Reducing Sensors in Mental Imagery Based Cognitive Task for Brain Computer Interface
2020 14th International Conference on Signal Processing and Communication Systems (ICSPCS)(2020)
摘要
The performance of mental imagery based brain-computer-interfaces (BCI) can be enhanced by improving Electroencephalography (EEG) signal classification. It is known that the optimal sensors/electrodes for mental imagery applications are the C3, Cz and C4 that are not present in low cost EEG acquisition devices like the Emotiv EPOC+ headset. Hence in this paper a framework is proposed to classify mental imagery tasks using alternative and reduced number of sensors available in Emotiv EPOC+ headset. In this paper four features are extracted from EEG signals which are Band Power (BP), Approximate Entropy (ApEn), statistical features, and wavelet-based features. For classification, Decision Tree (DT), Random Forest (RF), Support Vector Machine (SVM), and K-Nearest Neighbors (KNN) are used. 100% cross validation accuracy is achieved by using BP and ApEn features and KNN Classifier with all the 14 electrodes. It is further observed that most of the information necessary for the mental imagery classification is present at the FC5, FC6, P7, P8, AF3 and AF4 electrodes. By classifying the Band Power and ApEn features from the electrodes mentioned above and using the KNN classifier, an average cross validation accuracy of 99.75% is achieved. If the same features from the FC5, FC6, AF3 and AF4 electrodes are classified using KNN, an average cross validation accuracy of 98.55% can be achieved. Hence reduced number of sensors can be used successfully for motor imagery classification. Also based on the model selected, it can be concluded that out of the four mental imagery tasks (LEFT, RIGHT, PUSH and PULL), the PULL mental imagery task is the hardest to be classified, with a classification error of 2.4%.
更多查看译文
关键词
Mental Imagery,EEG,BCI,Band Power,Approximate Entropy
AI 理解论文
溯源树
样例
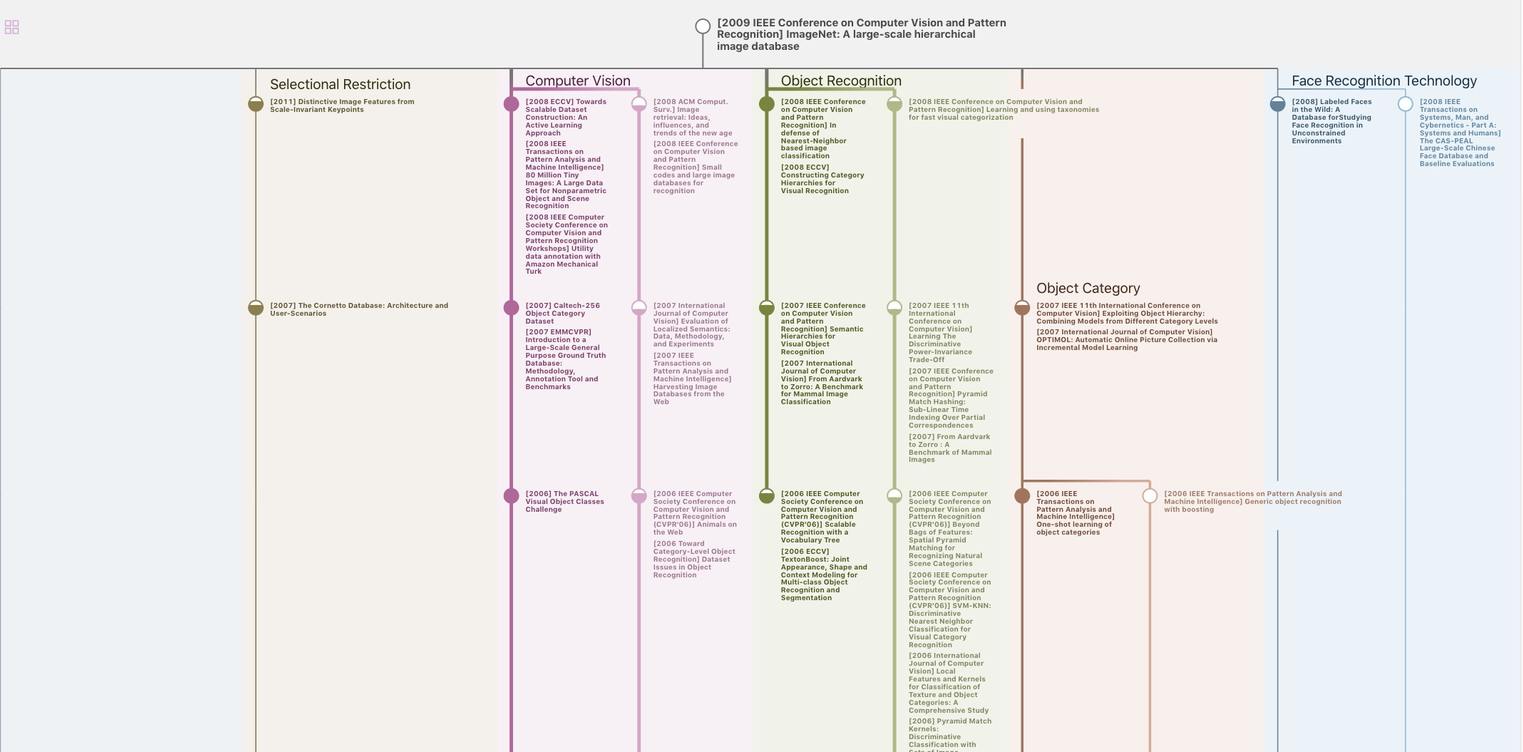
生成溯源树,研究论文发展脉络
Chat Paper
正在生成论文摘要