Research on Plant Disease Recognition Based on Deep Complementary Feature Classification Network.
SMC(2020)
摘要
Traditional convolutional neural network classification models often only focus on the most distinguishing feature regions of the image and ignore the weaker feature regions. However, the image position distribution of plant diseases is very uneven. If we use convolutional neural network for plant disease recognition, there will be insufficient feature response, which will cause recognition errors. Aiming at such problems, we have designed a deep complementary feature classification network. First, the network uses DeepLabv3+ and Conditional Random Field (CRF) to generate disease part detection frames in a weakly supervised manner and combines semantic segmentation to extract disease object instances. Then we designed Complementary Feature Part Generation Models. Finally, it uses a bidirectional Gated Recurrent Unit (Bi-GRU) to perform the classification and recognition of the complementary features described above. We performed experiments on the PlantVillage dataset. The experimental results show that the proposed network recognition accuracy is 99.21%, which is 4.2% higher than the baseline model xception-65 used. We also performed experiments on the grape disease data set that we created. The accuracy of the proposed network recognition is 93.46%, which is 7.2% higher than the baseline model xception-65. In addition, compared with the better algorithms for plant disease identification in recent years, the accuracy performance has also been improved.
更多查看译文
关键词
Plant disease identification, Deep complementary features, DeepLabv3+, CRF, Bi-GRU
AI 理解论文
溯源树
样例
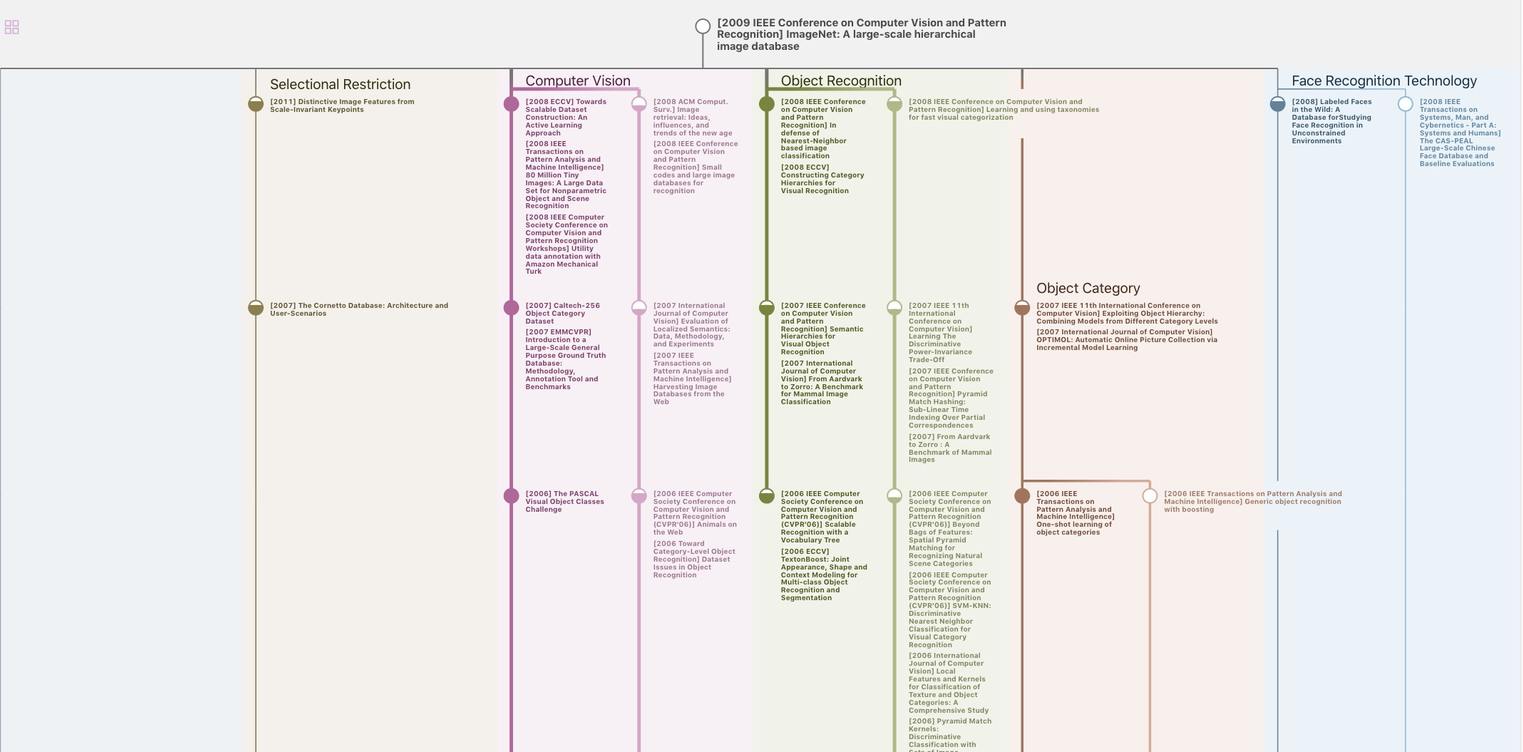
生成溯源树,研究论文发展脉络
Chat Paper
正在生成论文摘要