Multi-level Topological Analysis Framework for Multifocal Diseases
2020 16th International Conference on Control, Automation, Robotics and Vision (ICARCV)(2020)
摘要
Feature engineering and deep learning have been widely used to characterize imaging features in medical applications. However, the importance of geometric structure and spatial relationship of multiple lesions for multifocal diseases are often neglected by these methods. In this paper, we propose a Multi-level Topological Analysis (MTA) framework based on persistent homology, by capturing global-level topological invariants underlying geometric structure and local-level spatial adjacency relationship among lesions and local structure. In particular, a novel Filtration-based Community Discovery algorithm is designed to efficiently partition lesions to local clusters. Experiments demonstrate that our MTA framework outperforms five state-of-the-art persistent homology methods and achieved AUC 824±0.132 on a task of differentiating two multifocal diseases, Multiple Sclerosis and Neuromyelitis Optica.
更多查看译文
关键词
state-of-the-art persistent homology methods,multifocal diseases,deep learning,imaging features,medical applications,geometric structure,spatial relationship,global-level topological invariants,local-level spatial adjacency relationship,local structure,local clusters,MTA framework,novel filtration-based community discovery algorithm,multilevel topological analysis framework
AI 理解论文
溯源树
样例
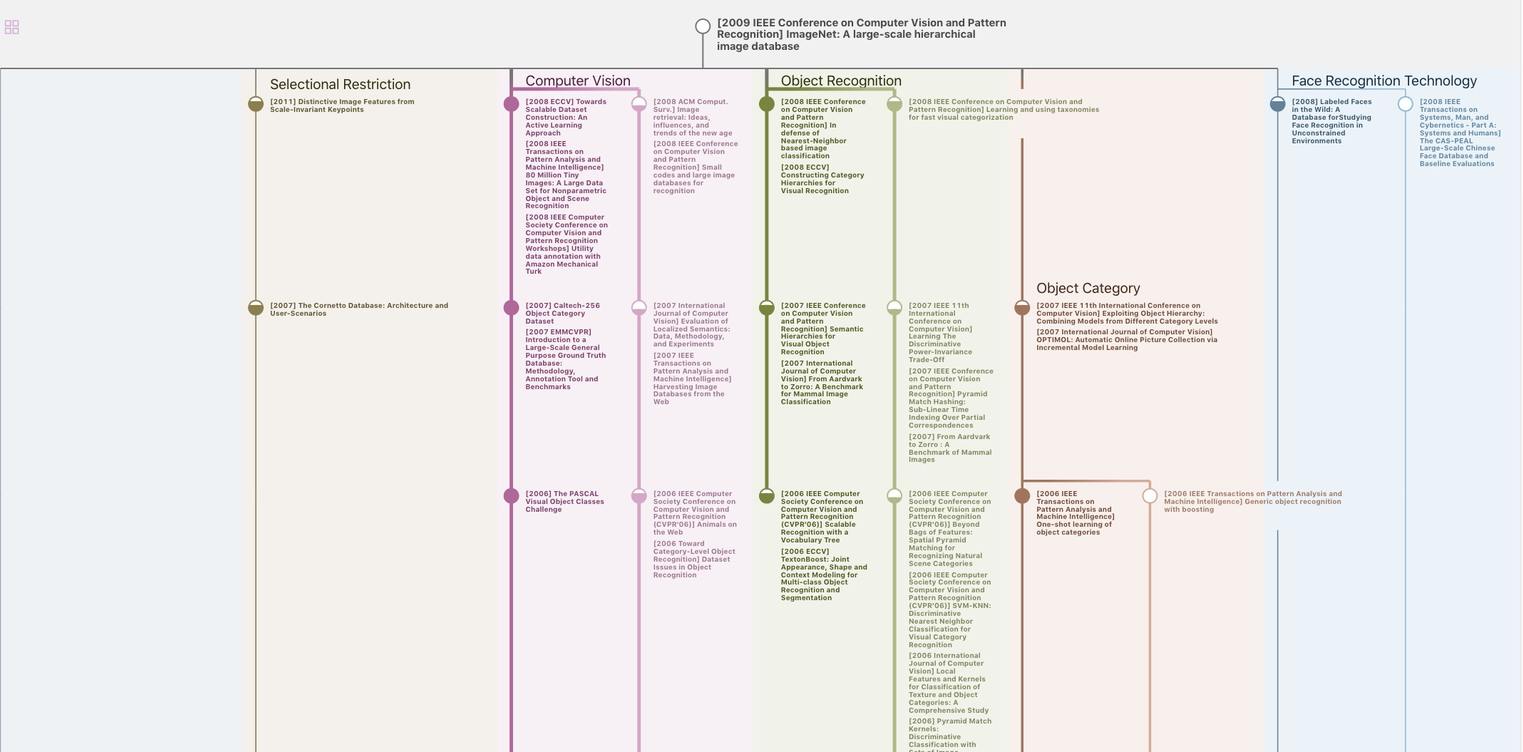
生成溯源树,研究论文发展脉络
Chat Paper
正在生成论文摘要