Automatically Resolve Trouble Tickets with Hybrid NLP.
SSCI(2020)
Abstract
Application service providers manage huge and complicated infrastructures. Like any complicated systems, things could go wrong due to various reasons (e.g. network connection response problems, infrastructure resource limitations, software malfunctioning issues, etc.) from time to time. How to quickly resolve issues when they happen becomes critical to help improve customer satisfaction and retention. Recently, fast advancement of natural language processing (NLP) algorithms have helped solving many practical problems by analyzing text information. Powerful algorithms have been developed to interpret human language and derive predictions. Ensemble models are also well suited to further improve performances as they can further explore the latent space to take advantage of features/weights discovered from a group of trained models. In this paper, we will introduce efficient machine learning algorithms to help application service providers manage and automatically resolve trouble tickets to significantly improve user experience and operational efficiency. We propose a hybrid approach to use both unsupervised clustering approach and supervised deep learning embedding to maximize the feature exploration and learning efficacy. Then we ensemble multiple optimized models to build the recommendation engine. This approach helps to incorporate the most relevant information, explore the corpus better for a given problem, managed to produce more consistent and robust predictions, and obtained better accuracy than any single model.
MoreTranslated text
Key words
NLP, Embedding, Machine Learning, Deep Learning, Telecommunication
AI Read Science
Must-Reading Tree
Example
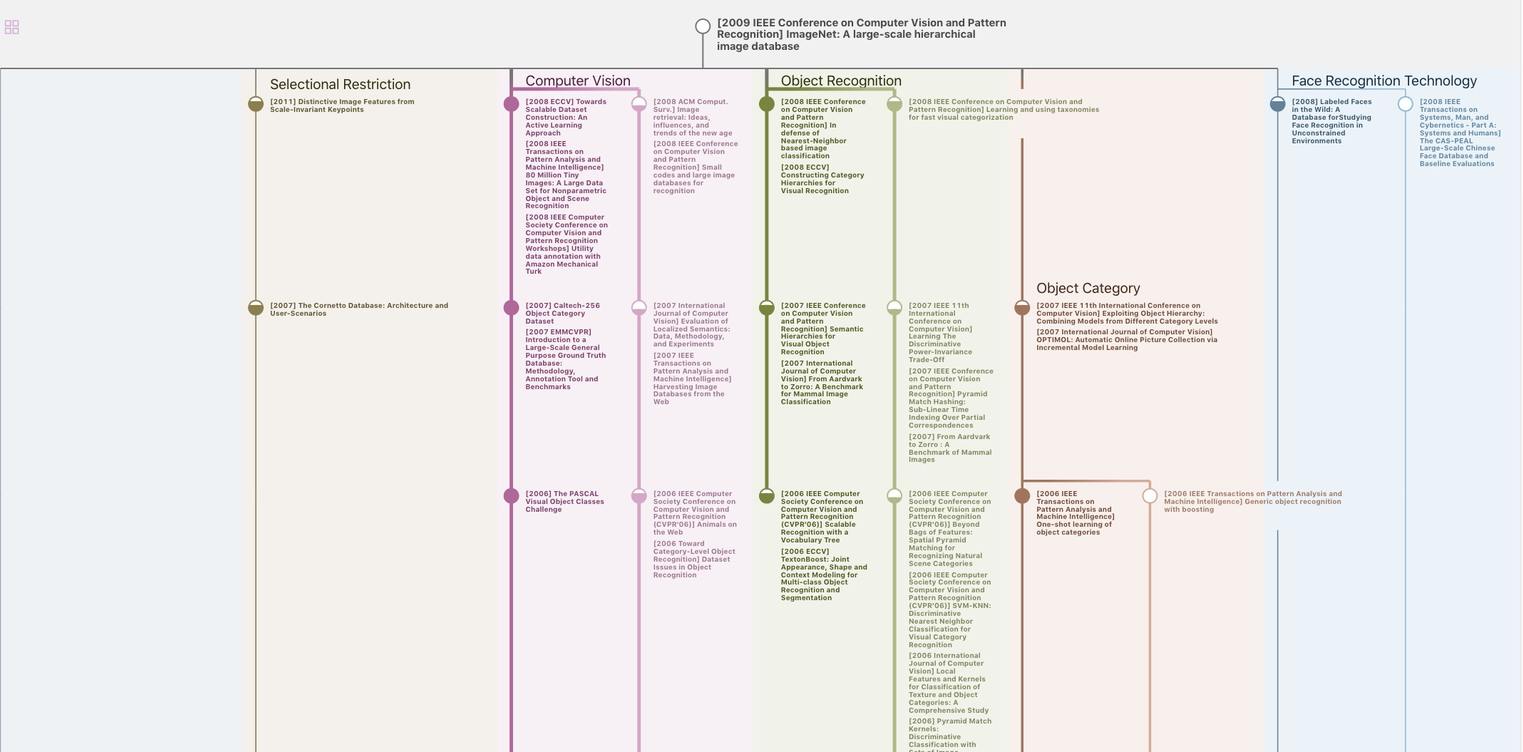
Generate MRT to find the research sequence of this paper
Chat Paper
Summary is being generated by the instructions you defined