Drop-Bottleneck: Learning Discrete Compressed Representation for Noise-Robust Exploration
ICLR(2021)
摘要
We propose a novel information bottleneck (IB) method named Drop-Bottleneck, which discretely drops input features that are irrelevant to the target variable. Drop-Bottleneck not only enjoys a simple and tractable compression objective but also additionally provides a deterministic compressed representation of the input variable, which is useful for inference tasks that require consistent representation. Moreover, it can perform feature selection considering each feature dimension\u0027s relevance to the target task, which is unattainable by most neural network-based IB methods. We propose an exploration method based on Drop-Bottleneck for reinforcement learning tasks. In a multitude of noisy and reward sparse maze navigation tasks in VizDoom (Kempka et al., 2016) and DMLab (Beattie et al., 2016), our exploration method achieves state-of-the-art performance. As a new IB framework, we demonstrate that Drop-Bottleneck outperforms Variational Information Bottleneck (VIB) (Alemi et al., 2017) in multiple aspects including adversarial robustness and dimensionality reduction.
更多查看译文
关键词
discrete compressed representation,exploration,learning,drop-bottleneck,noise-robust
AI 理解论文
溯源树
样例
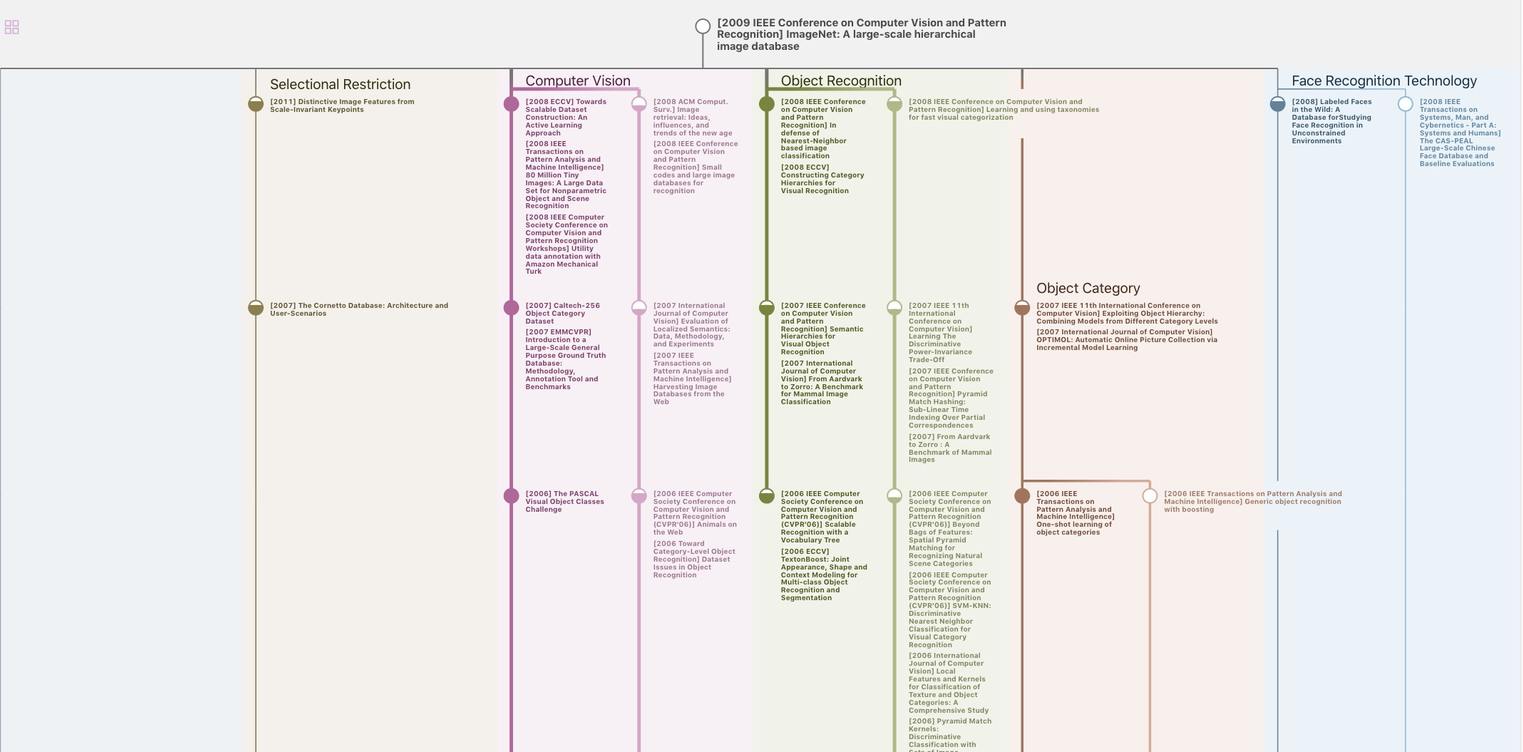
生成溯源树,研究论文发展脉络
Chat Paper
正在生成论文摘要