Online Dominant Generalized Eigenvectors Extraction Via A Randomized Method
28TH EUROPEAN SIGNAL PROCESSING CONFERENCE (EUSIPCO 2020)(2021)
Abstract
The generalized Hermitian eigendecomposition problem is ubiquitous in signal and machine learning applications. Considering the need of processing streaming data in practice and restrictions of existing methods, this paper is concerned with fast and efficient generalized eigenvectors tracking. We first present a computationally efficient algorithm based on randomization termed alternate-projections randomized eigenvalue decomposition (APR-EVD) to solve a standard eigenvalue problem. By exploiting rank-1 strategy, two online algorithms based on APR-EVD are developed for the dominant generalized eigenvectors extraction. Numerical examples show the practical applicability and efficacy of the proposed online algorithms.
MoreTranslated text
Key words
Randomized algorithms, dominant generalized eigenvectors, online algorithms, fast subspace tracking
AI Read Science
Must-Reading Tree
Example
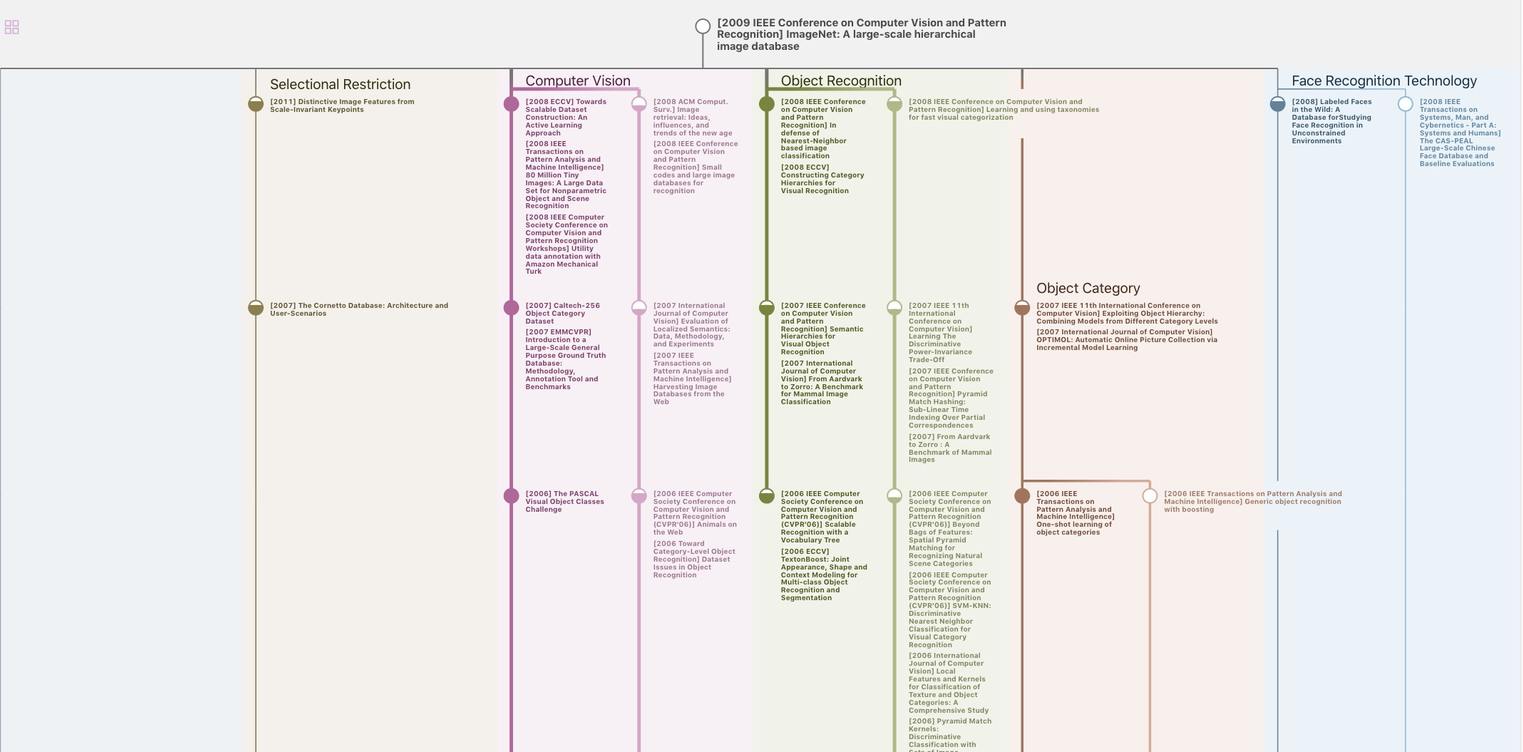
Generate MRT to find the research sequence of this paper
Chat Paper
Summary is being generated by the instructions you defined