Automatic Privacy and Utility Preservation for Mobility Data: A Nonlinear Model-Based Approach
Periodicals(2021)
摘要
AbstractThe widespread use of mobile devices and location-based services has generated a large number of mobility databases. While processing these data is highly valuable, privacy issues can occur if personal information is revealed. The prior art has investigated ways to protect mobility data by providing a wide range of Location Privacy Protection Mechanisms (LPPMs). However, the privacy level of the protected data significantly varies depending on the protection mechanism used, its configuration and on the characteristics of the mobility data. Meanwhile, the protected data still needs to enable some useful processing. To tackle these issues, we present PULP, a framework that finds the suitable protection mechanism and automatically configures it for each user in order to achieve user-defined objectives in terms of both privacy and utility. PULP uses nonlinear models to capture the impact of each LPPM on data privacy and utility levels. Evaluation of our framework is carried out with two protection mechanisms from the literature and four real-world mobility datasets. Results show the efficiency of PULP, its robustness and adaptability. Comparisons between LPPMs’ configurators and the state of the art further illustrate that PULP better realizes users’ objectives, and its computation time is in orders of magnitude faster.
更多查看译文
关键词
Privacy, Data privacy, Data models, Measurement, Computational modeling, Adaptation models, Usability, Security and privacy protection, modeling and prediction, security, integrity, and protection, location-dependent and sensitive, configuration control
AI 理解论文
溯源树
样例
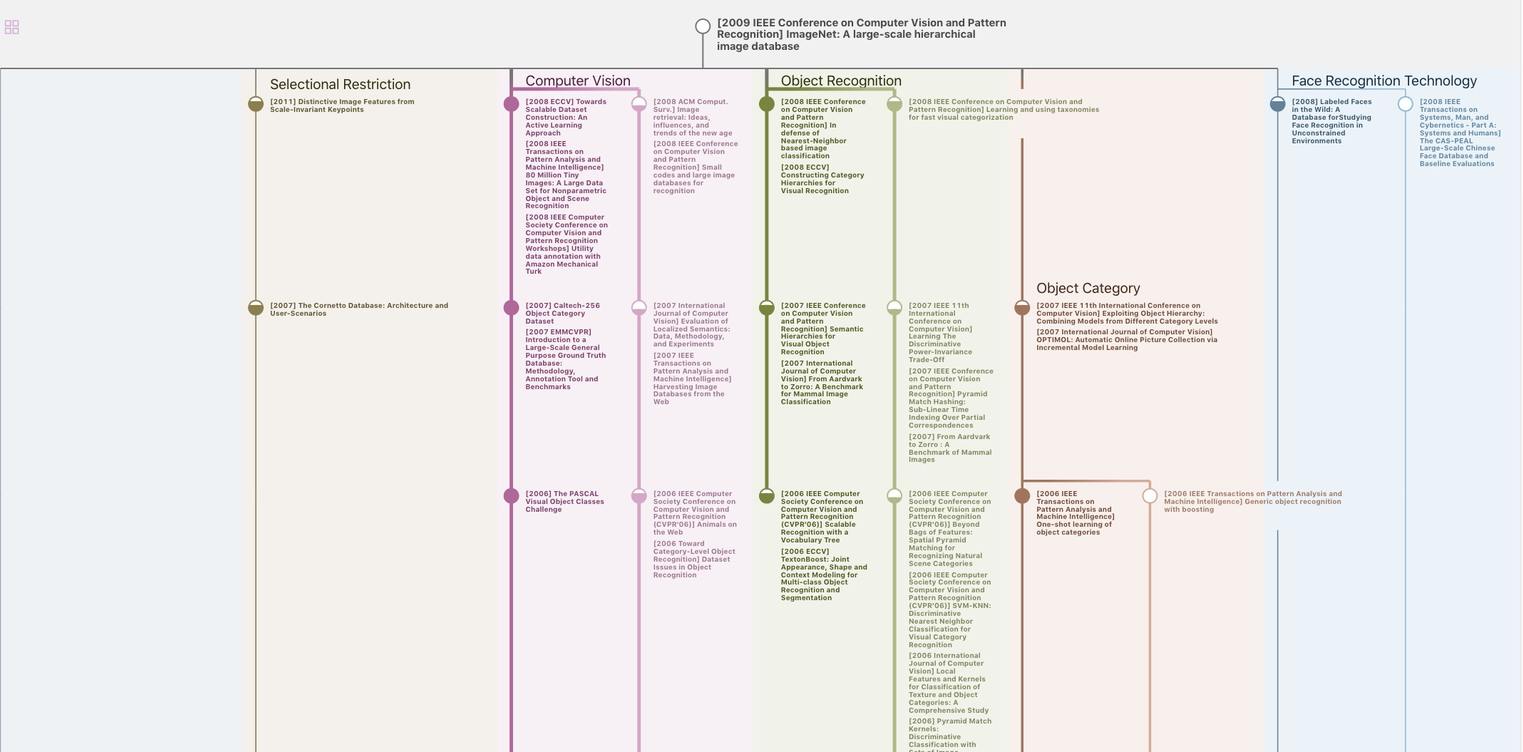
生成溯源树,研究论文发展脉络
Chat Paper
正在生成论文摘要