Multiple Speaker Localization Using Mixture Of Gaussian Model With Manifold-Based Centroids
28TH EUROPEAN SIGNAL PROCESSING CONFERENCE (EUSIPCO 2020)(2021)
摘要
A data-driven approach for multiple speakers localization in reverberant enclosures is presented. The approach combines semi-supervised learning on multiple manifolds with unsupervised maximum likelihood estimation. The relative transfer functions (RTFs) are used in both stages of the proposed algorithm as feature vectors, which are known to be related to source positions. The microphone positions are not known. In the training stage, a nonlinear, manifold-based, mapping between RTFs and source locations is inferred using single-speaker utterances. The inference procedure utilizes two RTF datasets: A small set of RTFs with their associated position labels; and a large set of unlabelled RTFs. This mapping is used to generate a dense grid of localized sources that serve as the centroids of a Mixture of Gaussians (MoG) model, used in the test stage of the algorithm to cluster RTFs extracted from multiple-speakers utterances. Clustering is applied by applying the expectation-maximization (EM) procedure that relies on the sparsity and intermittency of the speech signals. A preliminary experimental study, with either two or three overlapping speakers in various reverberation levels, demonstrates that the proposed scheme achieves high localization accuracy compared to a baseline method using a simpler propagation model.
更多查看译文
关键词
Manifold-learning, semi-supervised inference, mixture of Gaussians
AI 理解论文
溯源树
样例
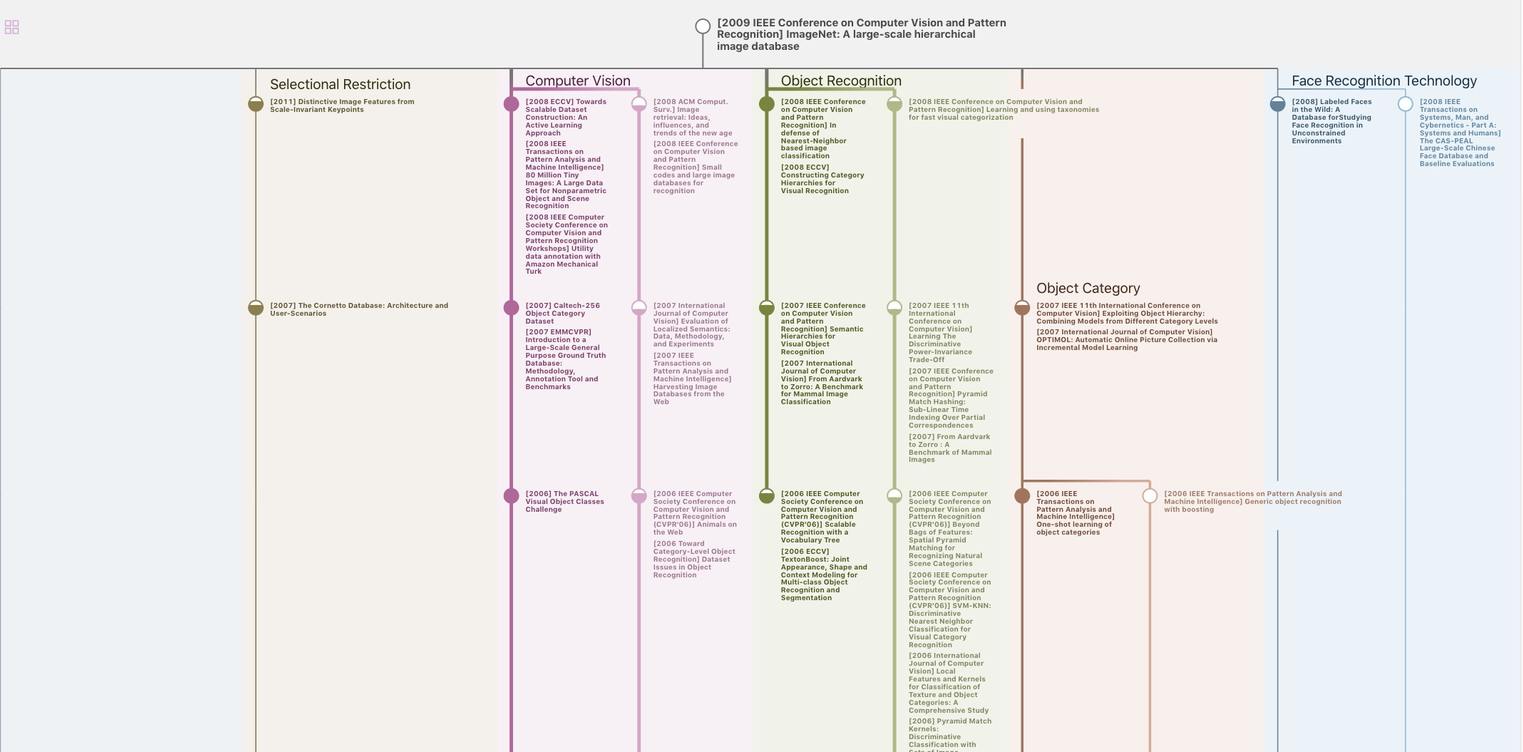
生成溯源树,研究论文发展脉络
Chat Paper
正在生成论文摘要