Nano-Crossbar Weighted Memristor-Based Convolution Neural Network Architecture for High-Performance Artificial Intelligence Applications.
Journal of nanoscience and nanotechnology(2021)
摘要
Nano memristor crossbar arrays, which can represent analog signals with smaller silicon areas, are popularly used to describe the node weights of the neural networks. The crossbar arrays provide high computational efficiency, as they can perform additions and multiplications at the same time at a cross-point. In this study, we propose a new approach for the memristor crossbar array architecture consisting of multi-weight nano memristors on each cross-point. As the proposed architecture can represent multiple integer-valued weights, it can enhance the precision of the weight coefficients in comparison with the existing memristor-based neural networks. This study presents a Radix-11 nano memristor crossbar array with weighted memristors; it validates the operations of the circuits, which use the arrays through circuit-level simulation. With the proposed Radix-11 approach, it is possible to represent eleven integer-valued weights. In addition, this study presents a neural network designed using the proposed Radix-11 weights, as an example of high-performance AI applications. The neural network implements a speech-keyword detection algorithm, and it was designed on a TensorFlow platform. The implemented keyword detection algorithm can recognize 35 Korean words with an inferencing accuracy of 95.45%, reducing the inferencing accuracy only by 2% when compared to the 97.53% accuracy of the real-valued weight case.
更多查看译文
关键词
Memristor
AI 理解论文
溯源树
样例
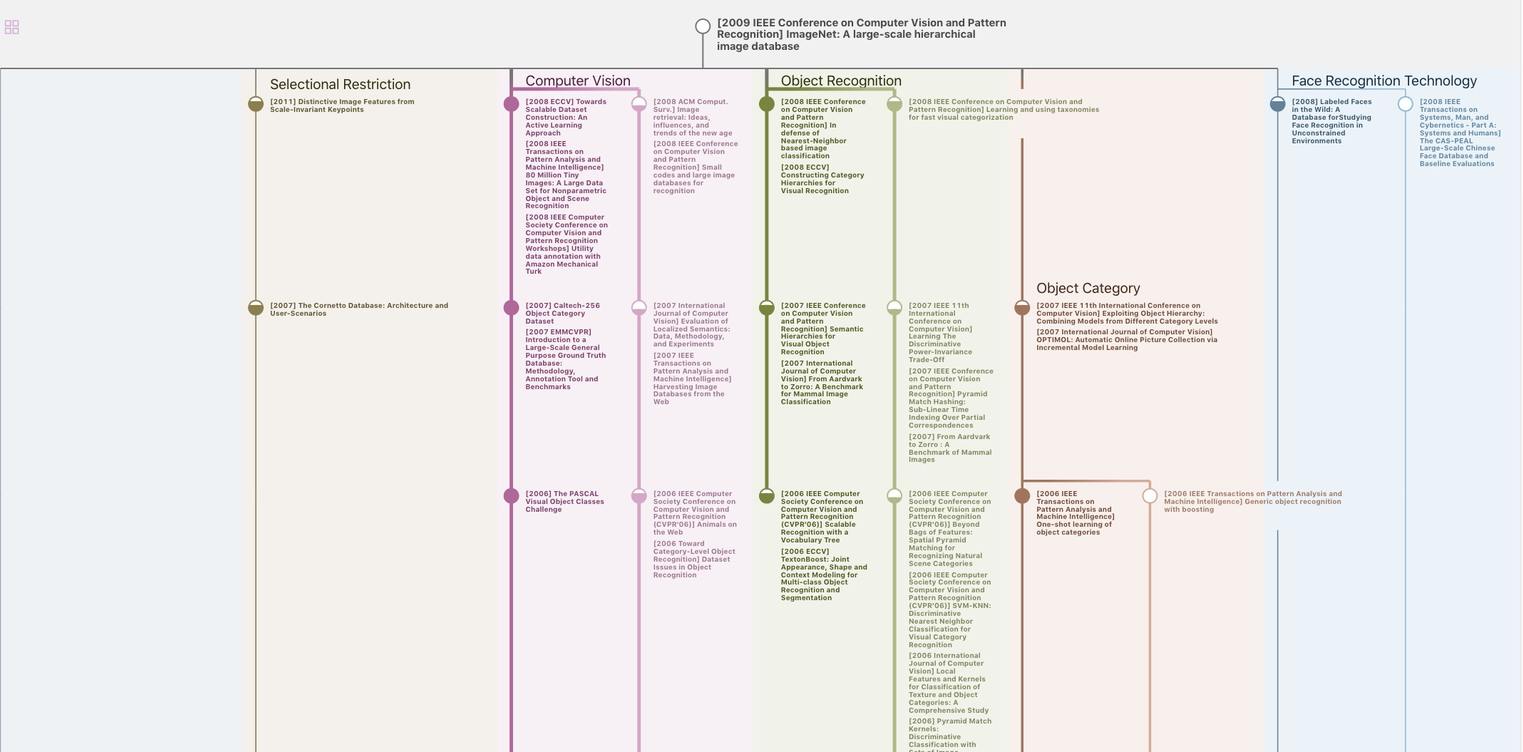
生成溯源树,研究论文发展脉络
Chat Paper
正在生成论文摘要