Review of different robust x-vector extractors for speaker verification
2020 28th European Signal Processing Conference (EUSIPCO)(2021)
摘要
Recently, the x-vector framework, extracted with deep neural network architectures, became the state-of-the-art method for speaker verification. Although another level of performance has been overcome with this approach, fine-tuning and optimizing the hyper-parameters of a deep neural network to obtain a robust x-vector extractor is cost- and time-consuming. Several approaches have been proposed to train robust x-vector extractors. In this paper, we propose to review and analyse the impact of the most significant x-vector related approaches, including variations in terms of data augmentation, number of epochs, size of mini-batch, acoustic features and frames per iteration. By applying these approaches to the default recipe provided in the Kaldi toolkit, we observed a significant relative gain of more than 50% in terms of EER on Speaker in the Wild and Voxceleb1-E datasets.
更多查看译文
关键词
x-vector,deep neural network,speaker verification
AI 理解论文
溯源树
样例
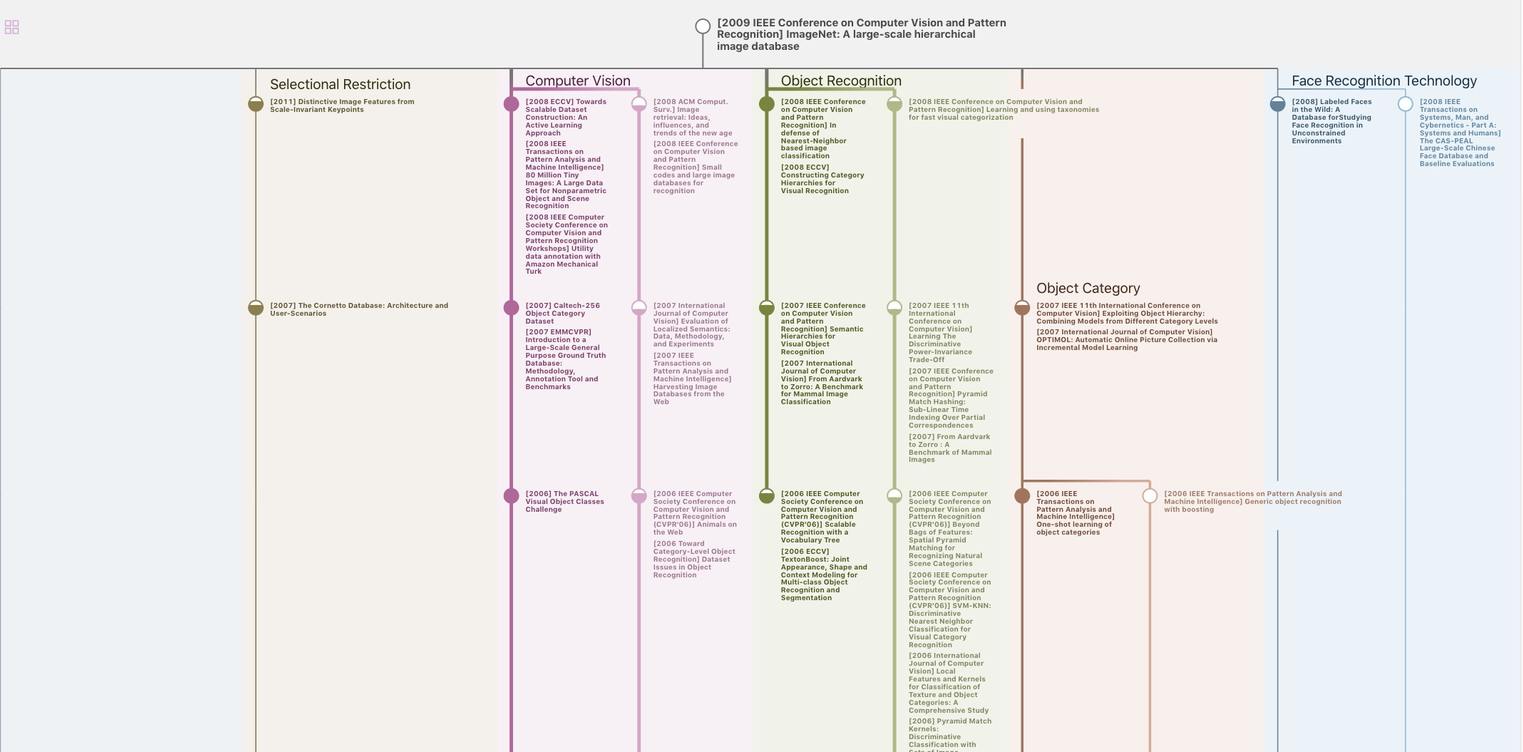
生成溯源树,研究论文发展脉络
Chat Paper
正在生成论文摘要