M3la: A Novel Approach Based On Encoder-Decoder With Attention Framework For Multi-Modal Multi-Label Learning
2020 INTERNATIONAL JOINT CONFERENCE ON NEURAL NETWORKS (IJCNN)(2020)
摘要
With the exponential growth of digital multimedia resources, in the real-world, most of the data are represented as a multi-modal form and usually with multiple semantic labels. Nowadays, Multi-modal Multi-label learning has become a very hot topic. However, previous methods either have not considered the relation between modalities and labels or the correlation among labels. In this paper, we considered the following three questions: (1) How to model the correlation among labels? (2) Is there a correlation between modality and label? (3) Whether the modal input order affects the prediction of individual instance, and how to find the most appropriate modal input sequence for each instance? To solve above problems, we proposed a novel method for Multi-modal Multi-label learning(MMML), which based on Encoder-Decoder with attention framwork named MMML-Attention(M3LA). The M3LA takes into account all of these issues. Specifically, benefit from the Encoder-Decoder with attention structure, on the one hand, M3LA can model the relation between modalities and labels. On the other hand, we introduce a correlation matrix to learn the correlation among labels, which can be obtained as parameter through the training process. It should be mentioned that label prediction occurs at every step of the decoder, and the prediction of the label is constantly corrected and then the most accurate prediction is obtained. To validate the effectiveness of the proposed method, we expermiented on widely used several benchmark datasets and compared with state-of-art approaches.
更多查看译文
关键词
multi-label, multi-modal, classfication, machine learning, deep learning
AI 理解论文
溯源树
样例
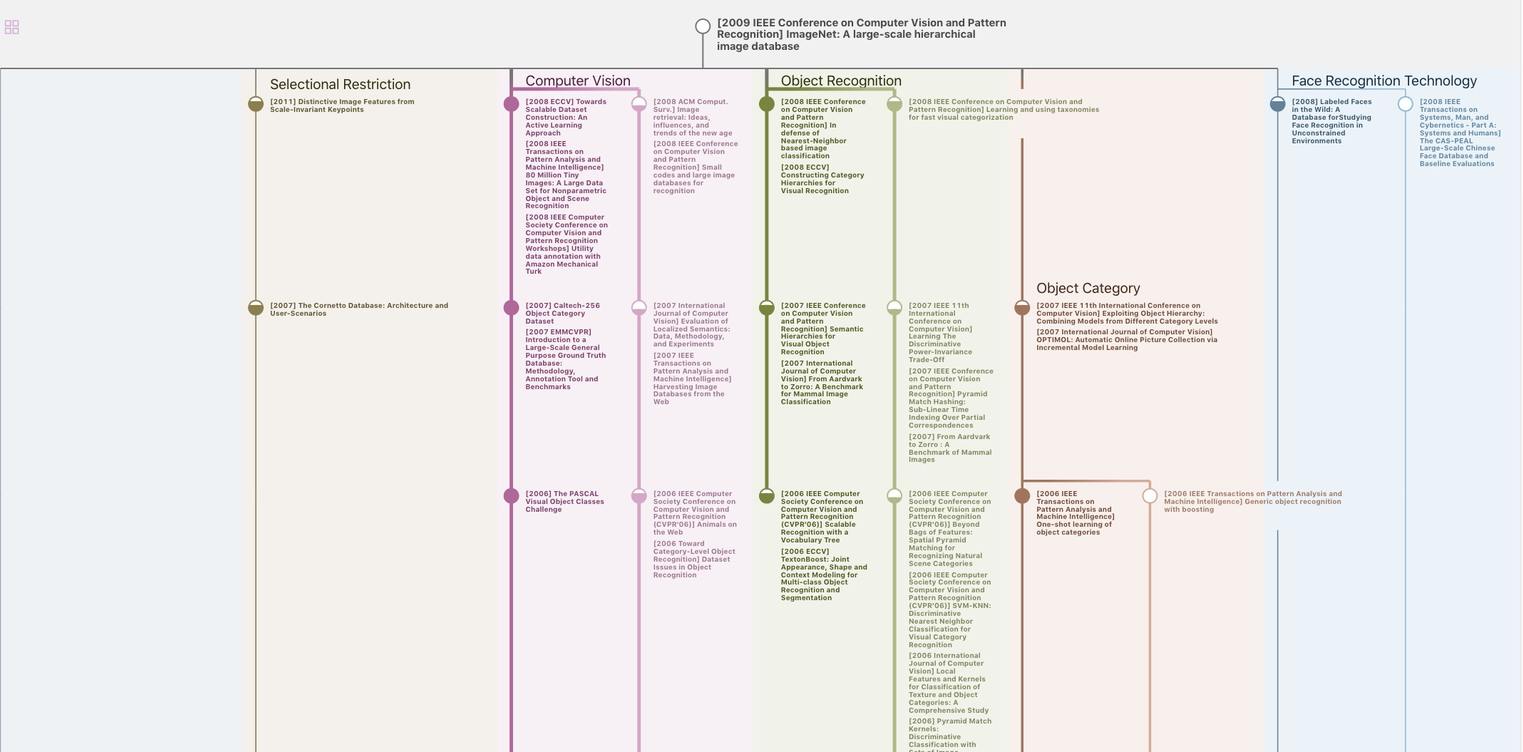
生成溯源树,研究论文发展脉络
Chat Paper
正在生成论文摘要