Nonconvex optimization on data manifold by accelerated curvature transport
2020 59th IEEE Conference on Decision and Control (CDC)(2020)
摘要
In this paper, we explore a new approach to optimization of cost or utility functions defined over a surface, a manifold, or its simplicial decomposition. In the era of Big Data, heterogeneous signal samples sometimes embed with less distortion in a lower dimensional space if the embedding space is a manifold rather than the traditional Euclidean space. If a utility function is defined over the data and if there is a need to identify significant events defined by extreme values of the utility function, we are faced with the problem of identifying the extreme minima/maxima points of the cost/utility function defined over the manifold or its triangulation. The fundamental idea developed here is to observe that at the extreme points the graph of the utility function has extreme curvature. Accordingly, the celebrated Ricci/Yamabe flow for uniformization of the curvature of the graph will show significant "curvature transport" in the vicinity of the extreme values, hence allowing their rapid identification, obviating the classical sorting. The novel theoretical contribution is to accelerate the process by compounding the Laplace operator.
更多查看译文
关键词
Euclidean space,extreme points,extreme curvature,nonconvex optimization,data manifold,accelerated curvature transport,Big Data,heterogeneous signal samples,embedding space
AI 理解论文
溯源树
样例
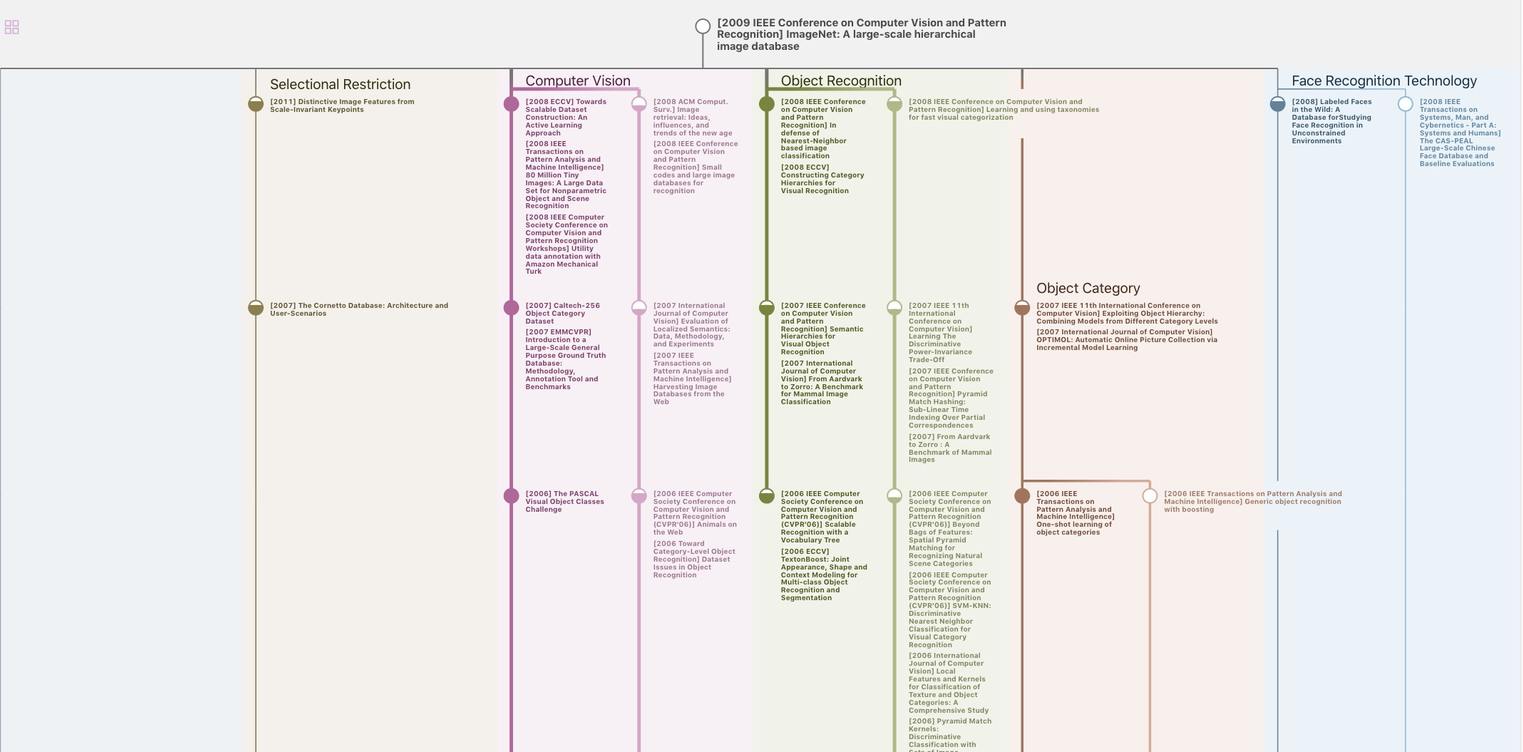
生成溯源树,研究论文发展脉络
Chat Paper
正在生成论文摘要