Supervised Transfer Learning at Scale for Medical Imaging
arxiv(2021)
摘要
Transfer learning is a standard technique to improve performance on tasks with limited data. However, for medical imaging, the value of transfer learning is less clear. This is likely due to the large domain mismatch between the usual natural-image pre-training (e.g. ImageNet) and medical images. However, recent advances in transfer learning have shown substantial improvements from scale. We investigate whether modern methods can change the fortune of transfer learning for medical imaging. For this, we study the class of large-scale pre-trained networks presented by Kolesnikov et al. on three diverse imaging tasks: chest radiography, mammography, and dermatology. We study both transfer performance and critical properties for the deployment in the medical domain, including: out-of-distribution generalization, data-efficiency, sub-group fairness, and uncertainty estimation. Interestingly, we find that for some of these properties transfer from natural to medical images is indeed extremely effective, but only when performed at sufficient scale.
更多查看译文
关键词
supervised transfer learning,medical imaging
AI 理解论文
溯源树
样例
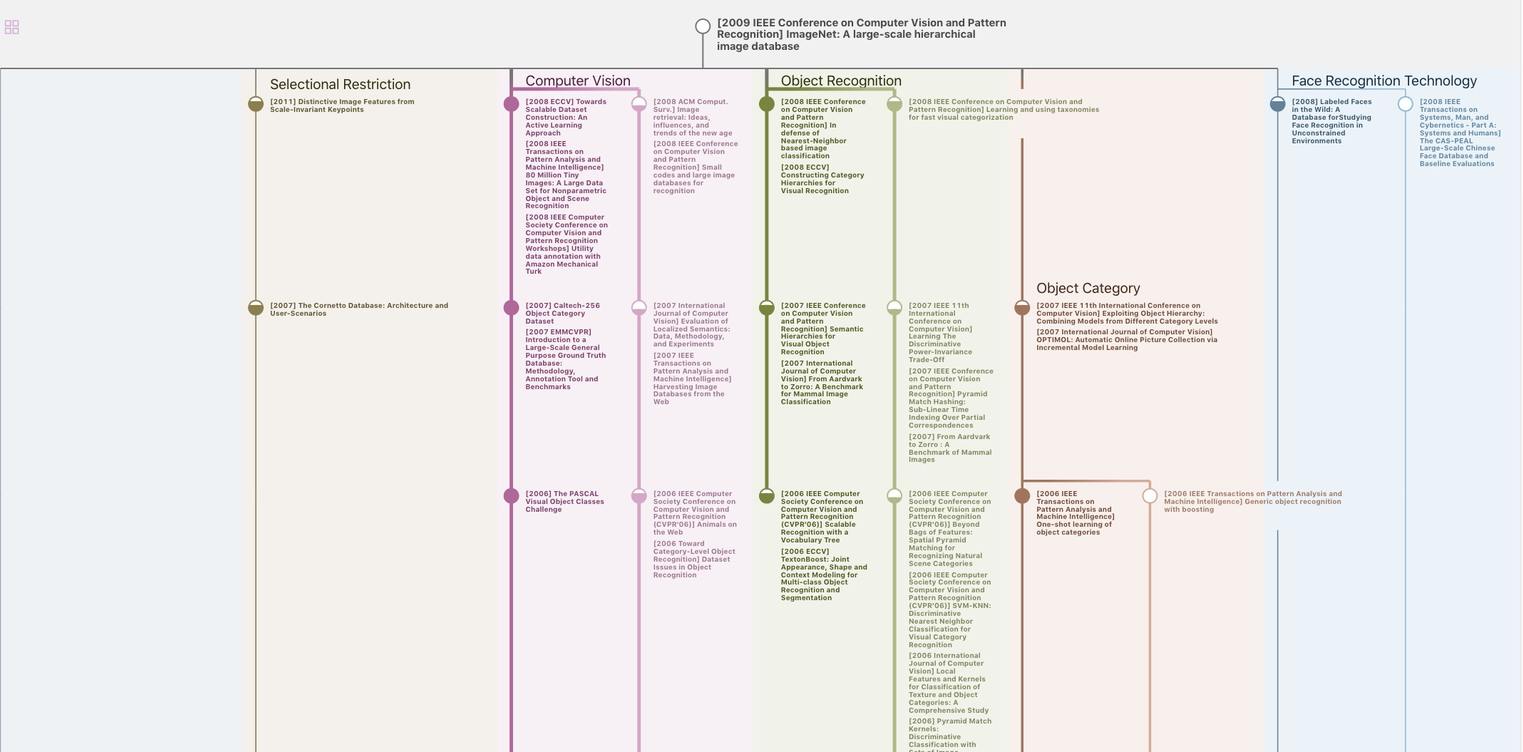
生成溯源树,研究论文发展脉络
Chat Paper
正在生成论文摘要