Free-Head Pose Estimation under Low-Resolution Scenarios.
SMC(2020)
摘要
Head pose offers vital cues to infer one's social attention in wide applications. Most existing head pose estimation algorithms have demonstrated competitive results taking high resolution images of frontal view as input. However, these approaches still work poorly if they are fed with low-resolution images. In a more common realistic scene such as computer vision assisted autism screening, images of unconstrained patients that are taken from distant cameras often have low-resolution and non-frontal faces. To this end, we present a multi-view scheme based CNN for free-head pose classification. Residual Networks are taken as the backbone model to generate effective feature representations of the low-resolution images. A novel multi-view feature fusion layer is proposed to address facial appearance variation over multi perspectives owing to free movement. Also, a multi loss function by combining binned classification and regression losses of different pose angles is employed to obtain a more precise pose estimation. The proposed method is evaluated on two scenarios: (1) a publicly available dataset and (2) a practical application to explore the social attention of a group with social deficits: autistic children. Experimental results suggest that our method significantly outperforms state-of-the-art multi-view pose classification methods and achieves comparable pose estimation results. Moreover, the proposed method can be extended to applications of quantitative analysis of social deficits.
更多查看译文
关键词
head pose estimation, multi view, low resolution, social attention
AI 理解论文
溯源树
样例
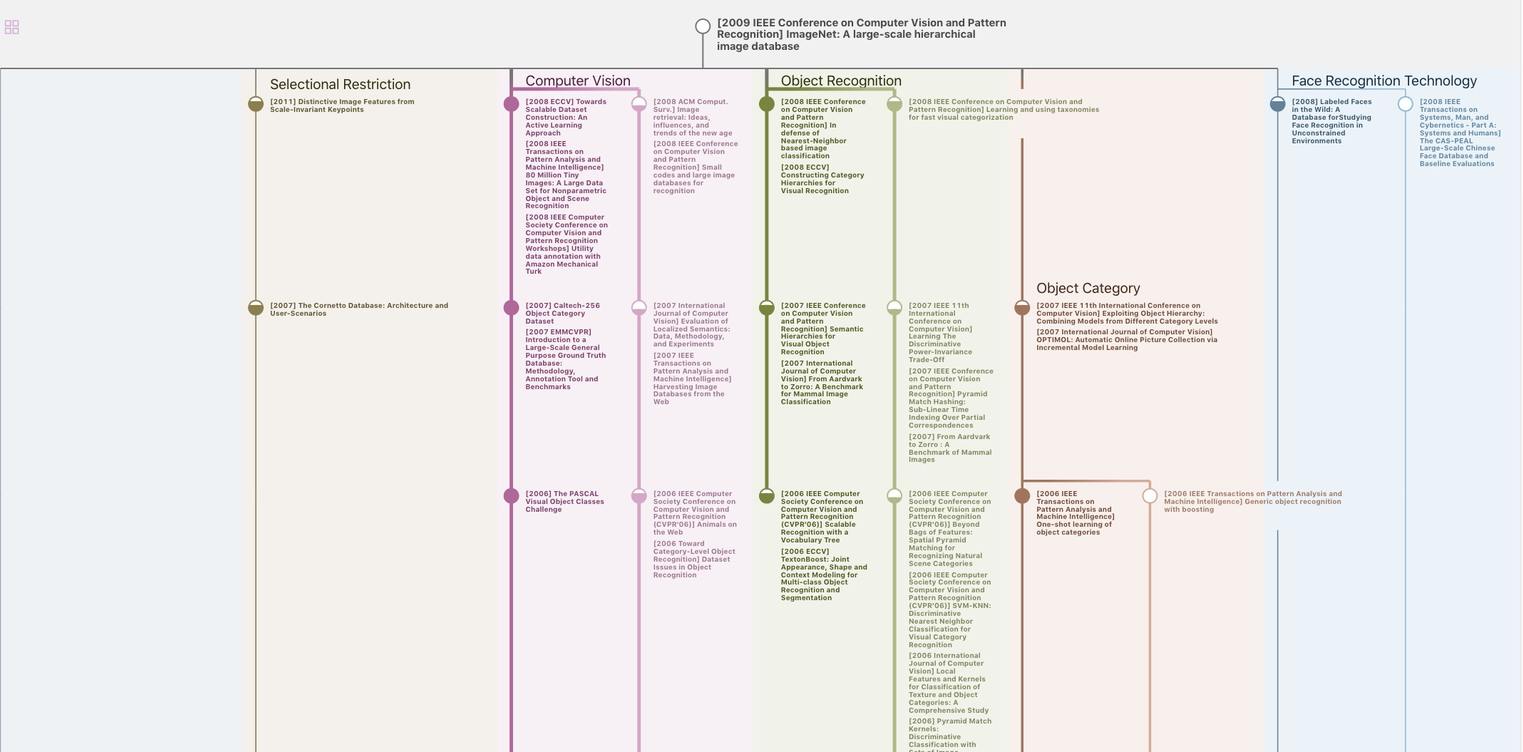
生成溯源树,研究论文发展脉络
Chat Paper
正在生成论文摘要