Robust Detection of Network Intrusion using Tree-based Convolutional Neural Networks
CODS-COMAD 2021: PROCEEDINGS OF THE 3RD ACM INDIA JOINT INTERNATIONAL CONFERENCE ON DATA SCIENCE & MANAGEMENT OF DATA (8TH ACM IKDD CODS & 26TH COMAD)(2021)
摘要
Automated Intrusion Detection Systems (IDS) are the first line of defense that monitor network activity to profile and identify suspicious activity. This detection of intrusion is further complicated due to the emergence of sophisticated network based attacks that are difficult to identify. Deep learning approaches have proven to be effective in isolating such attacks through efficient identification of non-linear relationships in data. In this work, we propose a hierarchical Convolutional Neural Network approach, TreeNets, that can be used as an IDS to identify the attacks and segregate them into binary outcomes. The paper depicts the usage of Binary Grey Wolf Optimization approach for identifying the optimal set of features. We exhibit three variants of TreeNets and compare their performance against state of the art machine learning and deep learning models on the NSLKDD dataset. Experimental results depict a competitive performance with an accuracy of 82.16% and 66.37% on KDDTest+ and KDD-Test-21 respectively.
更多查看译文
关键词
Intrusion detection, Tree Convolutional Networks, NSLKDD, Deep learning, Binary Grey Wolf Optimization
AI 理解论文
溯源树
样例
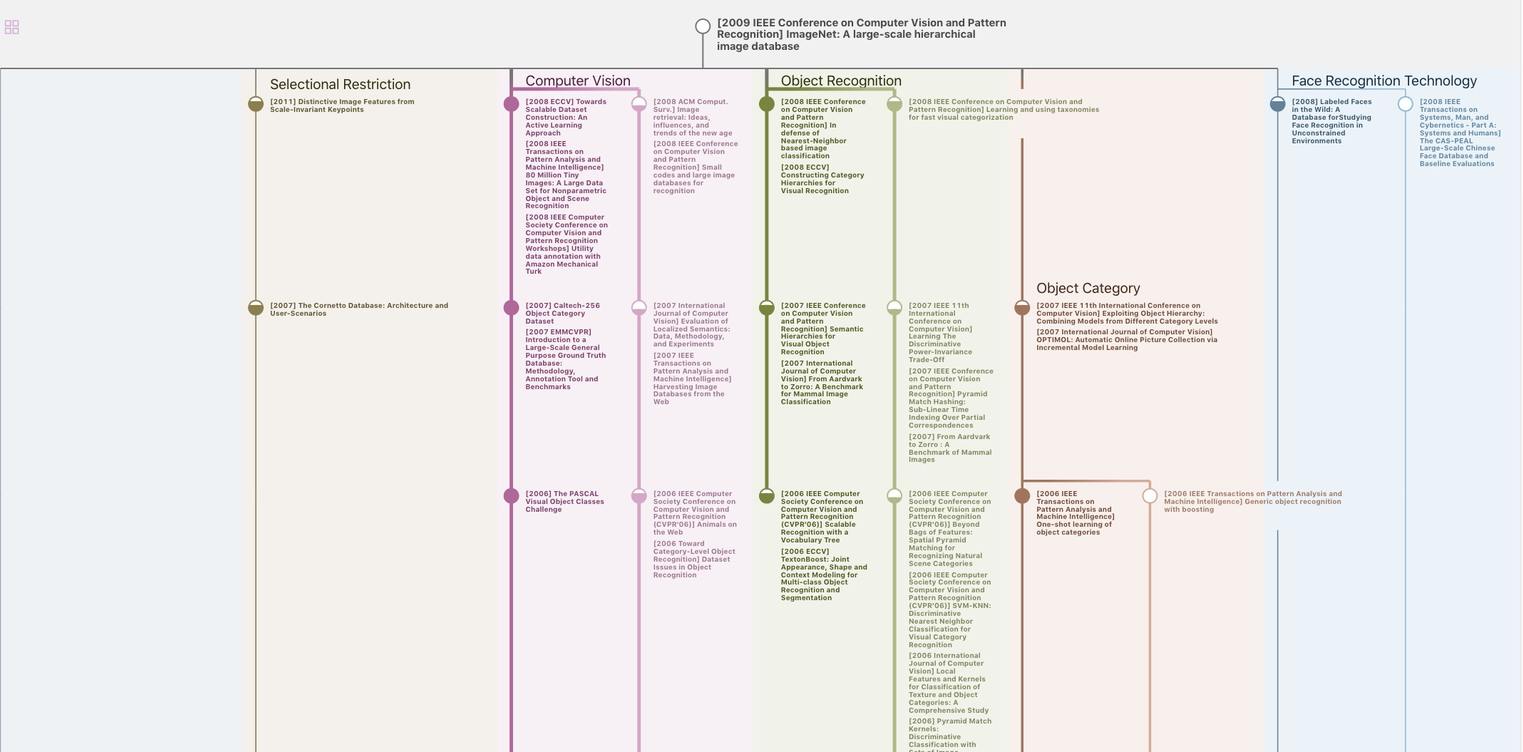
生成溯源树,研究论文发展脉络
Chat Paper
正在生成论文摘要