Context-Aware Deep Representation Learning for Geo-Spatiotemporal Analysis
2020 IEEE International Conference on Data Mining (ICDM)(2020)
摘要
The emergence of remote sensing technologies coupled with local monitoring workstations enables us the unprecedented ability to monitor the environment in large scale. Information mining from multi-channel geo-spatiotemporal data however poses great challenges to many computational sustainability applications. Most existing approaches adopt various dimensionality reduction techniques without fully taking advantage of the spatiotemporal nature of the data. In addition, the lack of labeled training data raises another challenge for modeling such data. In this work, we propose a novel semi-supervised attention-based deep representation model that learns context-aware spatiotemporal representations for prediction tasks. A combination of convolutional neural networks with a hybrid attention mechanism is adopted to extract spatial and temporal variations in the geo-spatiotemporal data. Recognizing the importance of capturing more complete temporal dependencies, we propose the hybrid attention mechanism which integrates a learnable global query into the classic self-attention mechanism. To overcome the data scarcity issue, sampled spatial and temporal context that naturally reside in the largely-available unlabeled geo-spatiotemporal data are exploited to aid meaningful representation learning. We conduct experiments on a large-scale real-world crop yield prediction task. The results show that our methods significantly outperforms existing state-of-the-art yield prediction methods, especially under the stress of training data scarcity.
更多查看译文
关键词
Spatiotemporal Prediction,Semi-supervised Learning,Attention,Transformer,Crop Yield Prediction
AI 理解论文
溯源树
样例
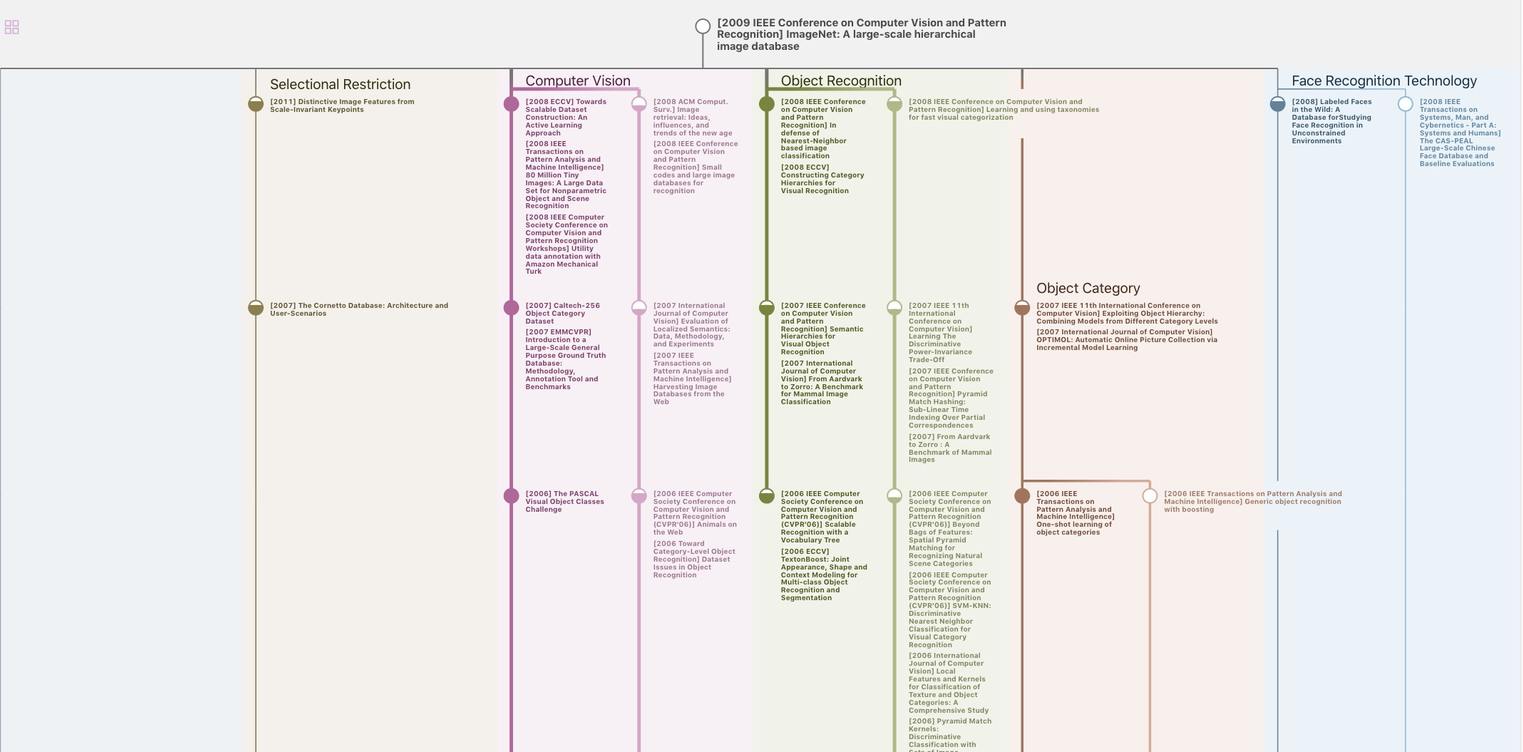
生成溯源树,研究论文发展脉络
Chat Paper
正在生成论文摘要