ROBUST BAYESIAN INFERENCE FOR BIG DATA: COMBINING SENSOR-BASED RECORDS WITH TRADITIONAL SURVEY DATA
ANNALS OF APPLIED STATISTICS(2022)
摘要
Big Data often presents as massive nonprobability samples. Not only is the selection mechanism often unknown but larger data volume amplifies the relative contribution of selection bias to total error. Existing bias adjustment approaches assume that the conditional mean structures have been correctly specified for the selection indicator or key substantive measures. In the presence of a reference probability sample, these methods rely on a pseudolike-lihood method to account for the sampling weights of the reference sample, which is parametric in nature. Under a Bayesian framework, handling the sampling weights is an even bigger hurdle. To further protect against model misspecification, we expand the idea of double robustness such that more flexible nonparametric methods as well as Bayesian models can be used for prediction. In particular, we employ Bayesian additive regression trees which not only capture nonlinear associations automatically but permit direct quantification of the uncertainty of point estimates through its posterior predictive draws. We apply our method to sensor-based naturalistic driving data from the second Strategic Highway Research Program using the 2017 National Household Travel Survey as a benchmark.
更多查看译文
关键词
Big Data, nonprobability sample, quasi-randomization, prediction model, doubly robust, augmented inverse propensity weighting, Bayesian additive regression trees
AI 理解论文
溯源树
样例
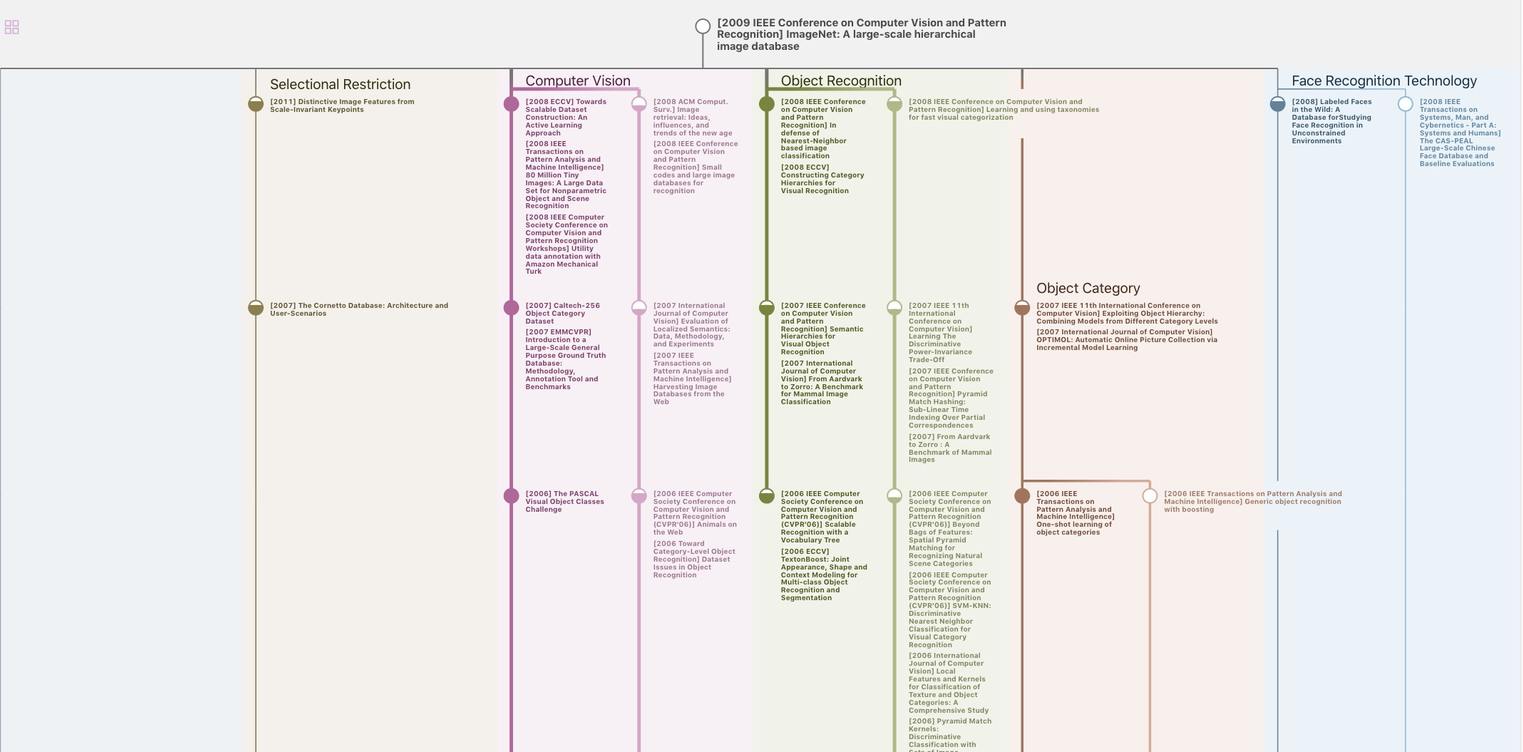
生成溯源树,研究论文发展脉络
Chat Paper
正在生成论文摘要