Deep Video Canonical Correlation Analysis For Steady State Motion Visual Evoked Potential Feature Extraction
28TH EUROPEAN SIGNAL PROCESSING CONFERENCE (EUSIPCO 2020)(2021)
Abstract
Recently, there has been a surge of interest in development of Brain Computer Interface (BCI) systems based on Steady-State motion-Visual Evoked Potentials (SSmVEP), where motion stimulation is utilized to address high brightness and uncomfortably issues associated with conventional light-flashing/flickering. In this paper, we propose a deep learning-based classification model that extracts features of the SSmVEPs directly from the videos of stimuli. More specifically, the proposed deep architecture, referred to as the Deep Video Canonical Correlation Analysis (DvCCA), consists of a Video Feature Extractor (VFE) layer that uses characteristics of videos utilized for SSmVEP stimulation to fit the template EEG signals of each individual, independently. The proposed VFE layer extracts features that are more correlated with the stimulation video signal as such eliminates problems, typically, associated with deep networks such as overfitting and lack of availability of sufficient training data. The proposed DvCCA is evaluated based on a real EEG dataset and the results corroborate its superiority against recently proposed state-of-the-art deep models.
MoreTranslated text
Key words
Steady State Motion Evoked Potentials, EEG Signals, Brain Computer Interfaces, Deep CCA
AI Read Science
Must-Reading Tree
Example
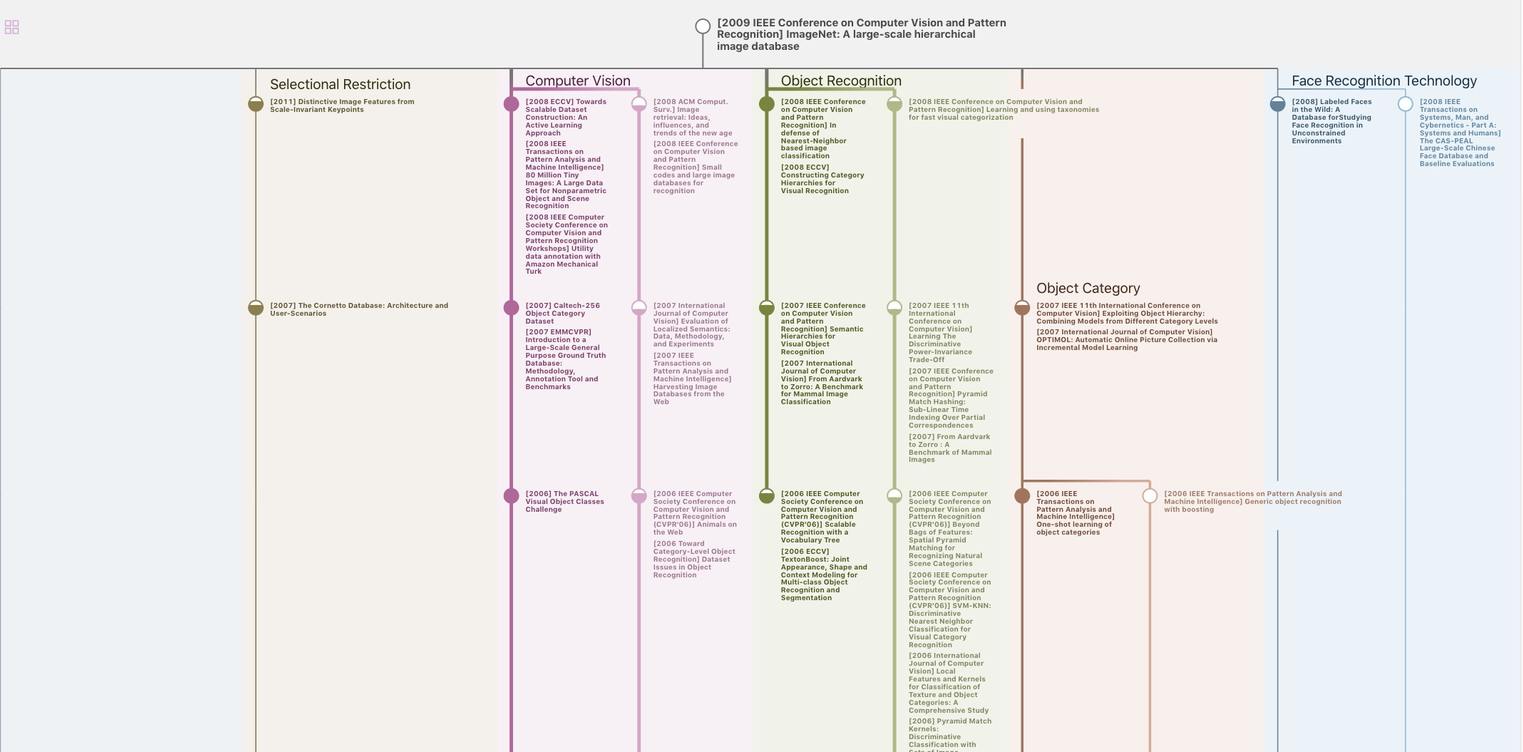
Generate MRT to find the research sequence of this paper
Chat Paper
Summary is being generated by the instructions you defined