Generalize ultrasound image segmentation via instant and plug & play style transfer
2021 IEEE 18th International Symposium on Biomedical Imaging (ISBI)(2021)
Abstract
Deep segmentation models that generalize to images with unknown appearance are important for real-world medical image analysis. Retraining models leads to high latency and complex pipelines, which are impractical in clinical settings. The situation becomes more severe for ultrasound image analysis because of their large appearance shifts. In this paper, we propose a novel method for robust segmentation under unknown appearance shifts. Our contribution is three-fold. First, we advance a one-stage plug-and-play solution by embedding hierarchical style transfer units into a segmentation architecture. Our solution can remove appearance shifts and perform segmentation simultaneously. Second, we adopt Dynamic Instance Normalization to conduct precise and dynamic style transfer in a learnable manner, rather than previously fixed style normalization. Third, our solution is fast and lightweight for routine clinical adoption. Given 400x400 image input, our solution only needs an additional 0.2 ms and 1.92M FLOPs to handle appearance shifts compared to the baseline pipeline. Extensive experiments are conducted on a large dataset from three vendors demonstrate our proposed method enhances the robustness of deep segmentation models.
MoreTranslated text
Key words
Ultrasound,Style transfer,Segmentation
AI Read Science
Must-Reading Tree
Example
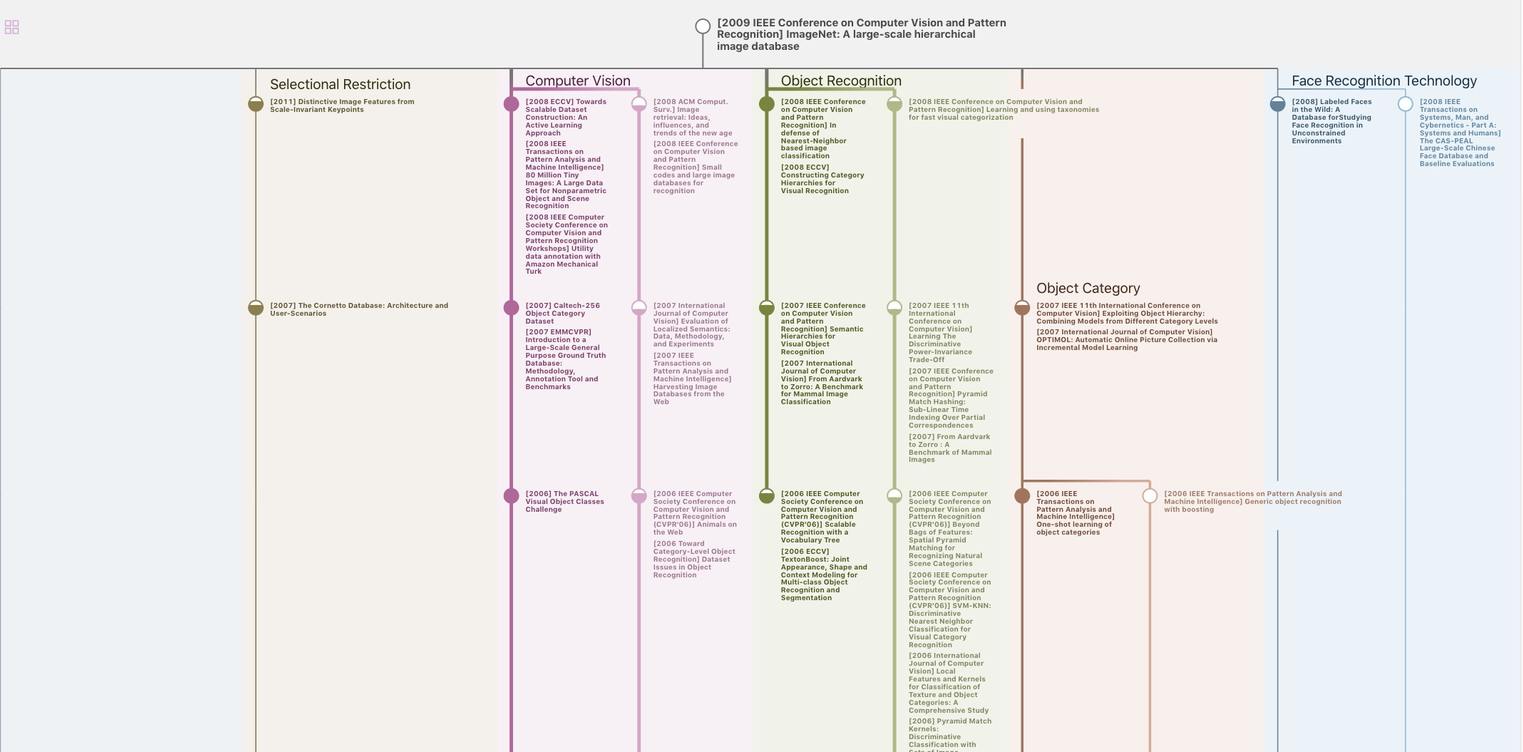
Generate MRT to find the research sequence of this paper
Chat Paper
Summary is being generated by the instructions you defined