A Bayesian neural network predicts the dissolution of compact planetary systems
PROCEEDINGS OF THE NATIONAL ACADEMY OF SCIENCES OF THE UNITED STATES OF AMERICA(2021)
摘要
We introduce a Bayesian neural network model that can accurately predict not only if, but also when a compact planetary system with three or more planets will go unstable. Our model, trained directly from short N-body time series of raw orbital elements, is more than two orders of magnitude more accurate at predicting instability times than analytical estimators, while also reducing the bias of existing machine learning algorithms by nearly a factor of three. Despite being trained on compact resonant and near-resonant three-planet configurations, the model demonstrates robust generalization to both nonresonant and higher multiplicity configurations, in the latter case outperforming models fit to that specific set of integrations. The model computes instability estimates up to 10(5) times faster than a numerical integrator, and unlike previous efforts provides confidence intervals on its predictions. Our inference model is publicly available in the SPOCK (https://github.com/dtamayo/spock) package, with training code open sourced (https://github.com/MilesCranmer/bnn chaos model).
更多查看译文
关键词
deep learning,planetary dynamics,Bayesian analysis,chaos
AI 理解论文
溯源树
样例
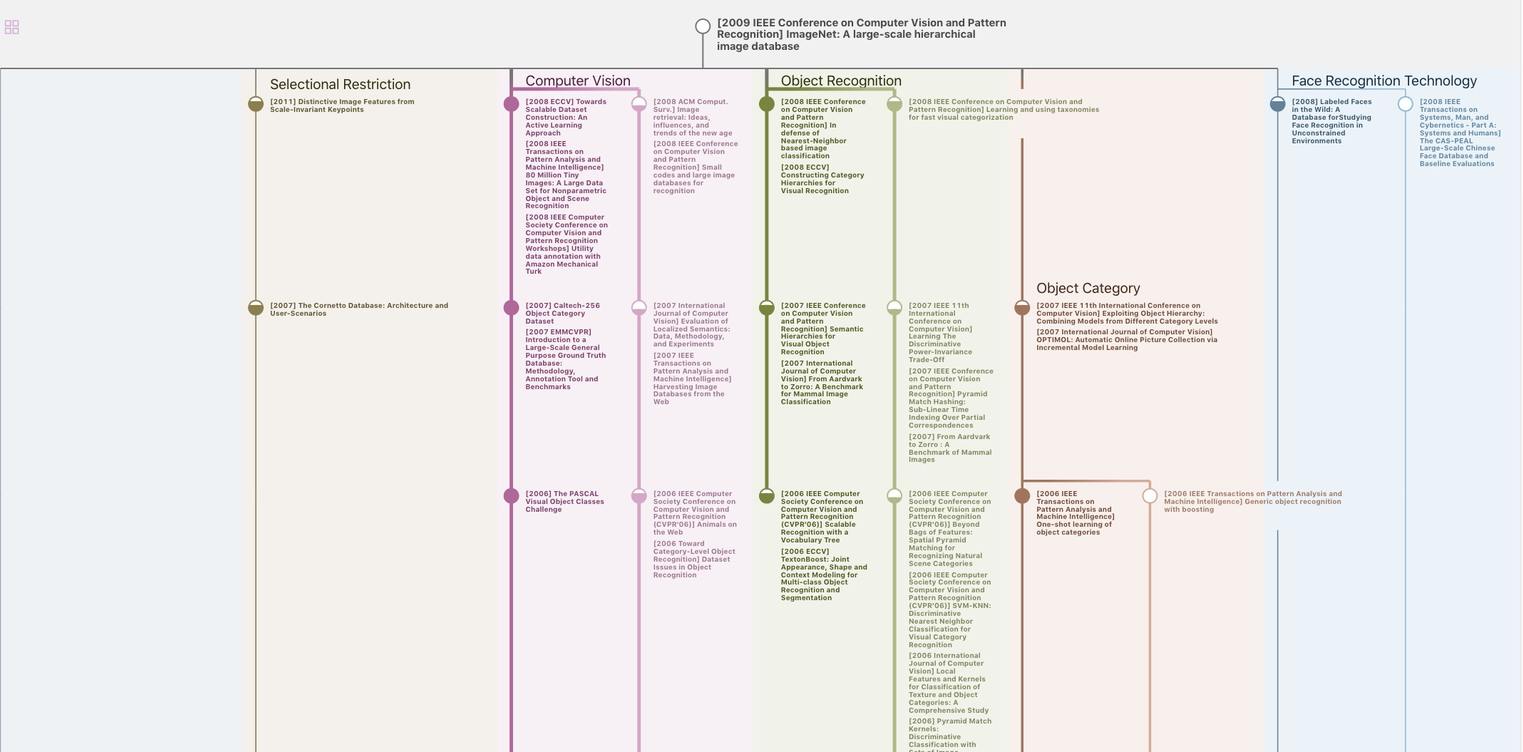
生成溯源树,研究论文发展脉络
Chat Paper
正在生成论文摘要