A Novel Multi-Knowledge Distillation Approach
IEICE TRANSACTIONS ON INFORMATION AND SYSTEMS(2021)
摘要
Knowledge distillation approaches can transfer information from a large network (teacher network) to a small network (student network) to compress and accelerate deep neural networks. This paper proposes a novel knowledge distillation approach called multi-knowledge distillation (MKD). MKD consists of two stages. In the first stage, it employs autoencoders to learn compact and precise representations of the feature maps (FM) from the teacher network and the student network, these representations can be treated as the essential of the FM, i.e., EFM. In the second stage, MKD utilizes multiple kinds of knowledge, i.e., the magnitude of individual sample's EFM and the similarity relationships among several samples' EFM to enhance the generalization ability of the student network. Compared with previous approaches that employ FM or the handcrafted features from FM, the EFM learned from autoencoders can be transferred more efficiently and reliably. Furthermore, the rich information provided by the multiple kinds of knowledge guarantees the student network to mimic the teacher network as closely as possible. Experimental results also show that MKD is superior to the-state-of-arts.
更多查看译文
关键词
knowledge distillation, deep neural networks, compress, accelerate, autoencoders
AI 理解论文
溯源树
样例
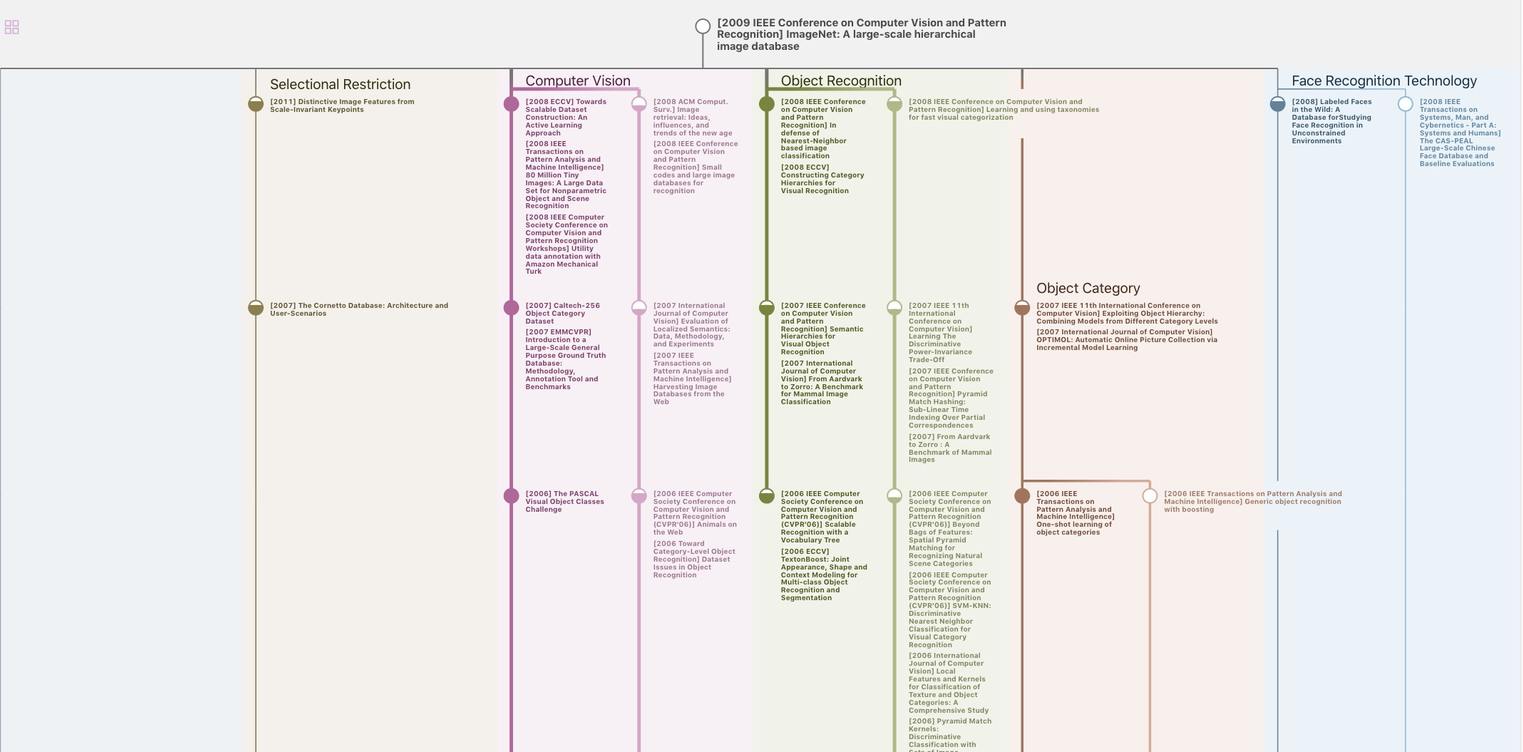
生成溯源树,研究论文发展脉络
Chat Paper
正在生成论文摘要