Learning Effective Value Function Factorization via Attentional Communication
2020 IEEE International Conference on Systems, Man, and Cybernetics (SMC)(2020)
摘要
How to achieve efficient cooperation among agents in partially observed environments remains an overarching problem in multi-agent reinforcement learning (MARL). Value function factorization learning is a promising way as it can efficiently address multi-agent credit assignment problem. However, existing value function factorization methods have been focusing on learning fully decentralized value functions, which are not effective for some complex tasks. To address this limitation, we propose a framework which enhances value function factorization by allowing communication during execution. Communication introduces extra information to help agents understand the complex environment and learn sophisticated factorization. Furthermore, the proposed mechanism of communication differs from existing methods since we additionally design a descriptive key along with the message. By the descriptive key, agents can dynamically measure the importance of different messages and achieve attentional communication. We evaluate our framework on a challenging set of StarCraft II micromanagement tasks, and show that it significantly outperforms existing value function factorization methods.
更多查看译文
关键词
multi-agent reinforcement learning,value function factorization,attention,communication
AI 理解论文
溯源树
样例
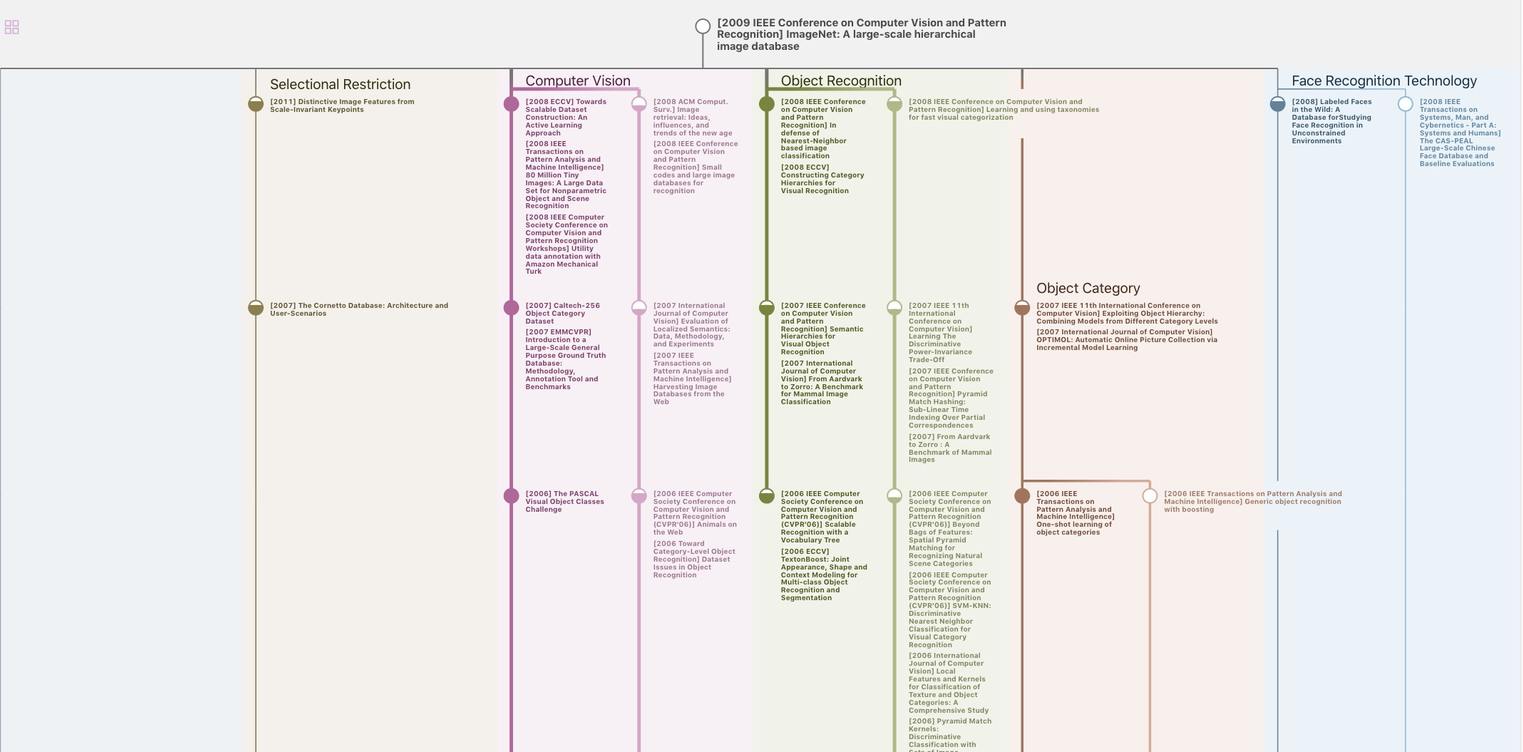
生成溯源树,研究论文发展脉络
Chat Paper
正在生成论文摘要