A metabolite‐based machine learning approach to diagnose Alzheimer‐type dementia in blood: Results from the European Medical Information Framework for Alzheimer disease …
Alzheimer's & Dementia: Translational Research & Clinical Interventions(2019)
Abstract
Introduction Machine learning (ML) may harbor the potential to capture the metabolic complexity in Alzheimer Disease (AD). Here we set out to test the performance of metabolites in blood to categorize AD when compared to CSF biomarkers. Methods This study analyzed samples from 242 cognitively normal (CN) people and 115 with AD‐type dementia utilizing plasma metabolites (n = 883). Deep Learning (DL), Extreme Gradient Boosting (XGBoost) and Random Forest (RF) were used to differentiate AD from CN. These models were internally validated using Nested Cross Validation (NCV). Results On the test data, DL produced the AUC of 0.85 (0.80–0.89), XGBoost produced 0.88 (0.86–0.89) and RF produced 0.85 (0.83–0.87). By comparison, CSF measures of amyloid, p‐tau and t‐tau (together with age and gender) produced with XGBoost the AUC values of 0.78, 0.83 and 0.87, respectively …
MoreTranslated text
AI Read Science
Must-Reading Tree
Example
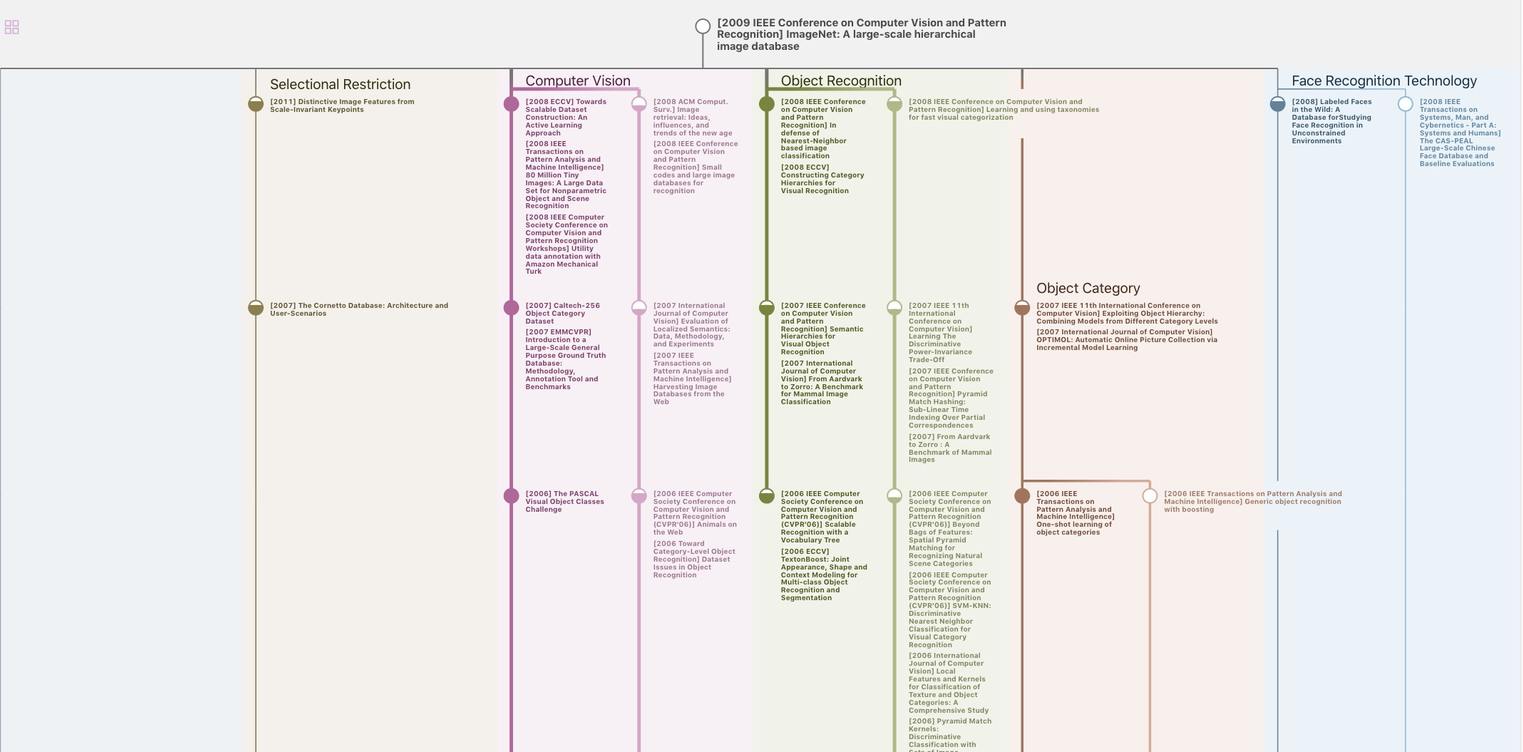
Generate MRT to find the research sequence of this paper
Chat Paper
Summary is being generated by the instructions you defined