Input Optimisation Network for Semantic Segmentation of Underexposed Images
2020 IEEE International Symposium on Safety, Security, and Rescue Robotics (SSRR)(2020)
摘要
Autonomous Vehicles are required to operate robustly across widely varied scenarios and conditions, however environmental factors such as weather and lighting can impede the capabilities of the perception systems required for safe operation. In this work we investigate the effects lighting changes can have on semantic segmentation of urban road scenes, specifically how segmentation performance is affected by underexposed imagery. Using two publicly available datasets, we simulate incorrectly set camera exposure and compare the performance of a standard pre-trained deep semantic segmentation network on correctly and incorrectly exposed images. We then introduce a novel input optimization network, which aims to modify a given image such that it generates an optimal response from a pre-trained semantic segmentation network. We compare our approach to an adversarially-trained model and demonstrate significantly improved semantic segmentation performance over that of unoptimised images.
更多查看译文
关键词
autonomous vehicles,environmental factors,urban road scenes,input optimisation network,underexposed images,pretrained deep semantic segmentation network,camera exposure,multiple object classes,image features,road scene understanding
AI 理解论文
溯源树
样例
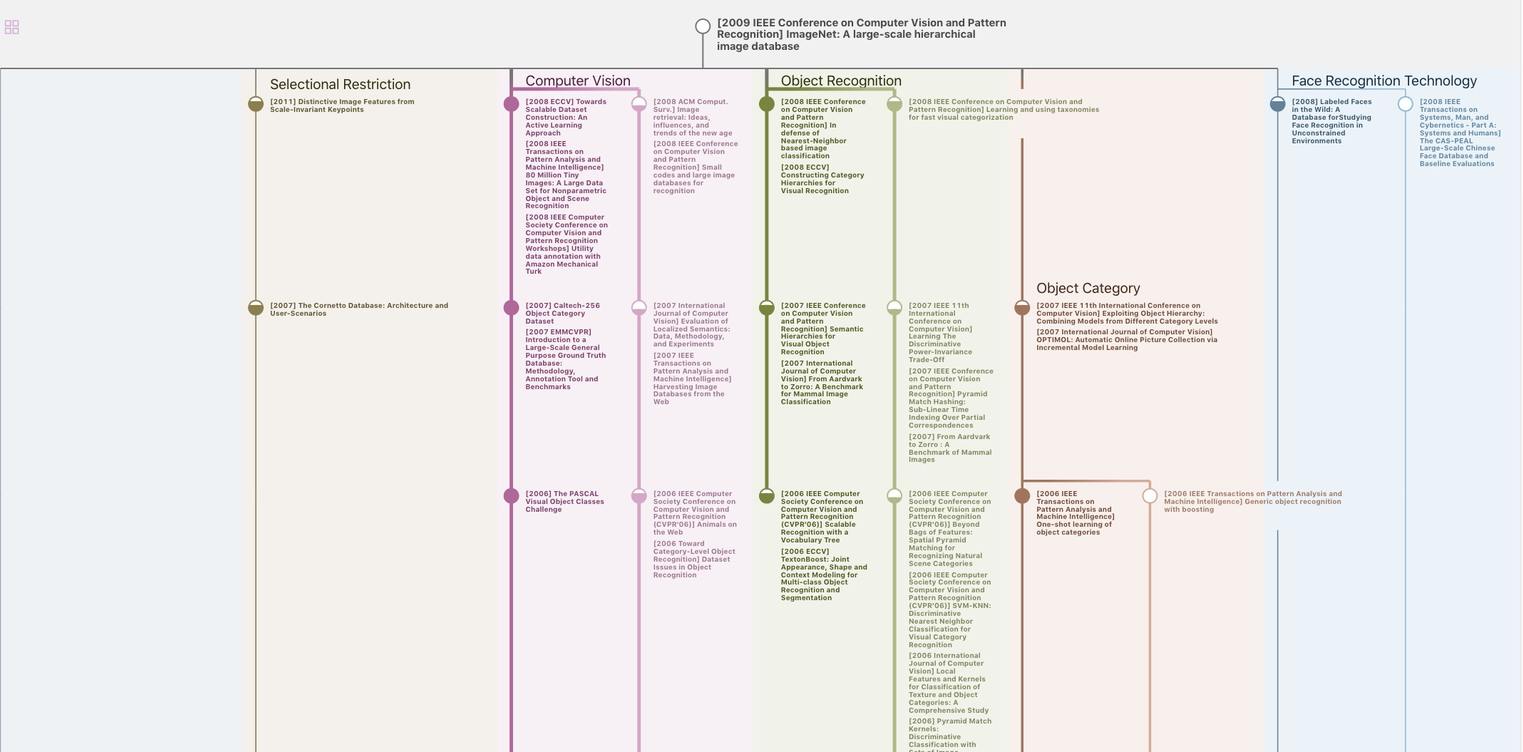
生成溯源树,研究论文发展脉络
Chat Paper
正在生成论文摘要