Separation Of Color Channels From Conventional Colonoscopy Images Improves Deep Neural Network Detection Of Polyps
JOURNAL OF BIOMEDICAL OPTICS(2021)
Abstract
Significance: Colorectal cancer incidence has decreased largely due to detection and removal of polyps. Computer-aided diagnosis development may improve on polyp detection and discrimination.Aim: To advance detection and discrimination using currently available commercial colonoscopy systems, we developed a deep neural network (DNN) separating the color channels from images acquired under narrow-band imaging (NBI) and white-light endoscopy (WLE).Approach: Images of normal colon mucosa and polyps from colonoscopies were studied. Each color image was extracted based on the color channel: red/green/blue. A multilayer DNN was trained using one-channel, two-channel, and full-color images. The trained DNN was then tested for performance in detection of polyps.Results: The DNN performed better using full-colored NBI over WLE images in the detection of polyps. Furthermore, the DNN performed better using the two-channel red + green images when compared to full-color WLE images.Conclusions: The separation of color channels from full-color NBI and WLE images taken from commercially available colonoscopes may improve the ability of the DNN to detect and discriminate polyps. Further studies are needed to better determine the color channels and combination of channels to include and exclude in DNN development for clinical use. (C) The Authors. Published by SPIE under a Creative Commons Attribution 4.0 Unported License.
MoreTranslated text
Key words
artificial intelligence algorithms, deep learning, polyp discrimination, colorectal cancer, narrow-band imaging, color channel separation
AI Read Science
Must-Reading Tree
Example
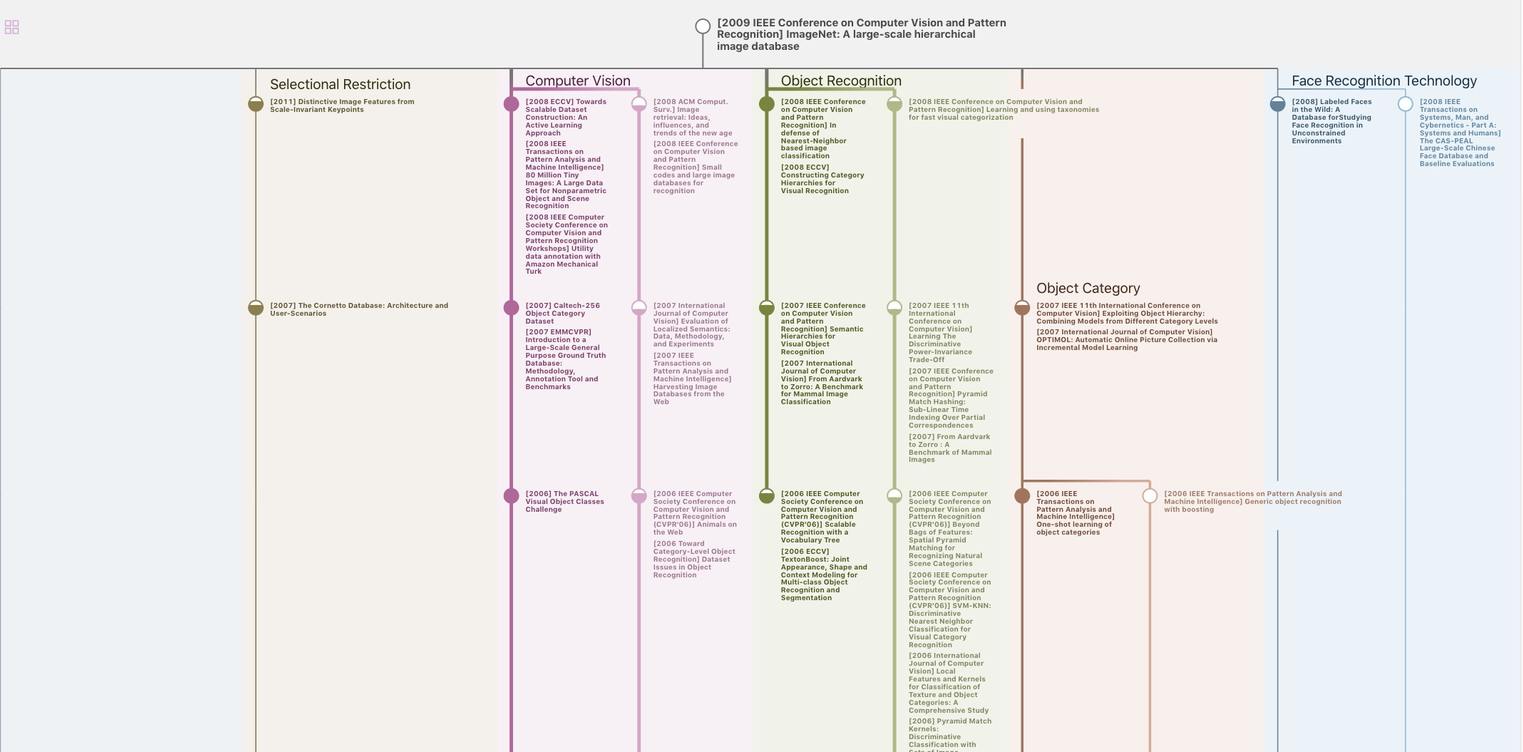
Generate MRT to find the research sequence of this paper
Chat Paper
Summary is being generated by the instructions you defined