CSTA-2P1D UNet: Consecutive Spatio-Temporal Attention for Multi-Scale 3D Pancreas Segmentation
ICCCNT(2020)
摘要
Accurate pancreas segmentation is a crucial task for the diagnosis of diseases as well as for developing modern surgical tools. In this paper, a novel architecture, named Consecutive Spatio-Temporal Attention (CSTA) 2 Plus 1 Dimensional (2P1D) UNet, is proposed for the volumetric segmentation of the pancreas in CT images. CSTA mechanism is inspired by recent advancements in Squeeze & Excitation (SE) module. It recalibrates feature maps, by multiplying learnable spatial and channel-wise attention maps, sequentially. Further, incorporation of Multi-Sale Output (MSO) and Group Normalization (GN) improves the localization performance and training stability of the model, respectively. Moreover, 2P1D convolution is introduced to enhance the segmentation results of our proposed architecture. Smoothed Dice Loss (SDL) is used as a loss function for training of the proposed model. Collectively, a state-of-the-art performance of 83.70%, 87.70%, and 85.60% on dice score, sensitivity, and precision is achieved, verifying the model's effectiveness for the pancreas segmentation.
更多查看译文
关键词
Pancreas Segmentation,Consecutive Spatio-Temporal Attention,2 Plus 1 D-UNet,Multi-Scale Output,Group Normalization,Smoothed Dice Loss
AI 理解论文
溯源树
样例
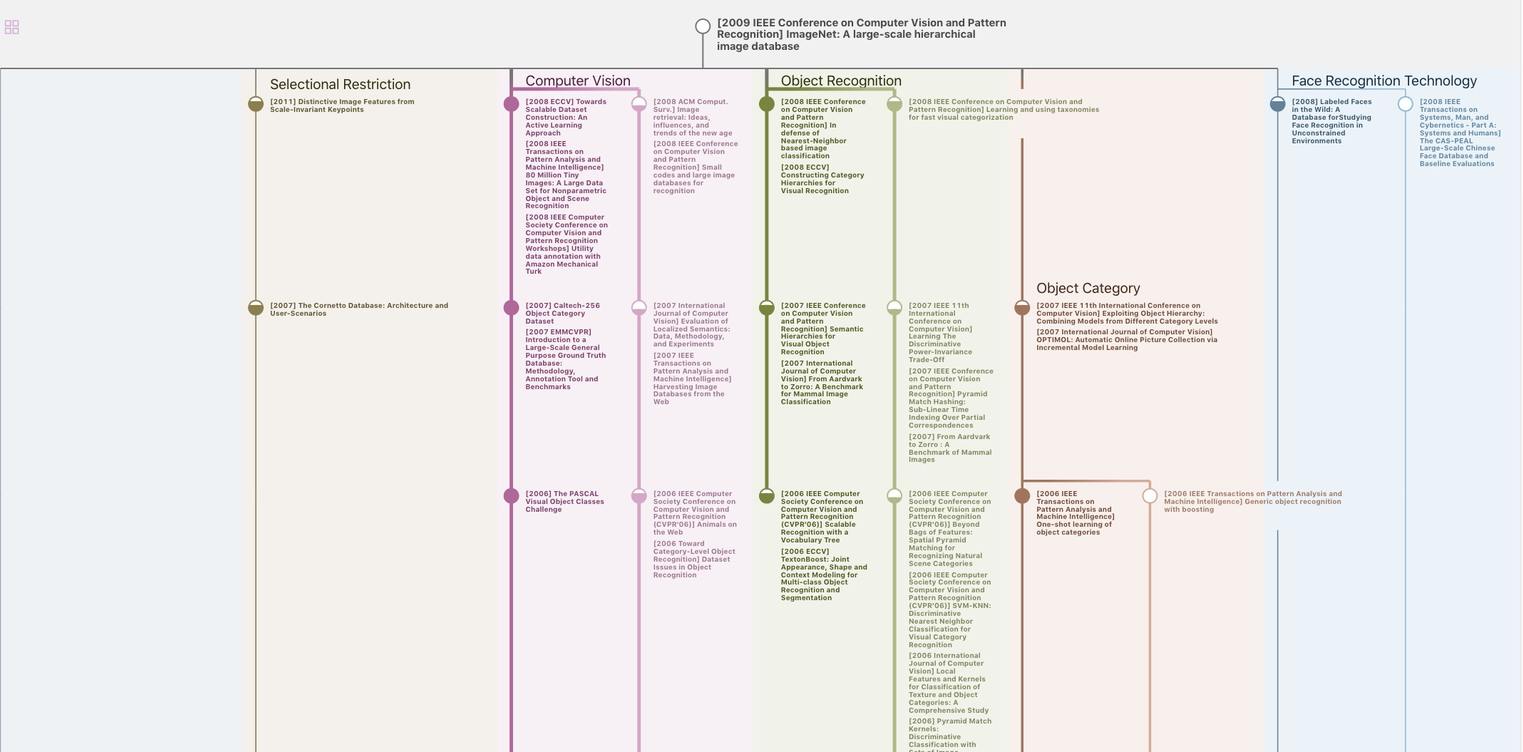
生成溯源树,研究论文发展脉络
Chat Paper
正在生成论文摘要