Self-supervised Vessel Enhancement Using Flow-Based Consistencies
MEDICAL IMAGE COMPUTING AND COMPUTER ASSISTED INTERVENTION - MICCAI 2021, PT II(2021)
摘要
Vessel segmentation is an essential task in many clinical applications. Although supervised methods have achieved state-of-art performance, acquiring expert annotation is laborious and mostly limited for two-dimensional datasets with a small sample size. On the contrary, unsupervised methods rely on handcrafted features to detect tubelike structures such as vessels. However, those methods require complex pipelines involving several hyper-parameters and design choices rendering the procedure sensitive, dataset-specific, and not generalizable. We propose a self-supervised method with a limited number of hyper-parameters that is generalizable across modalities. Our method uses tube-like structure properties, such as connectivity, profile consistency, and bifurcation, to introduce inductive bias into a learning algorithm. To model those properties, we generate a vector field that we refer to as a flow. Our experiments on various public datasets in 2D and 3D show that our method performs better than unsupervised methods while learning useful transferable features from unlabeled data. Unlike generic self-supervised methods, the learned features learn vessel-relevant features that are transferable for supervised approaches, which is essential when the number of annotated data is limited.
更多查看译文
关键词
vessel enhancement,self-supervised,flow-based
AI 理解论文
溯源树
样例
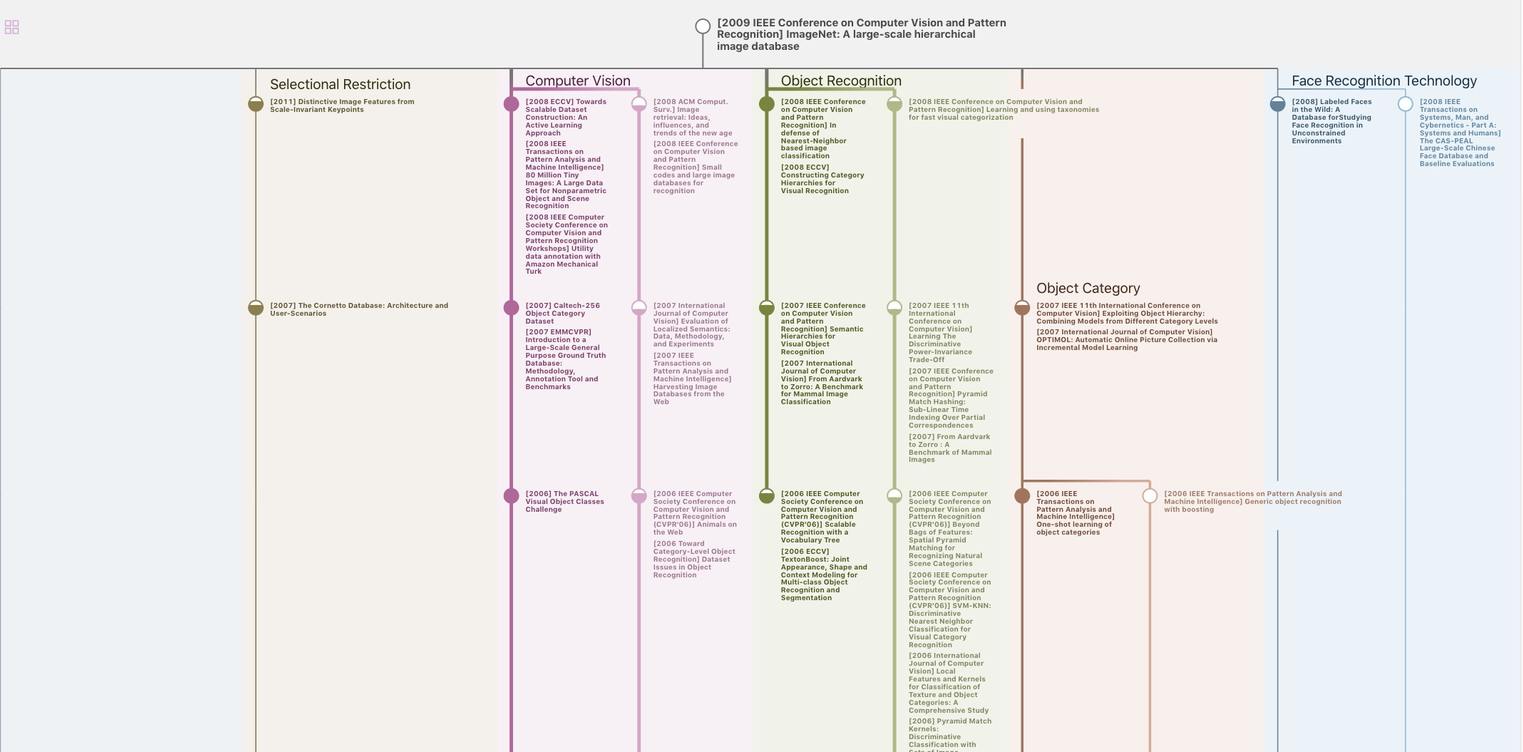
生成溯源树,研究论文发展脉络
Chat Paper
正在生成论文摘要