LIME: Learning Inductive Bias for Primitives of Mathematical Reasoning
INTERNATIONAL CONFERENCE ON MACHINE LEARNING, VOL 139(2021)
摘要
While designing inductive bias in neural architectures has been widely studied, we hypothesize that transformer networks are flexible enough to learn inductive bias from suitable generic tasks. Here, we replace architecture engineering by encoding inductive bias in the form of datasets. Inspired by Peirce's view that deduction, induction, and abduction are the primitives of reasoning, we design three synthetic tasks that are intended to require the model to have these three abilities. We specifically design these tasks to be synthetic and devoid of mathematical knowledge to ensure that only the fundamental reasoning biases can be learned from these tasks. This defines a new pre-training methodology called "LIME" (Learning Inductive bias for Mathematical rEasoning). Models trained with LIME significantly outperform vanilla transformers on four very different large mathematical reasoning benchmarks. Unlike dominating the computation cost as traditional pre-training approaches, LIME requires only a small fraction of the computation cost of the typical downstream task. The code for generating LIME tasks is available at https://github.com/tonywu9/LIME.
更多查看译文
关键词
inductive bias,primitives,reasoning,learning
AI 理解论文
溯源树
样例
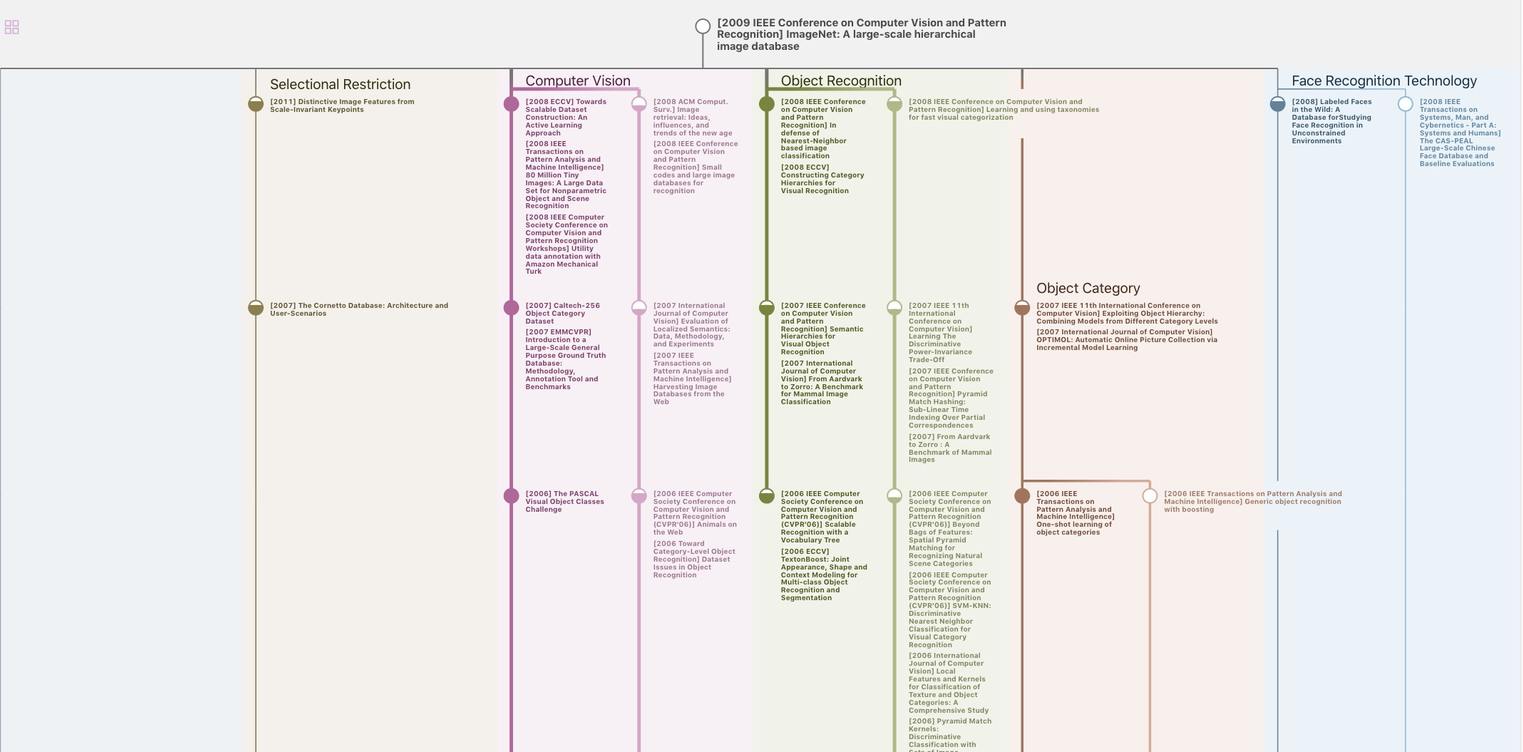
生成溯源树,研究论文发展脉络
Chat Paper
正在生成论文摘要