Reranking for Large-Scale Statistical Machine Translation
NEURAL INFORMATION PROCESSING SERIES(2009)
摘要
Statistical machine translation systems conduct a nonexhaustive search of the (extremely large) space of all possible translations by keeping a list of the current n-best candidates. In practice, it was observed that the ranking of the candidates within the n-best list can be fairly poor, which means that the system is unable to return the best of the available N translations. In this chapter we propose a novel algorithm for reranking these n-best candidates. Our approach was successfully applied to large-scale, state-of-the-art commercial systems that are trained on up to three orders of magnitude more data than previously reported in reranking studies. In order to reach this goal, we create an ensemble of rerankers that are trained in parallel, each of them using just a fraction of the available data. Our empirical evaluation on two mature language pairs, Chinese-English and French-English, shows improvements of around 0.5 and 0.2 BLEU on corpora of 80 million and 1.1 billion words, respectively.
更多查看译文
AI 理解论文
溯源树
样例
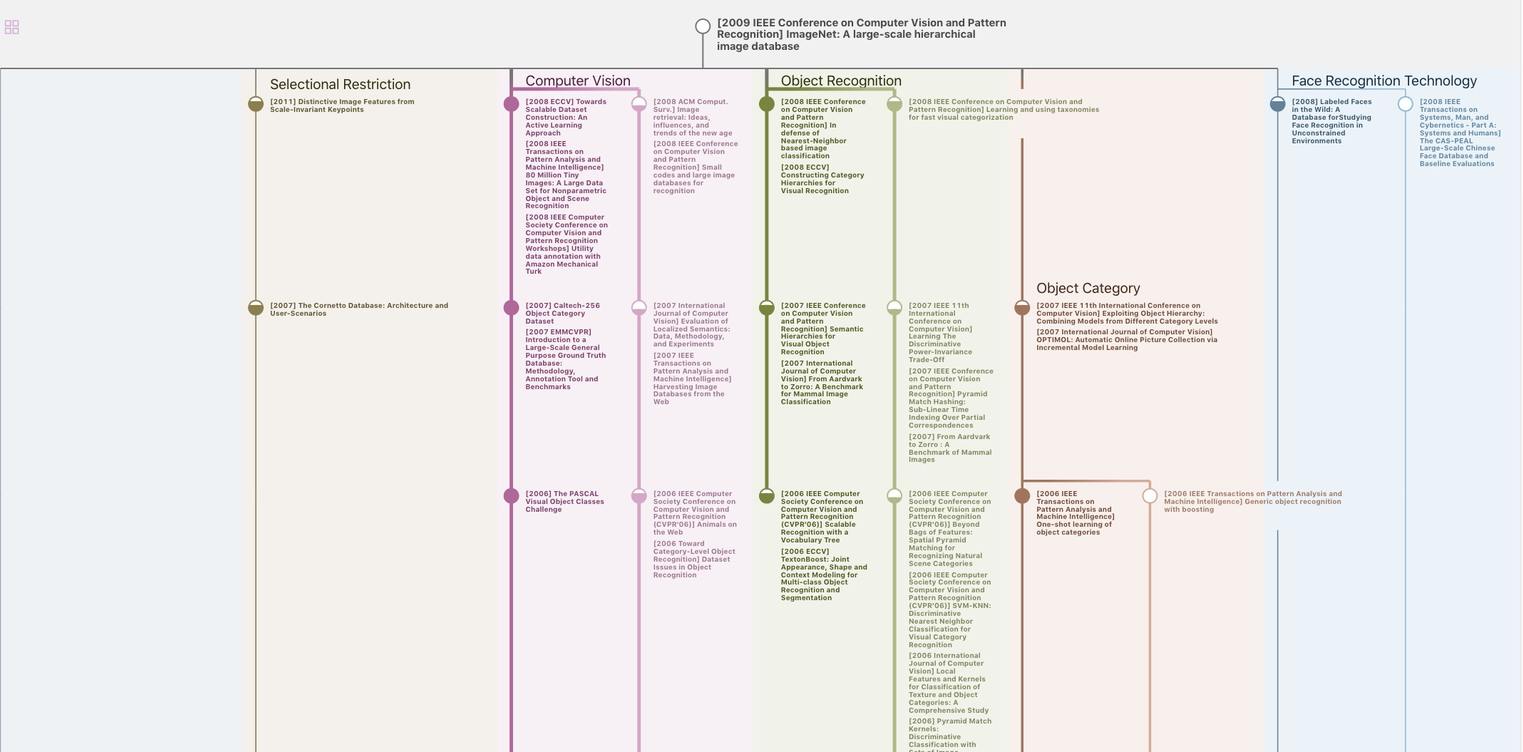
生成溯源树,研究论文发展脉络
Chat Paper
正在生成论文摘要