Optimization of Learning Strategies for ARTM-Based Topic Models.
HAIS(2020)
摘要
Topic modelling is a popular unsupervised method for text processing which provides interpretable document representation. One of the most high-level approaches, considering its capability of imitating the behaviour of various methods such as LDA or PLSA, is based on additive regularization technique. However, due to its flexibility and advanced regularization abilities, it is challenging to find optimal learning strategy to create high-quality topics, because a user needs to select the regularizers with their values and determine the order of application. At the same time, there is a lack of research on parameters optimization of topic models, especially for ARTM-based models. Our work proposes an approach that formalizes the learning strategy into a vector of parameters which can be solved with an evolutionary or Bayesian approach. An experimental study conducted on English and Russian datasets indicates that the proposed learning strategy can be successfully optimized even in the presence of strong constrains.
更多查看译文
关键词
topic,models,learning strategies,artm-based
AI 理解论文
溯源树
样例
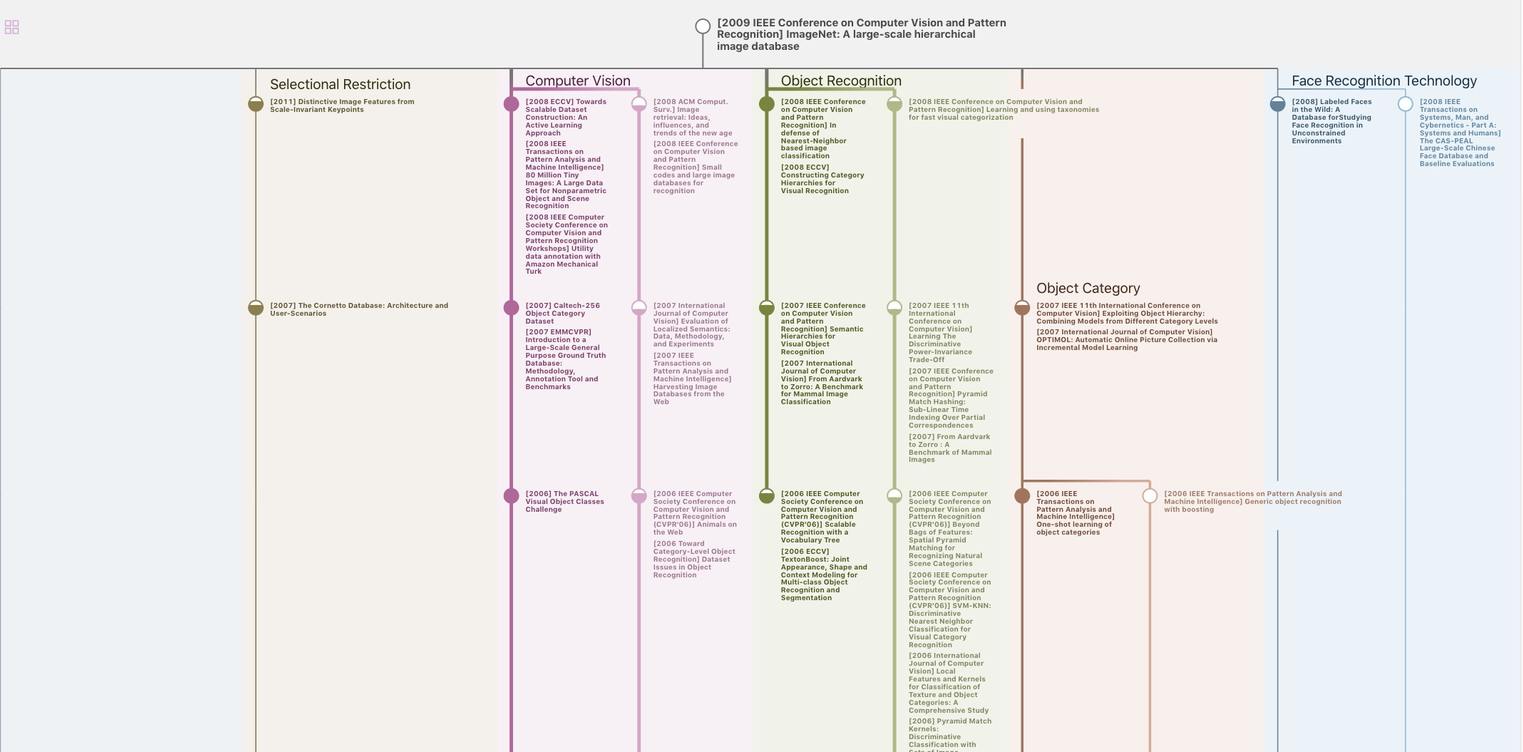
生成溯源树,研究论文发展脉络
Chat Paper
正在生成论文摘要