Enabling Realistic Health Data Re-Identification Risk Assessment Through Adversarial Modeling
JOURNAL OF THE AMERICAN MEDICAL INFORMATICS ASSOCIATION(2021)
摘要
Objective: Re-identification risk methods for biomedical data often assume a worst case, in which attackers know all identifiable features (eg, age and race) about a subject. Yet, worst-case adversarial modeling can overestimate risk and induce heavy editing of shared data. The objective of this study is to introduce a framework for assessing the risk considering the attacker's resources and capabilities.Materials and Methods: We integrate 3 established risk measures (ie, prosecutor, journalist, and marketer risks) and compute re-identification probabilities for data subjects. This probability is dependent on an attacker's capabilities (eg, ability to obtain external identified resources) and the subject's decision on whether to reveal their participation in a dataset. We illustrate the framework through case studies using data from over 1 000 000 patients from Vanderbilt University Medical Center and show how re-identification risk changes when attackers are pragmatic and use 2 known resources for attack: (1) voter registration lists and (2) social media posts.Results: Our framework illustrates that the risk is substantially smaller in the pragmatic scenarios than in the worst case. Our experiments yield a median worst-case risk of 0.987 (where 0 is least risky and 1 is most risky); however, the median reduction in risk was 90.1% in the voter registration scenario and 100% in the social media posts scenario. Notably, these observations hold true for a wide range of adversarial capabilities.Conclusions: This research illustrates that re-identification risk is situationally dependent and that appropriate adversarial modeling may permit biomedical data sharing on a wider scale than is currently the case.
更多查看译文
关键词
health data, data sharing, data privacy, re-identification risk
AI 理解论文
溯源树
样例
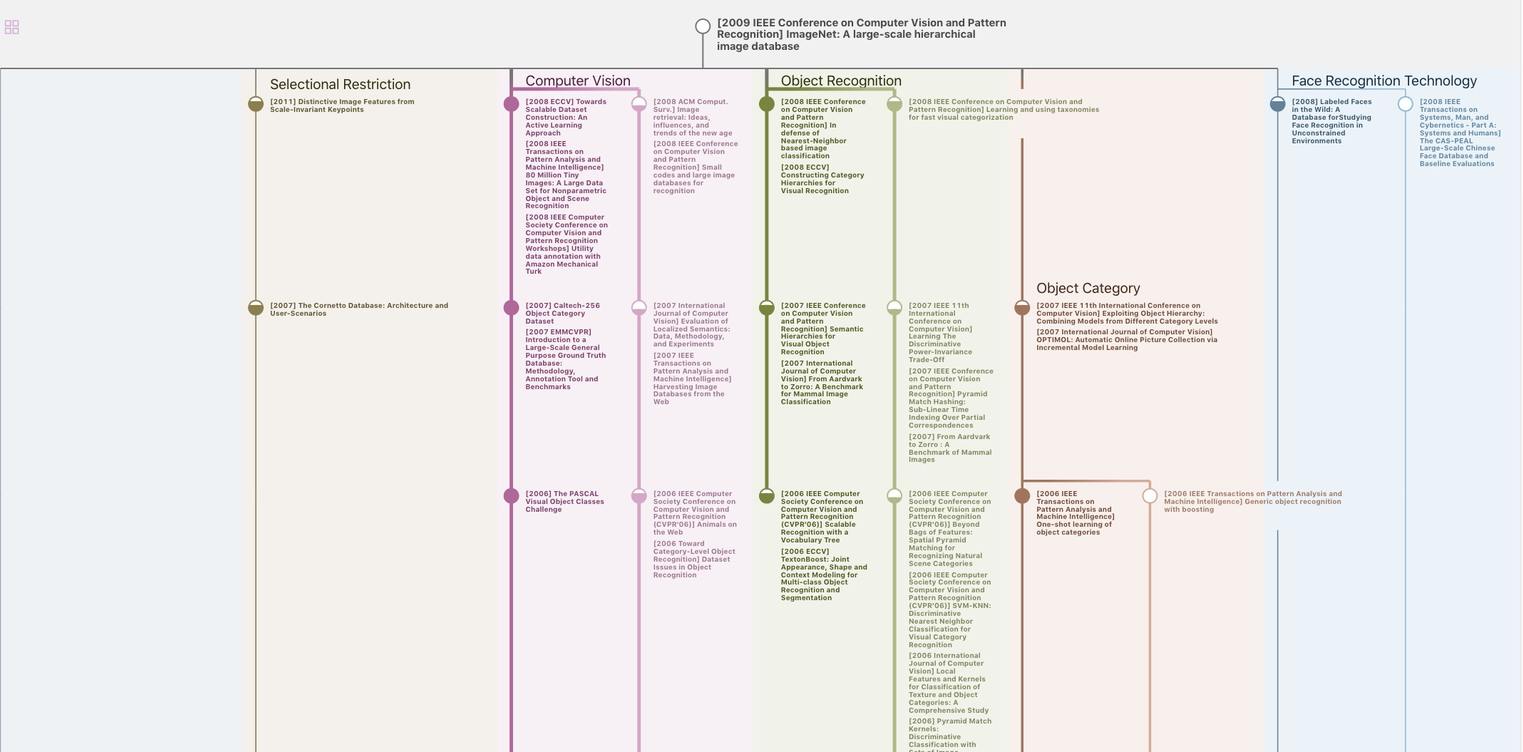
生成溯源树,研究论文发展脉络
Chat Paper
正在生成论文摘要