Distributed Semi-Private Image Classification Based On Information-Bottleneck Principle
28TH EUROPEAN SIGNAL PROCESSING CONFERENCE (EUSIPCO 2020)(2021)
摘要
In this paper, we propose a framework for semi-privacy-preserving image classification. It allows each user to train a model on her/his own particular data class, after which the output features are shared centrally. The model parameters are never shared. Individual users each use an auto-encoder to empirically ascertain their private data distribution. The resulting features are sufficiently discriminative between the private datasets. A central server aggregates all labeled output features together with a subset of the private data into a final classifier over all classes from all users. The latter forms a trade-off between privacy and classification performance. We demonstrate the viability of this scheme empirically and showcase the privacy performance compromise.
更多查看译文
关键词
privacy, information bottleneck, image classification, semi-private model
AI 理解论文
溯源树
样例
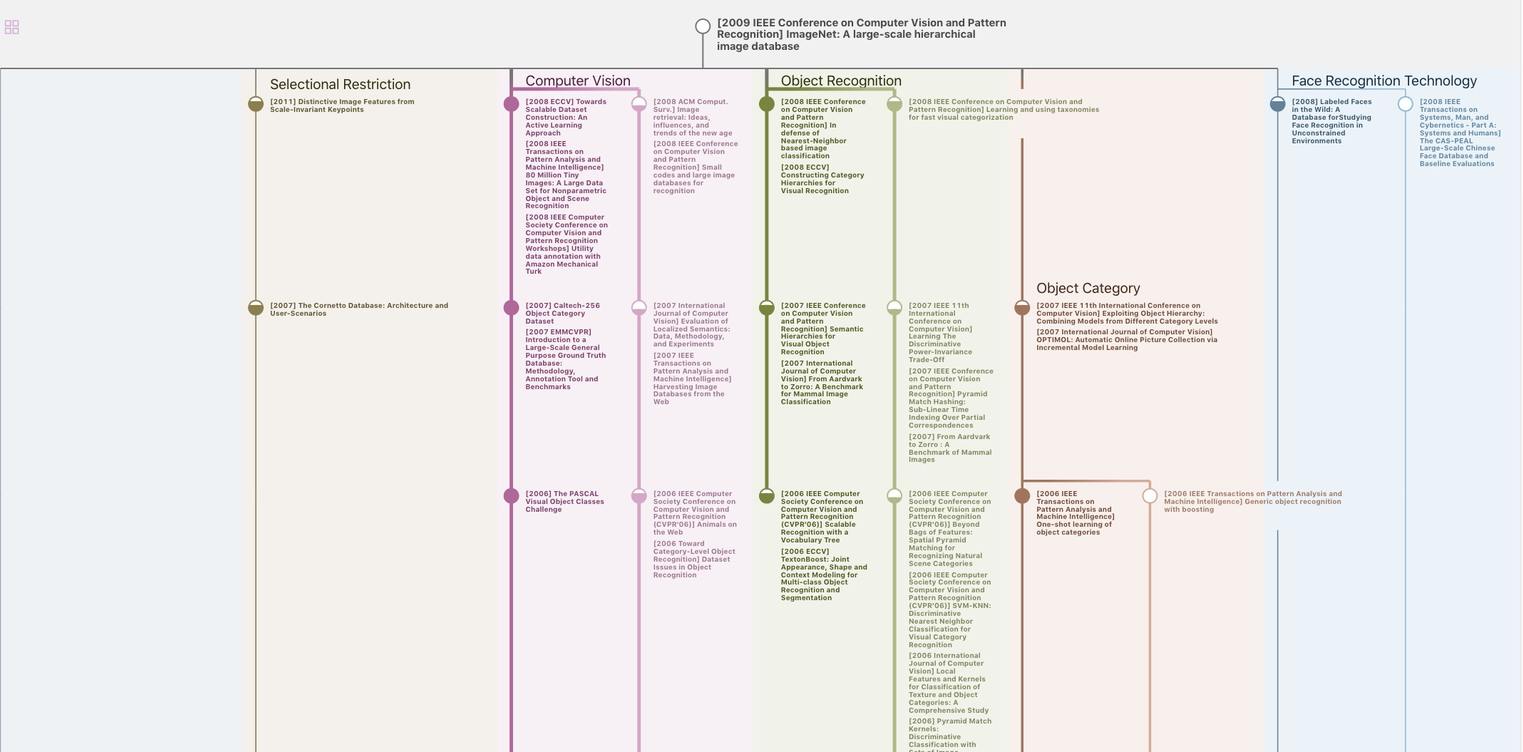
生成溯源树,研究论文发展脉络
Chat Paper
正在生成论文摘要