Generalization bounds via distillation
ICLR(2021)
摘要
This paper provides a suite of mathematical tools to bound the generalization error of networks that possess low-complexity distillations --- that is, when there exist simple networks whose softmax outputs approximately match those of the original network. The primary contribution is the aforementioned bound, which upper bounds the test error of a network by the sum of its training error, the distillation error, and the complexity of the distilled network. Supporting this, secondary contributions include: a generalization bound which can handle convolutions and skip connections, a generalization analysis of the compression step leading to a bound with small width- and depth-dependence via weight matrix stable ranks, and a sampling theorem to sparsify dense networks. The bounds and their behavior are illustrated empirically on the standard mnist and cifar datasets.
更多查看译文
关键词
bounds
AI 理解论文
溯源树
样例
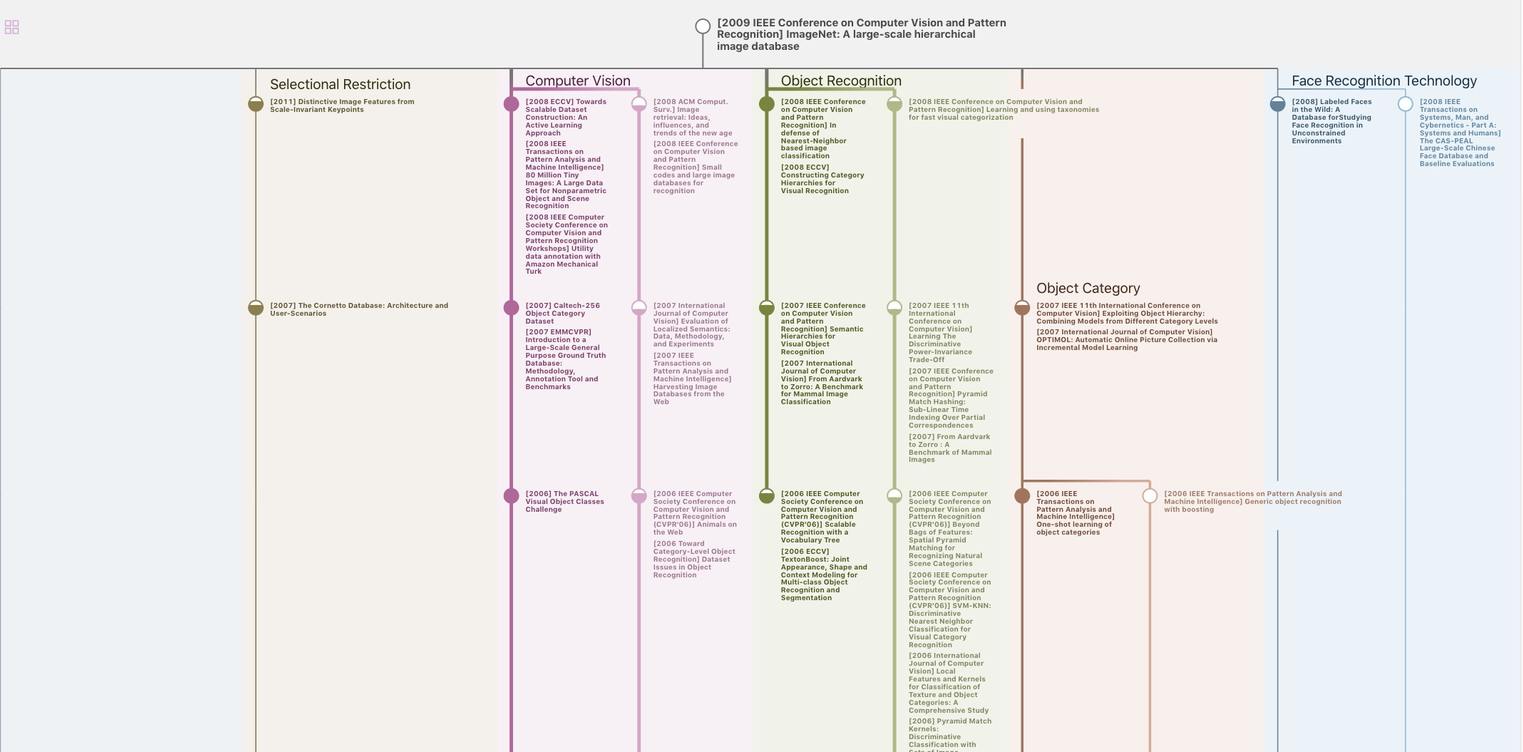
生成溯源树,研究论文发展脉络
Chat Paper
正在生成论文摘要