Model-based micro-data reinforcement learning: what are the crucial model properties and which model to choose?
ICLR(2021)
摘要
We contribute to micro-data model-based reinforcement learning (MBRL) by rigorously comparing popular generative models using a fixed (random shooting) control agent. We find that on an environment that requires multimodal posterior predictives, mixture density nets outperform all other models by a large margin. When multimodality is not required, our surprising finding is that we do not need probabilistic posterior predictives: deterministic models may perform optimally but only if they are trained with a probabilistic goal, allowing heteroscedasticity at training time. Our hypothesis is that heteroscedasticity somehow alleviates long-term error accumulation which often hinders the performance of MBRL. At the methodological side, we design metrics and an experimental protocol which can be used to evaluate the various models, predicting their asymptotic performance when using them on the control problem. Using this framework, we improve the state-of-the-art sample complexity of MBRL on Acrobot by two to four folds, using an aggressive training schedule which is outside of the hyperparameter interval usually considered.
更多查看译文
关键词
micro-data micro-data,reinforcement,crucial model-based properties
AI 理解论文
溯源树
样例
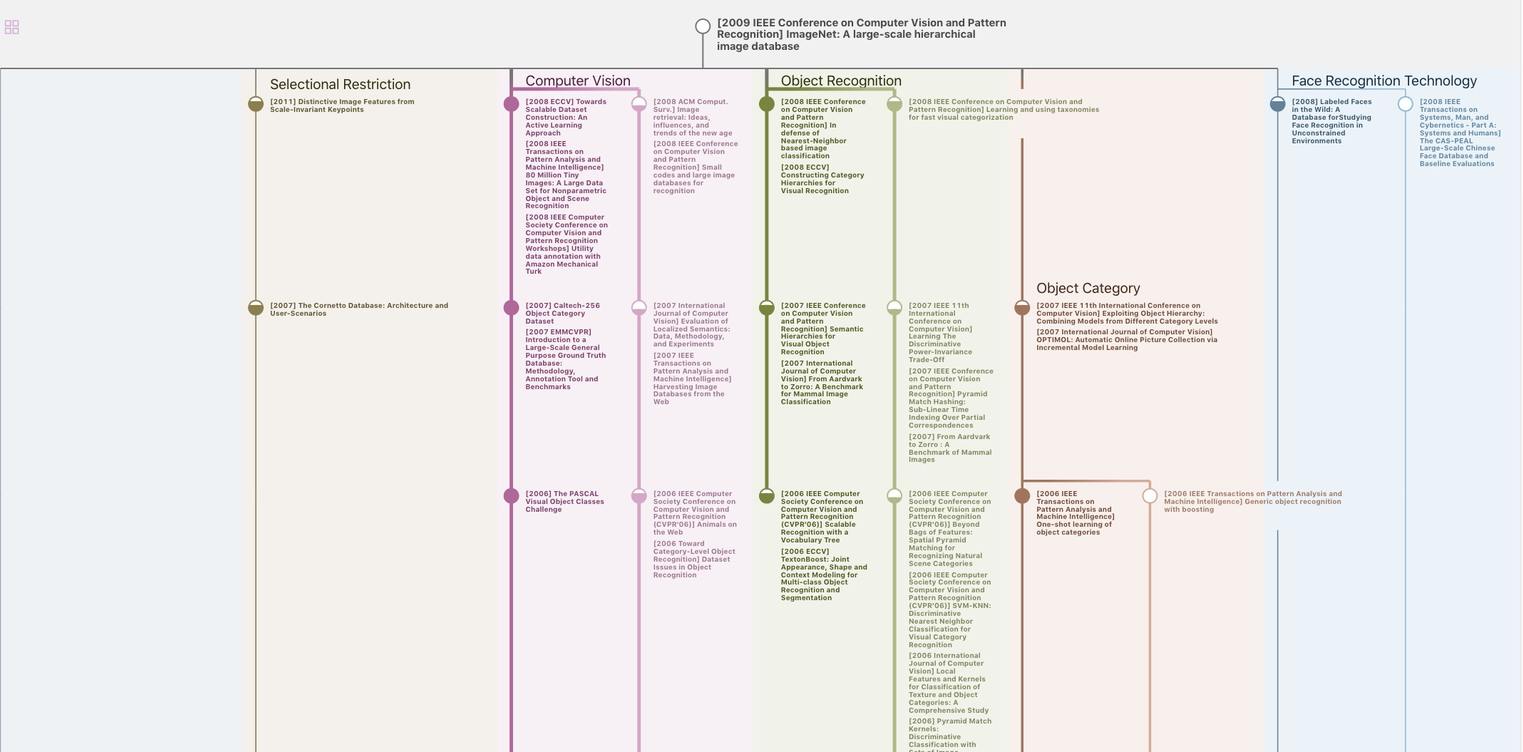
生成溯源树,研究论文发展脉络
Chat Paper
正在生成论文摘要