Generative Adversarial Networks and Transfer Learning for Non-Intrusive Load Monitoring in Smart Grids
2020 IEEE International Conference on Communications, Control, and Computing Technologies for Smart Grids (SmartGridComm)(2020)
摘要
Non-intrusive load monitoring (NILM) objective is to disaggregate the total power consumption of a building into individual appliance-level profiles. This gives insights to consumers to efficiently use energy and realizes smart grid efficiency outcomes. While many studies focus on achieving accurate models, few of them address the models generalizability. This paper proposes two approaches based on generative adversarial networks to achieve high-accuracy load disaggregation. Concurrently, the paper addresses the model generalizability in two ways, the first is by transfer learning by parameter sharing and the other is by learning compact common representations between source and target domains. This paper also quantitatively evaluate the worth of these transfer learning approaches based on the similarity between the source and target domains. The models are evaluated on three open-access datasets and outperformed recent machine-learning methods.
更多查看译文
关键词
Non-intrusive load monitoring,load disaggregation,generative adversarial networks,transfer learning
AI 理解论文
溯源树
样例
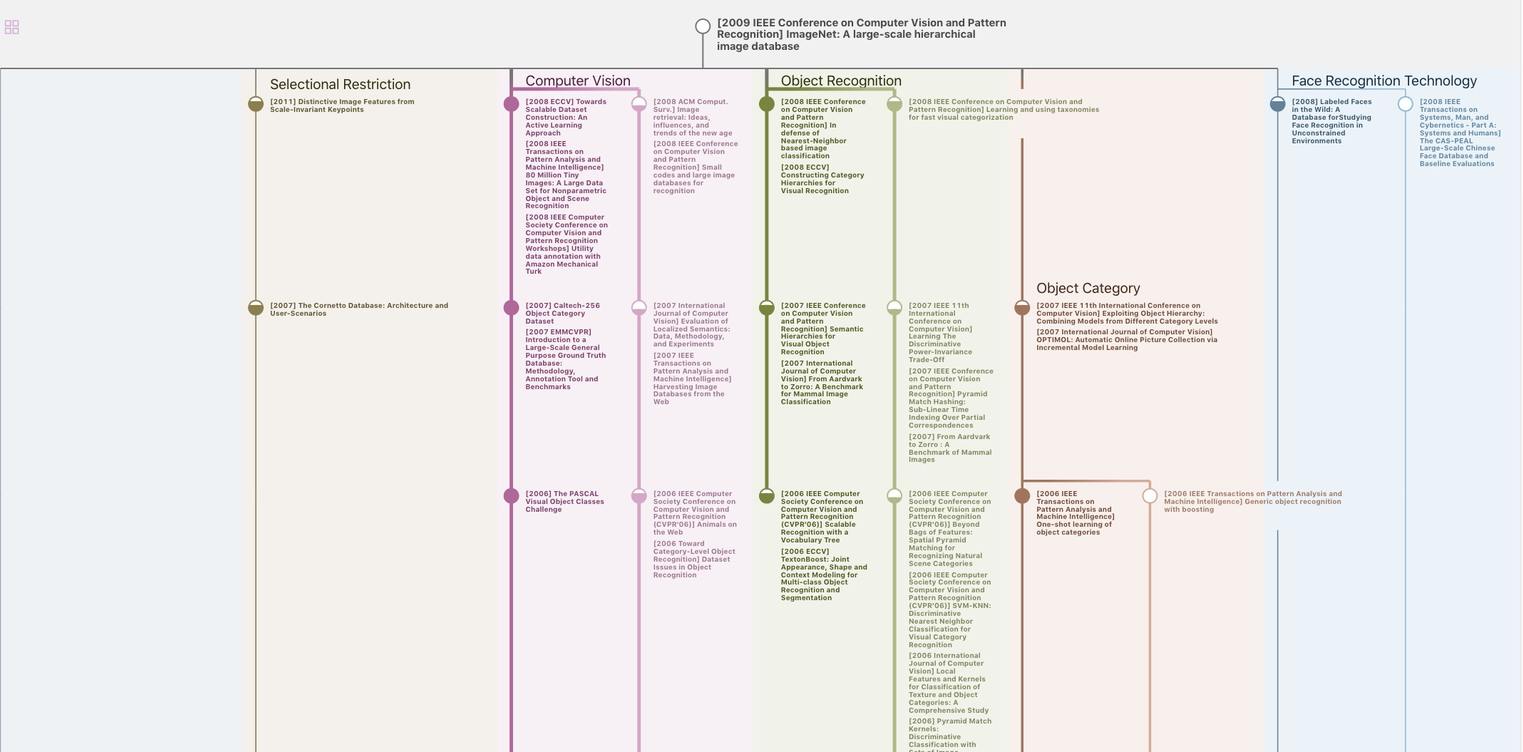
生成溯源树,研究论文发展脉络
Chat Paper
正在生成论文摘要