Heteroscedasticity-aware residuals-based contextual stochastic optimization
arxiv(2021)
摘要
We explore generalizations of some integrated learning and optimization frameworks for data-driven contextual stochastic optimization that can adapt to heteroscedasticity. We identify conditions on the stochastic program, data generation process, and the prediction setup under which these generalizations possess asymptotic and finite sample guarantees for a class of stochastic programs, including two-stage stochastic mixed-integer programs with continuous recourse. We verify that our assumptions hold for popular parametric and nonparametric regression methods.
更多查看译文
关键词
contextual stochastic
AI 理解论文
溯源树
样例
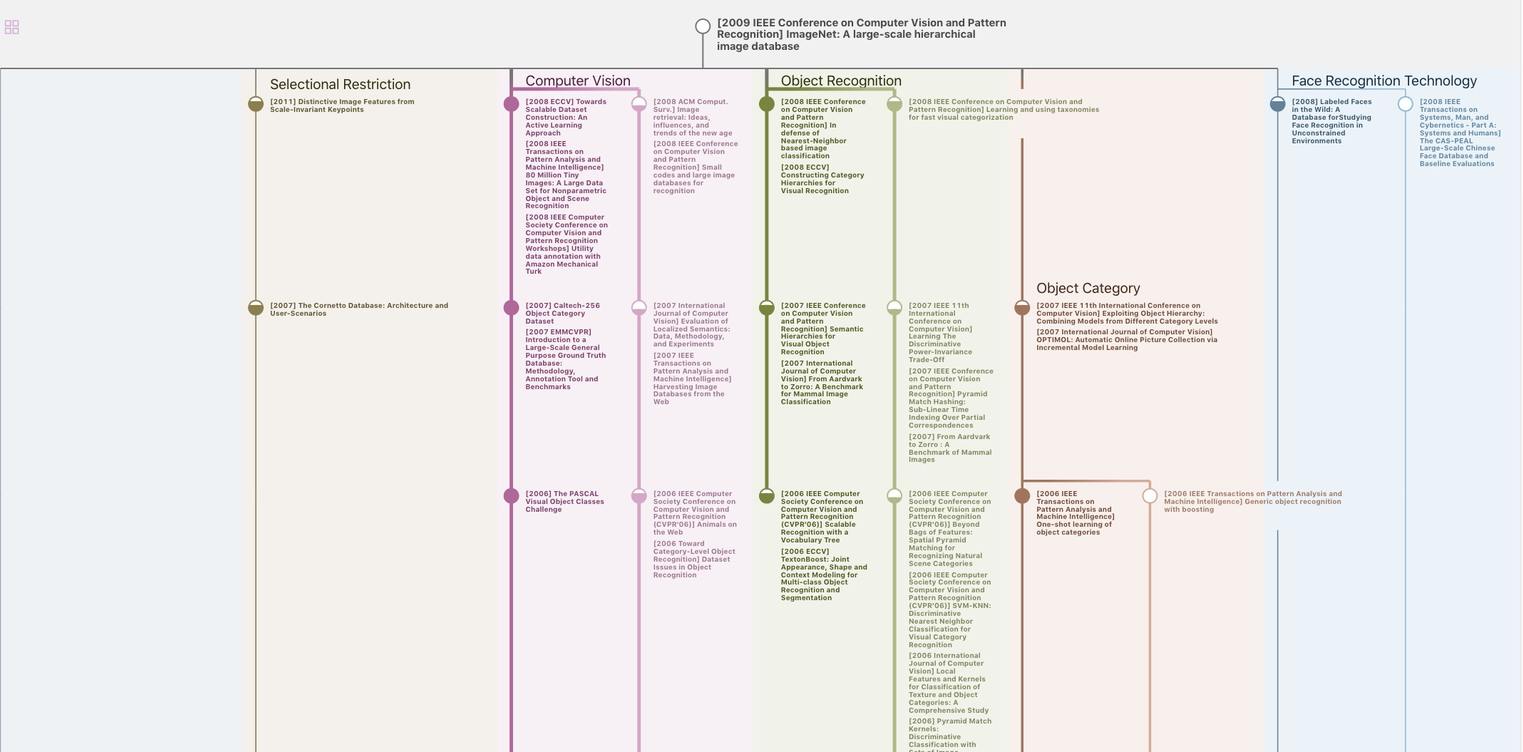
生成溯源树,研究论文发展脉络
Chat Paper
正在生成论文摘要