Randomised preconditioning for the forcing formulation of weak-constraint 4D-Var
QUARTERLY JOURNAL OF THE ROYAL METEOROLOGICAL SOCIETY(2021)
摘要
There is growing awareness that errors in the model equations cannot be ignored in data assimilation methods such as four-dimensional variational assimilation (4D-Var). If allowed for, more information can be extracted from observations, longer time windows are possible, and the minimisation process is easier, at least in principle. Weak-constraint 4D-Var estimates the model error and minimises a series of quadratic cost functions, which can be achieved using the conjugate gradient (CG) method; minimising each cost function is called an inner loop. CG needs preconditioning to improve its performance. In previous work, limited-memory preconditioners (LMPs) have been constructed using approximations of the eigenvalues and eigenvectors of the Hessian in the previous inner loop. If the Hessian changes significantly in consecutive inner loops, the LMP may be of limited usefulness. To circumvent this, we propose using randomised methods for low-rank eigenvalue decomposition and use these approximations to construct LMPs cheaply using information from the current inner loop. Three randomised methods are compared. Numerical experiments in idealized systems show that the resulting LMPs perform better than the existing LMPs. Using these methods may allow more efficient and robust implementations of incremental weak-constraint 4D-Var.
更多查看译文
关键词
data assimilation, limited-memory preconditioners, randomised methods, sparse symmetric positive-definite systems, weak-constraint 4D-Var
AI 理解论文
溯源树
样例
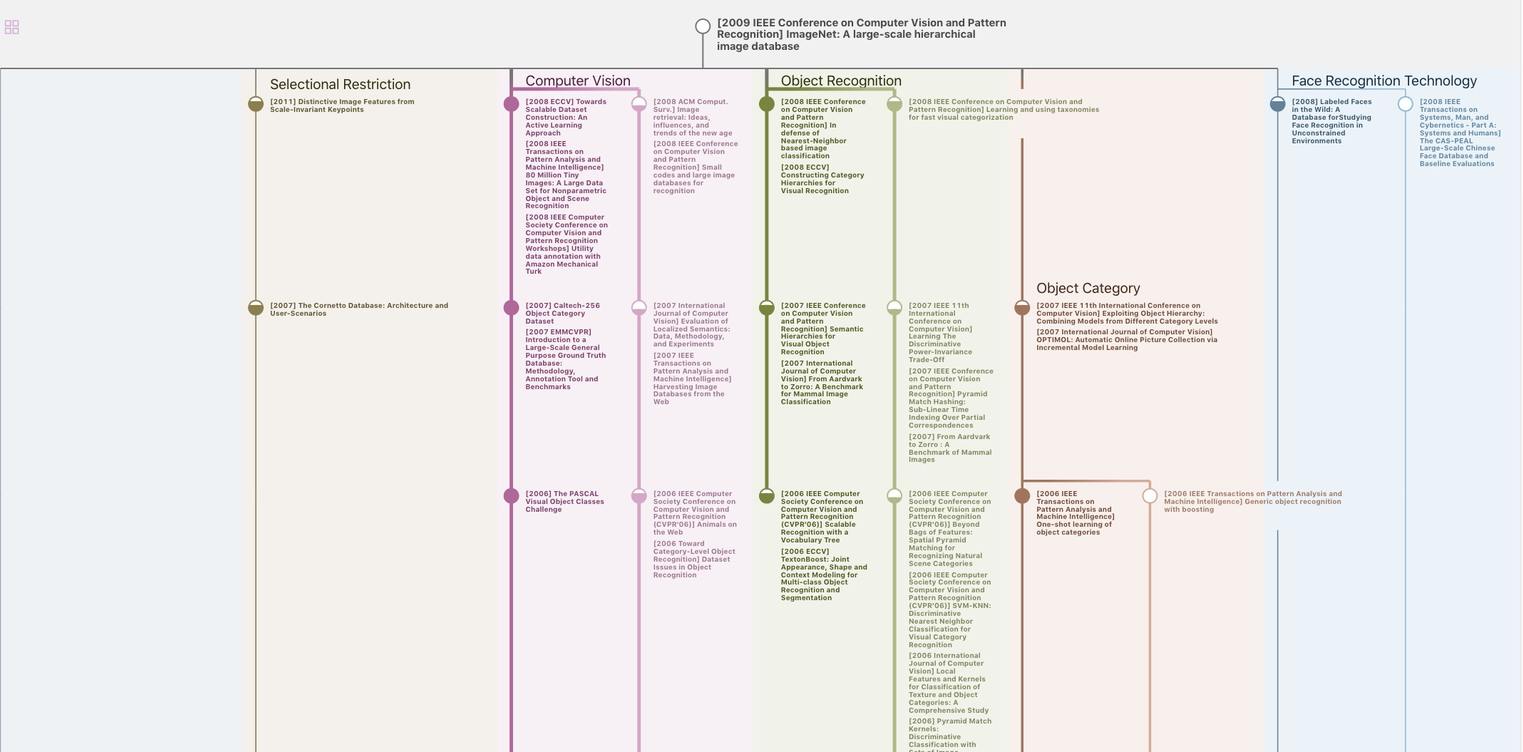
生成溯源树,研究论文发展脉络
Chat Paper
正在生成论文摘要