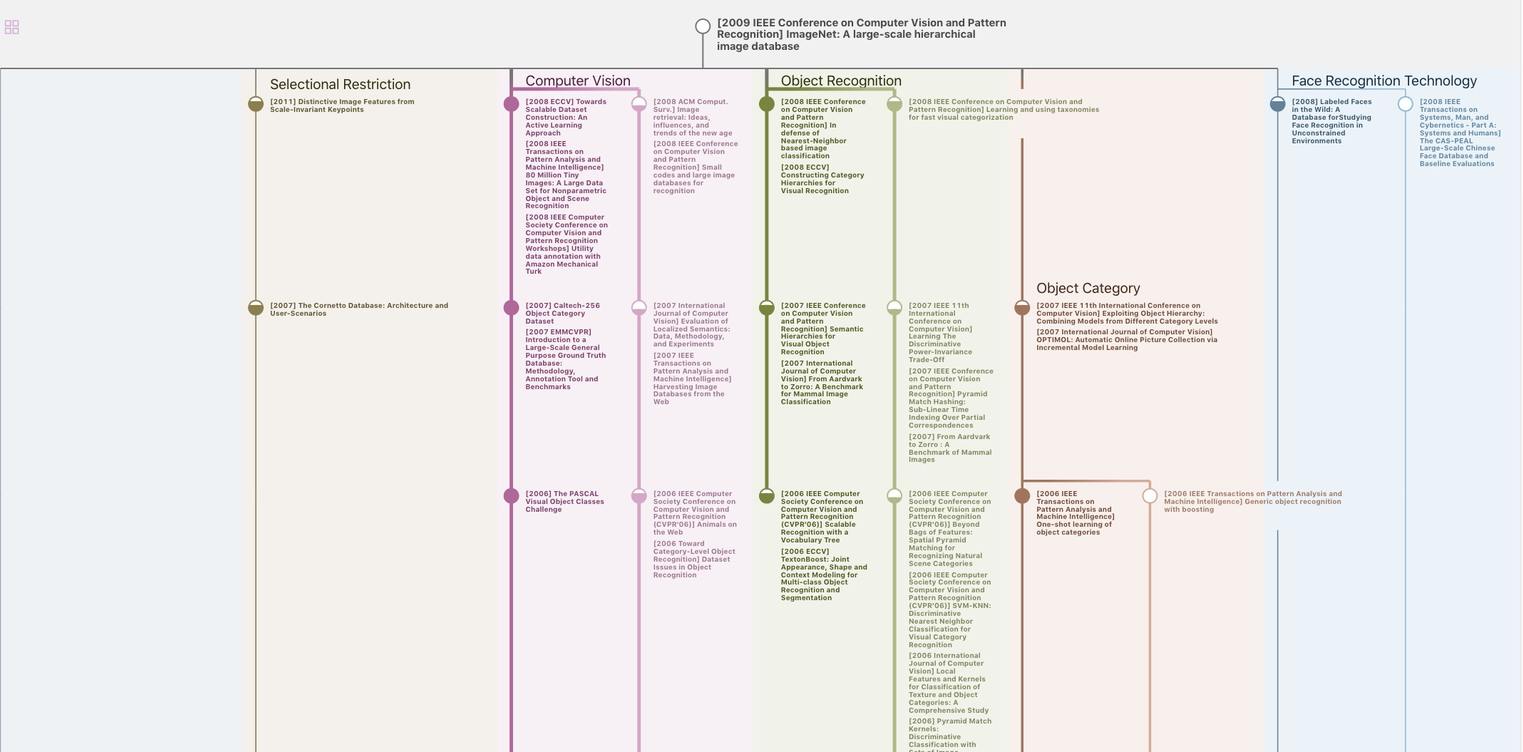
生成溯源树,研究论文发展脉络
Chat Paper
正在生成论文摘要