A Computational Model For Latent Learning Based On Hippocampal Replay
2020 INTERNATIONAL JOINT CONFERENCE ON NEURAL NETWORKS (IJCNN)(2020)
摘要
We show how hippocampal replay could explain latent learning, a phenomenon observed in animals where unrewarded pre-exposure to an environment, i.e. habituation, improves task learning rates once rewarded trials begin. We first describe a computational model for spatial navigation inspired by rat studies. The model exploits offline replay of trajectories previously learned by applying reinforcement learning. Then, to assess our hypothesis, the model is evaluated in a "multiple Tmaze" environment where rats need to learn a path from the start of the maze to the goal. Simulation results support our hypothesis that pre-exposed or habituated rats learn the task significantly faster than non-pre-exposed rats. Results also show that this effect increases with the number of pre-exposed trials.
更多查看译文
关键词
computational model,latent learning,hippocampal replay,unrewarded pre-exposure,task learning rates,spatial navigation,rat studies,reinforcement learning,multiple T-maze environment,nonpre-exposed rats,pre-exposed trials,habituated rat learning
AI 理解论文
溯源树
样例
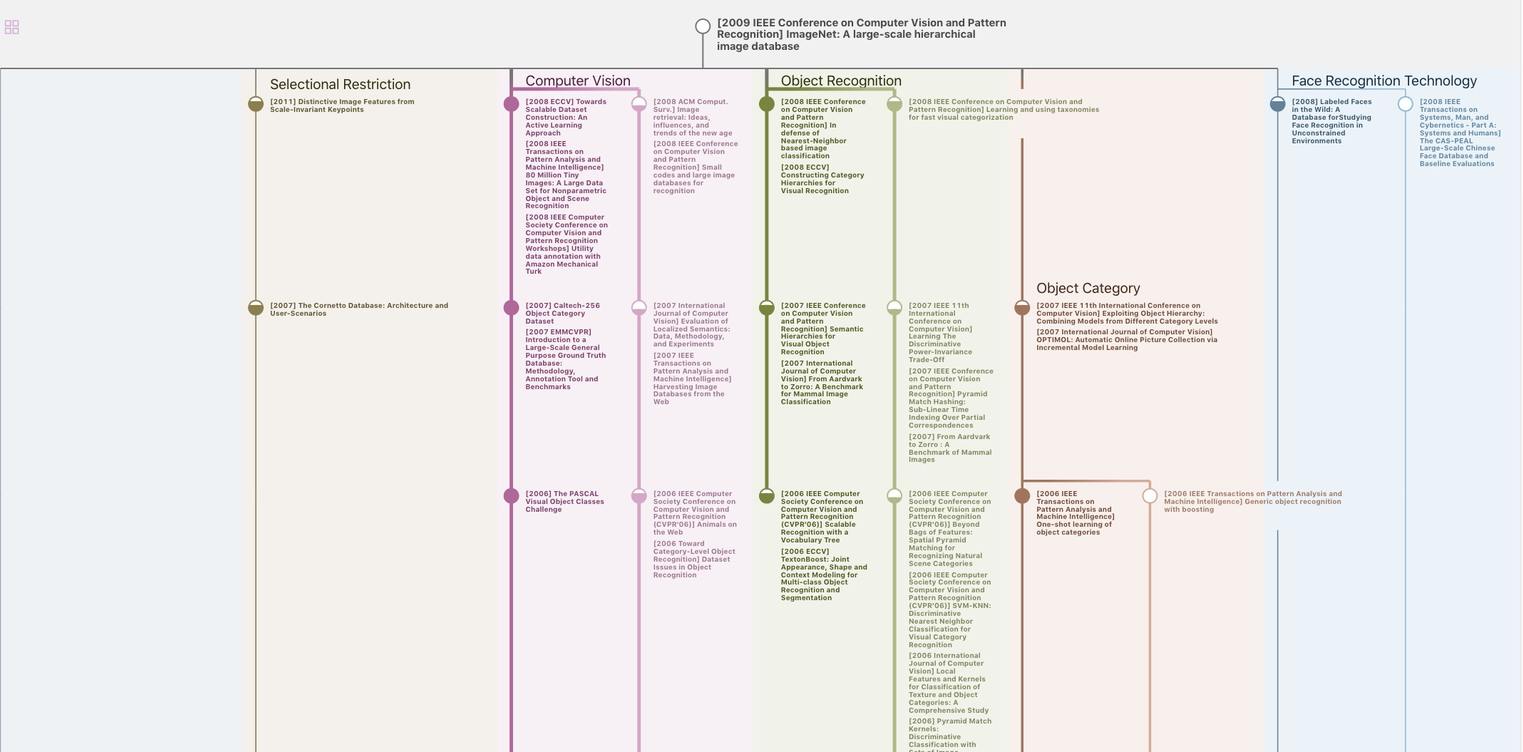
生成溯源树,研究论文发展脉络
Chat Paper
正在生成论文摘要